SD-DSAN: Saliency-Driven Dense Spatial Attention Network for Pan-Sharpening.
IEEE International Geoscience and Remote Sensing Symposium (IGARSS)(2022)
摘要
The demands for spectral and spatial quality in remote sensing (RS) images vary from region to region. Saliency detection is an effective tool to distinguish different regions with different demands. In this paper, we introduce saliency detection to satisfy these demands and propose a novel saliency-driven pan-sharpening network to further improve the fusion quality. Firstly, we combine foreground distribution with background prior to generate the initial saliency map, and implement least-square optimization to improve the detection accuracy. Then, we construct a dense spatial attention network trained through a new spatial-spectral-based loss function designed by saliency to meet diverse spectral and spatial needs of different regions. Thus, accurate fused images can be predicted. Experiments on SPOT-5 dataset indicate that our proposal has excellent properties with respect to the unified spatial-spectral quality against state-of-the-art methods.
更多查看译文
关键词
Pan-sharpening,spatial-spectral-based loss,remote sensing,residual network,saliency detection
AI 理解论文
溯源树
样例
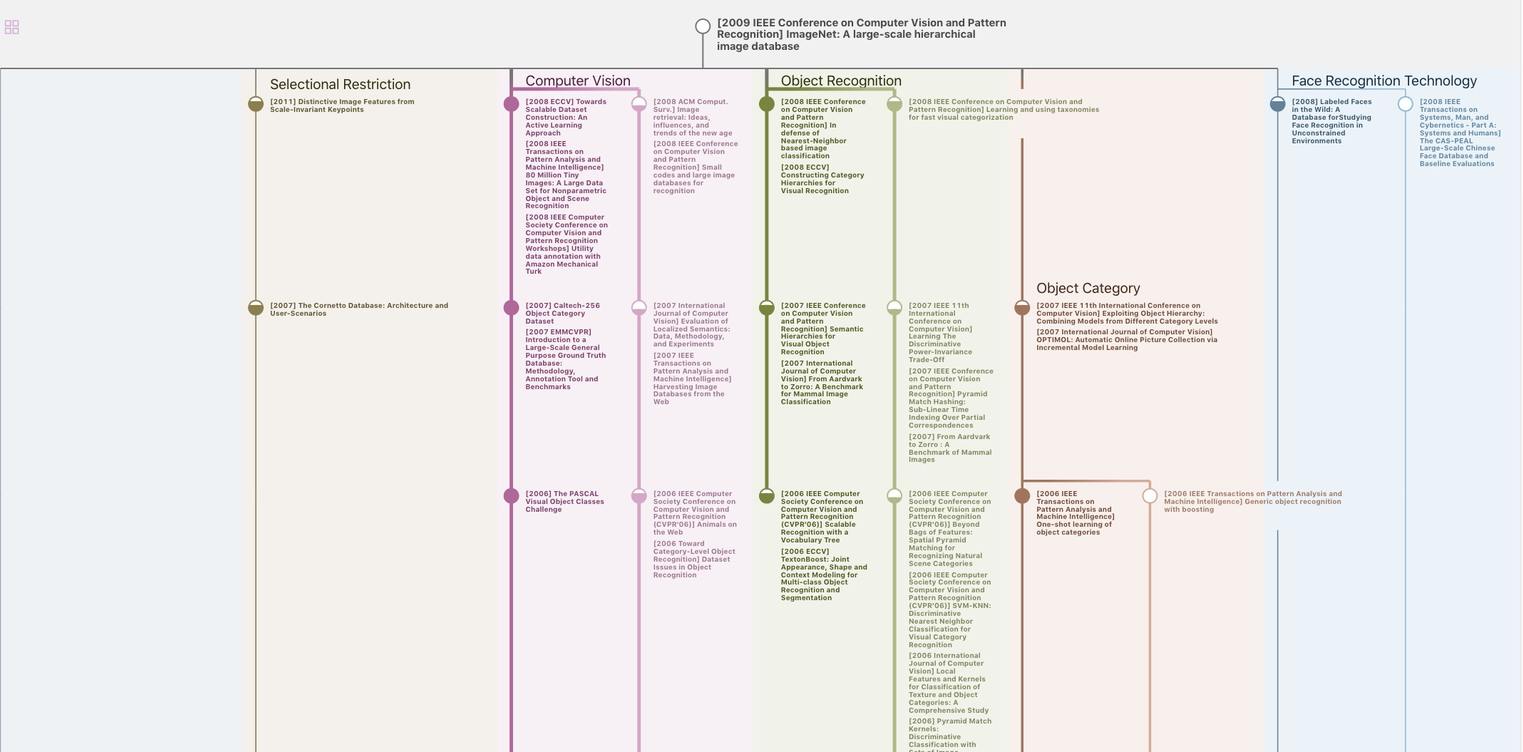
生成溯源树,研究论文发展脉络
Chat Paper
正在生成论文摘要