Dense 3D Model Reconstruction for Digital City Using Computationally Efficient Multi-View Stereo Networks.
IEEE International Geoscience and Remote Sensing Symposium (IGARSS)(2022)
摘要
Deep learning has shown promising results on dense three-dimensional (3D) model reconstruction from RGB images in recent years. However, the reconstruction of large-scale 3D models required for digital city remains very challenging even for such deep learning based methods. In this paper, we propose a convolutional neural network (CNN)-based Multi-View-Stereo (MVS) method that uses a double UNet approach searching for image features. The proposed network first utilizes a double U-Net to extract the image features of a coarser resolution for the sake of reduced memory requirements. After that, the cost volume is built via the differentiable homography warping. The cascade structure is designed to extract the information in a small-scale cost volume before a large-scale cost volume carries out fusion and finer depth map estimation. As a result, the proposed network can efficiently produce highly accurate 3D point clouds using a fraction of the GPU memory and runtime required by conventional methods. Extensive experiments on the DTU benchmarks as well as the Tanks and Temples benchmarks confirm that the proposed network can achieve outstanding reconstruction accuracy and model completeness.
更多查看译文
关键词
dense 3d model reconstruction,digital city,multi-view
AI 理解论文
溯源树
样例
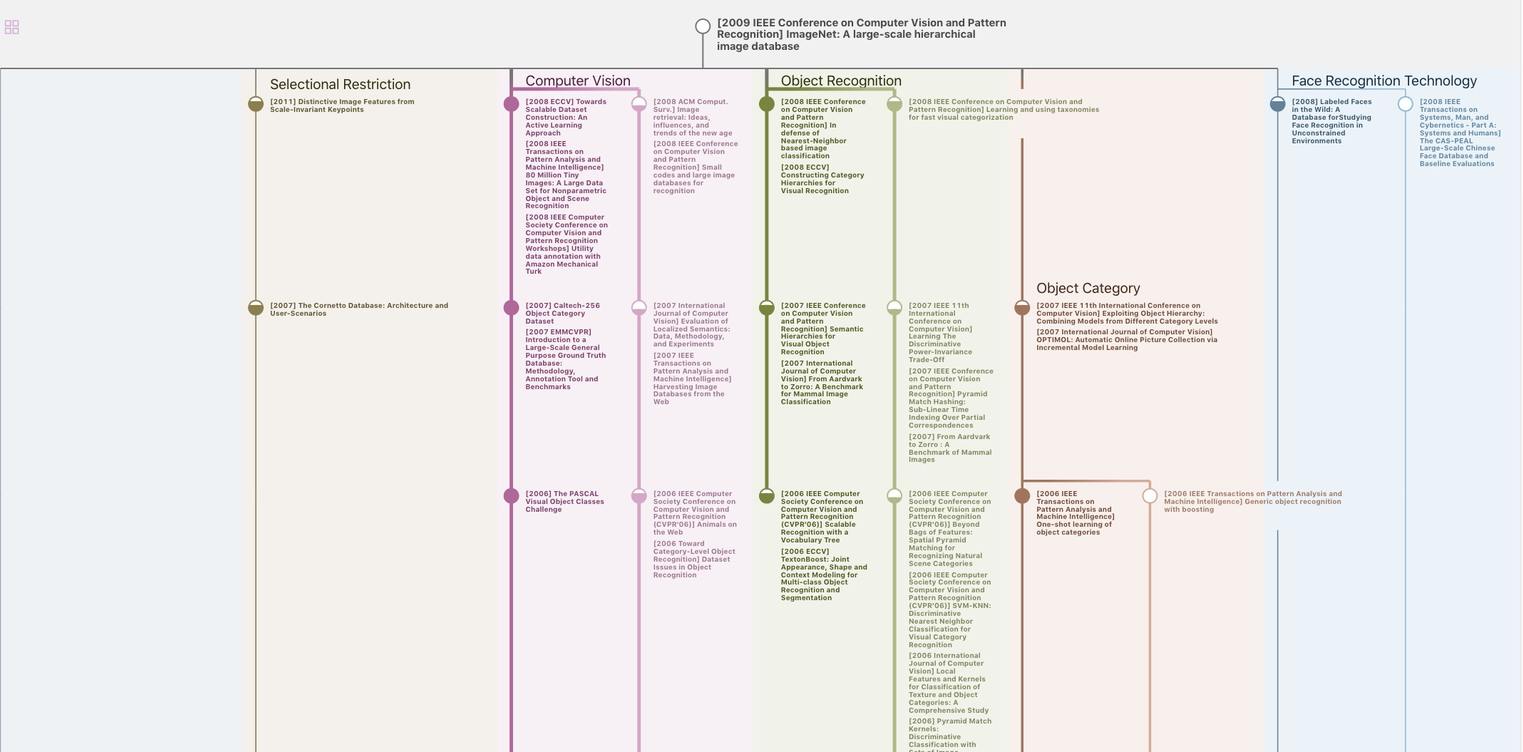
生成溯源树,研究论文发展脉络
Chat Paper
正在生成论文摘要