Building a Landsat-8 Cloud Detection Sample Database Using a Semi-Supervised Learning Method.
IEEE International Geoscience and Remote Sensing Symposium (IGARSS)(2022)
摘要
Cloud detection of satellite images is an important preprocessing step for remote sensing applications due to the adverse effect of clouds on data analysis. With the development of machine learning methods, an increasing number of studies have applied the state-of-art technology to detect clouds in satellite imagery. However, machine learning-based cloud detection methods are often limited by a small number of training samples with insufficient representativeness. To improve the robustness of cloud detection, developing a method to automatically construct a reliable training sample database is a meaningful issue. This paper explores a hybrid Erosion morphology processing and Disagreement-based Semi-supervised Learning model (EDSL) to refine cloud samples generated by the widely-used Fmask algorithm. Experimental results show that the sample accuracy after refinement could be improved from the original Fmask cloud masks. Further, the random forest model trained by the sample database after EDSL refinement achieves comparable accuracy but higher efficiency with Fmask algorithm, thus proving the effectiveness of the above method.
更多查看译文
关键词
Cloud Detection, Sample Database, Semi-supervised learning, EDSL, Random Forest
AI 理解论文
溯源树
样例
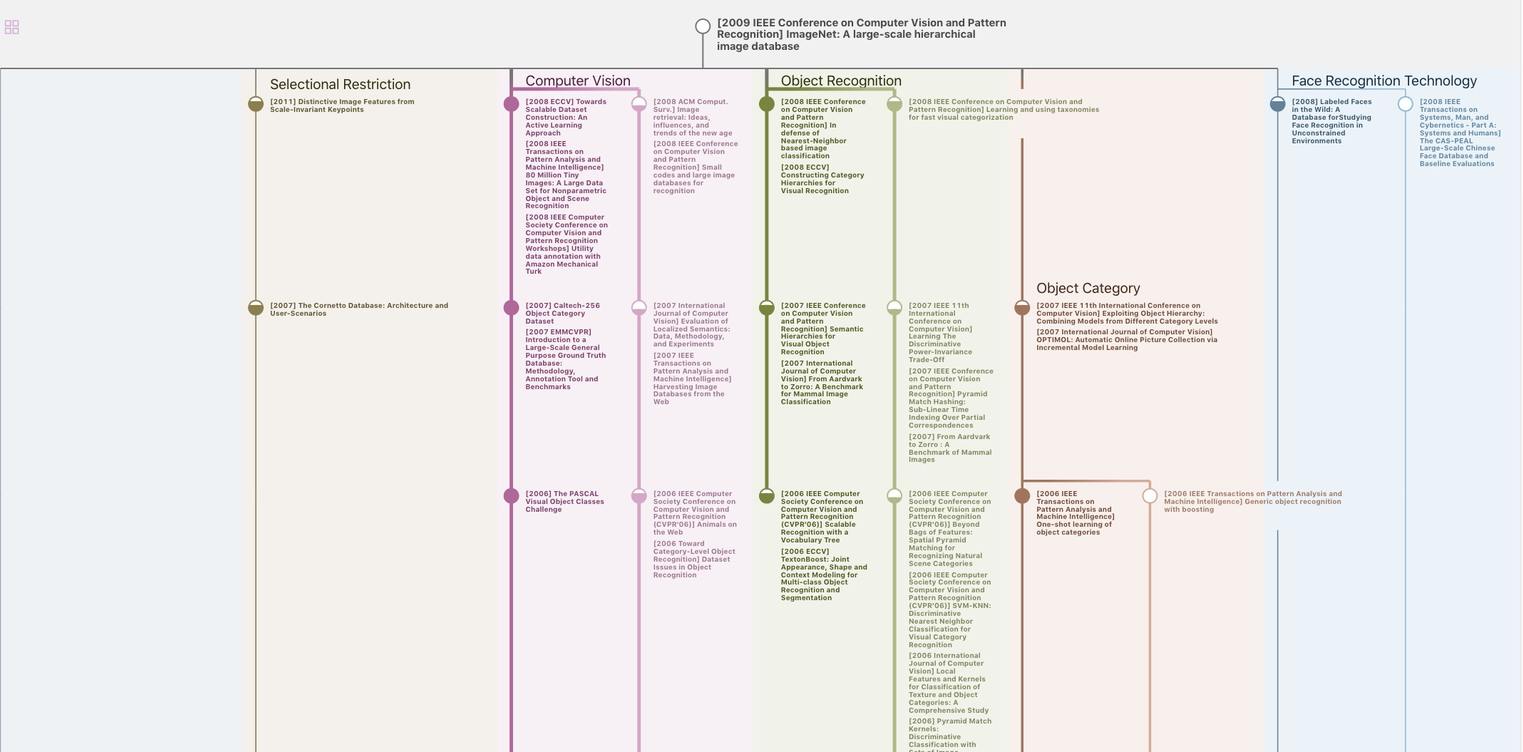
生成溯源树,研究论文发展脉络
Chat Paper
正在生成论文摘要