Autoencoder in Autoencoder Network Based on Low-Rank Embedding for Anomaly Detection in Hyperspectral Images.
IEEE International Geoscience and Remote Sensing Symposium (IGARSS)(2022)
摘要
The main purpose of anomaly detection in hyperspectral images is to detect targets that are different from their surroundings. With the development of deep learning technology, anomaly detection in hyperspectral images using deep neural networks has drawn great attention in recent years. However, most of the existing deep learning-based anomaly detection algorithms fail to consider the low-rank properties of the background and underutilize the rich spectral information of the image. In this paper, we propose a novel autoencoder in autoencoder network based on low-rank module embedding for anomaly detection in hyperspectral images. Firstly, the background is purified by using the low-rank module (LRM), and then the image background is reconstructed by using autoencoder in autoencoder network (AiANet), which is a spatial-spectral dual encoding-decoding network. AiANet fully considers the differences between anomalies and backgrounds in spatial and spectral dimensions to better reconstruct the background. Finally, the anomaly appears in images as reconstruction errors. Our proposed method effectively exploits the low-rank property of the backgrounds and makes full use of the spectral information to extract pure backgrounds to separate anomalies. Experiments on two real hyperspectral images demonstrate that the proposed method outperforms the other competitors.
更多查看译文
关键词
Anomaly detection,convolutional neural network,low rank
AI 理解论文
溯源树
样例
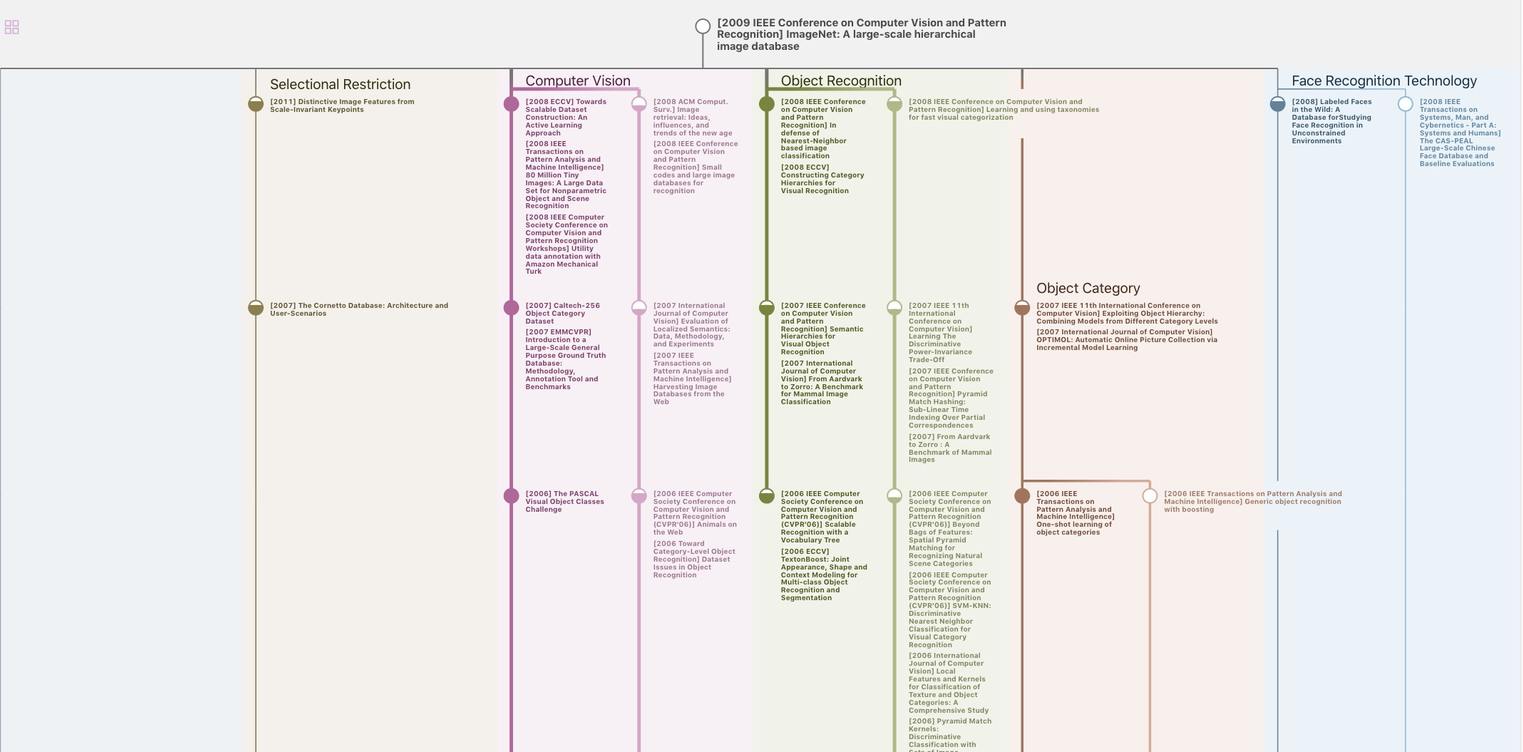
生成溯源树,研究论文发展脉络
Chat Paper
正在生成论文摘要