MSBRNet: Multi-Scale Background Reconstruction Network with Low-Rank Embedding for Anomaly Detection in Hyperspectral Images.
IEEE International Geoscience and Remote Sensing Symposium (IGARSS)(2022)
摘要
The primary purpose of anomaly detection in hyperspectral images (HSI) is to detect different anomaly targets from their surrounding backgrounds. Recently, anomaly detection has been well developed by deep learning technology. However, previous works utilize deep neural networks as a feature extractor followed by a traditional detector to detect anomalies, which cannot separate background and anomaly effectively. In this paper, we try to extract low-rank background features using neural networks to make full use of the low-rank properties of the background and then reconstruct the background with these features to directly separate the anomaly from the background, so we propose an multi-scale background reconstruction network with low-rank embedding (MSBRNet) for anomaly detection in HSI. Firstly, we use a low-rank background features extraction module (LBM) to extract low-rank background features. Then the background is reconstructed using a multi-scale background reconstruction module (MBRM). Finally, we calculate the mean square error of the input image and the output background to measure the effect of background reconstruction, and anomalies appear as reconstruction errors. Experiments on two publicly available experimental datasets demonstrate the significant advantage of the proposed method over other competitors.
更多查看译文
关键词
hyperspectral images,anomaly detection,background,multi-scale,low-rank
AI 理解论文
溯源树
样例
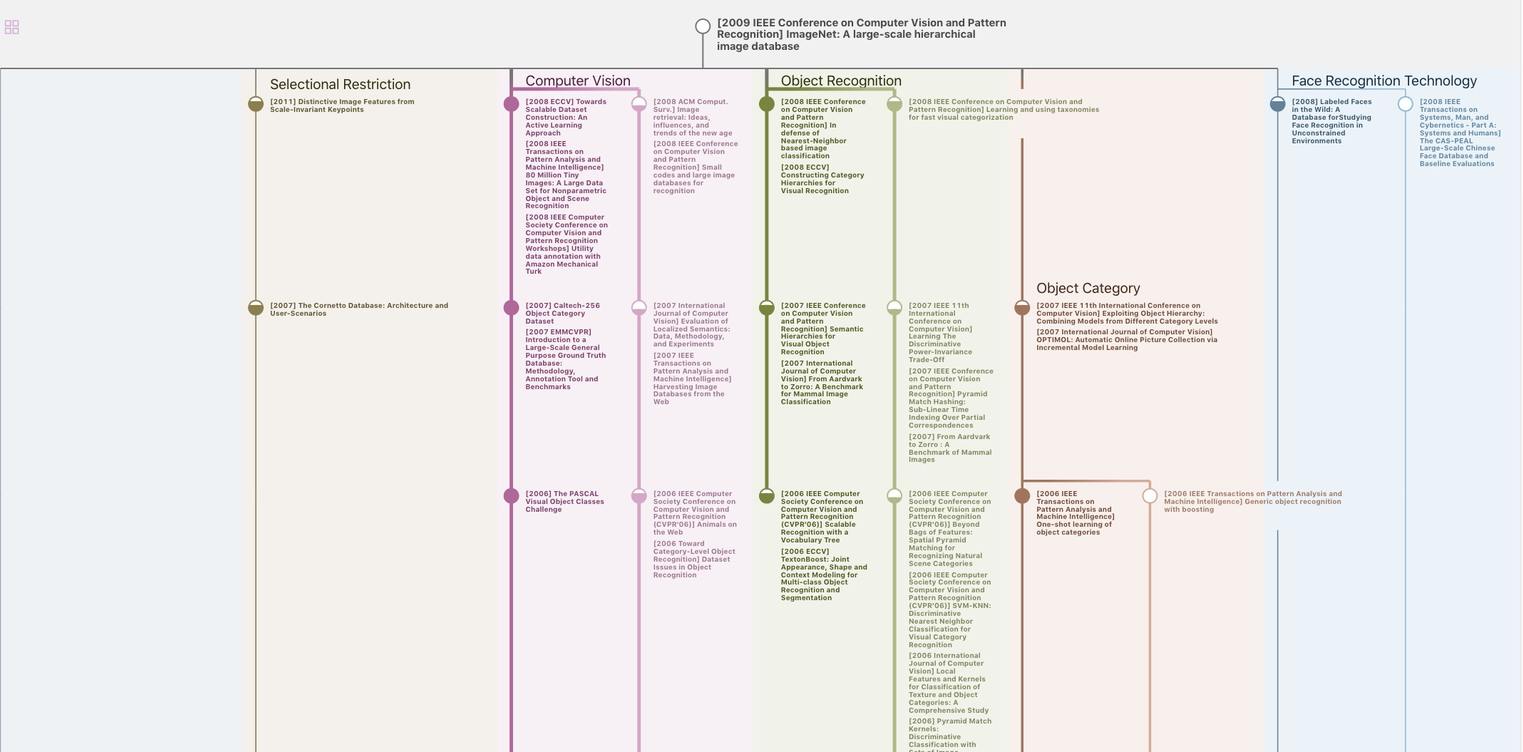
生成溯源树,研究论文发展脉络
Chat Paper
正在生成论文摘要