Semi-Supervised Semantic Segmentation of SAR Images Based on Cross Pseudo-Supervision
IEEE International Geoscience and Remote Sensing Symposium (IGARSS)(2022)
摘要
Due to the unique imaging mechanism and wide application of synthetic aperture radar (SAR), SAR image interpretation has been researched by more and more scholars. The supervised SAR image semantic segmentation methods that based on deep learning require a large number of accurate pixel-level labels, which are very hard to obtain. The lack of labeled samples limits the practical application of deep learning methods in SAR image semantic segmentation. To reduce the requirement of labeled data, we decided to introduce the cross pseudo-supervision network (CPS-Net) into SAR image semi-supervised semantic segmentation and promote the development of semi-supervised learning in SAR image interpretation. The semi-supervised segmentation based on CPS-Net has the following advantages: (1) CPS-Net encourages high similarity between two networks with the same input data, which helps improve the performance. (2) CPS-Net can make better use of the pseudo-supervision of unlabeled data to guide the network training. Experimental results show that CPS-Net achieves excellent semi-supervised semantic segmentation results on Sentinel-1 dual-polarization data with less labeled data. Compared with well-known semantic segmentation methods U-Net and DeeplabV3+, the performance of SAR image segmentation is significantly improved.
更多查看译文
关键词
SAR, Semi-supervised, Semantic segmentation, Cross pseudo-supervision network
AI 理解论文
溯源树
样例
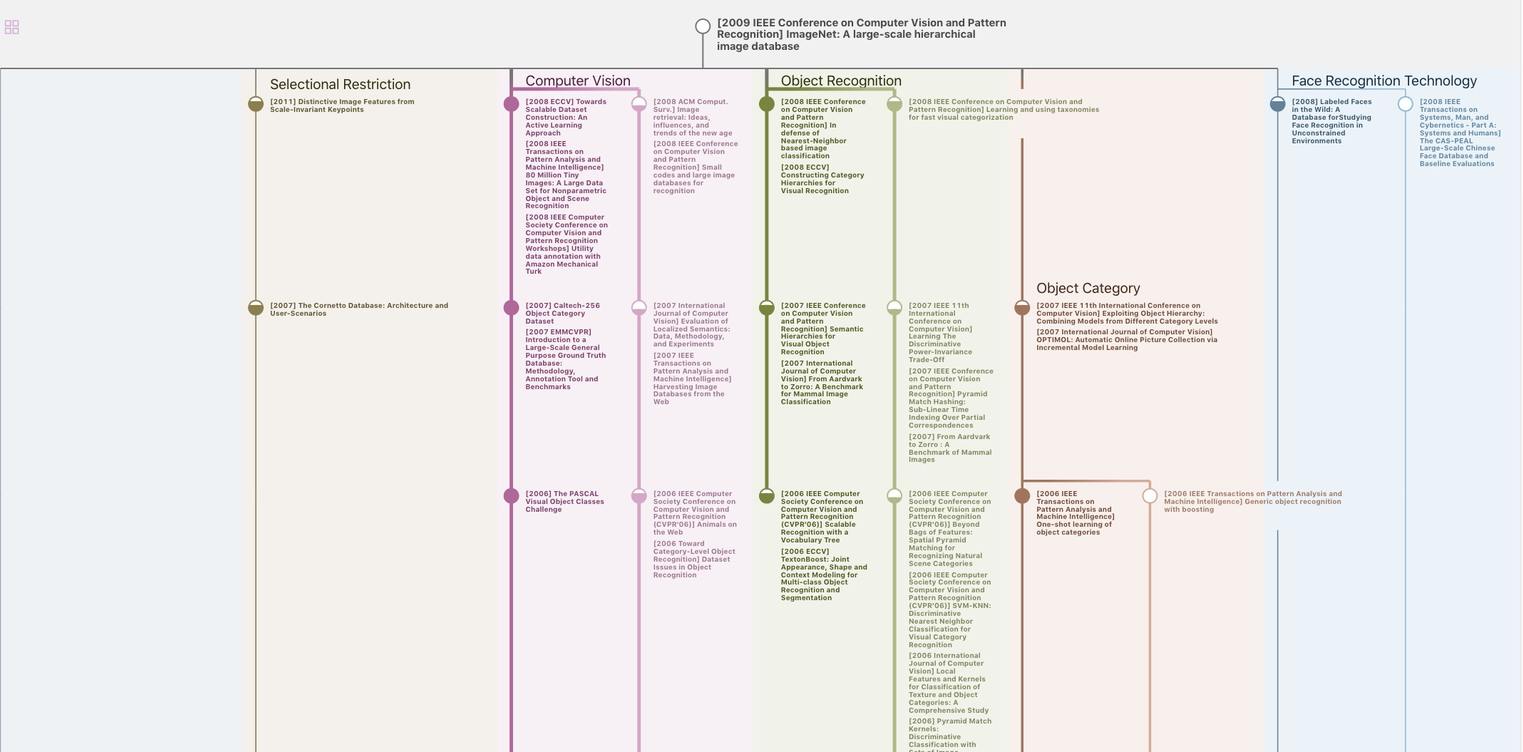
生成溯源树,研究论文发展脉络
Chat Paper
正在生成论文摘要