Efficient Use of Interferometric Coherence for Improvement in Classification of Urban and Tall Vegetation with SAR Data
IGARSS 2022 - 2022 IEEE International Geoscience and Remote Sensing Symposium(2022)
摘要
Classification of synthetic aperture radar data has been attempted by researchers in the past for several years, with varying degrees of success. One of the most challenging problem in such classifications is the segregation of urban (built-up region) and tall vegetation (TV). This is due to lack of difference in their scattering power. Therefore, an attempt has been made to improve urban and TV classification accuracy by exploring the use of Sentinel-1 interferometric coherence images as an additional feature along with other popularly used scattering features. A convolutional neural network (CNN) is trained on these features to obtain a good accuracy for classifying urban, TV and other classes. Obtained results are analyzed in both visual and quantitative ways. It is observed that when interferometric coherence images are added as an additional input feature in the CNN, producer and user accuracy for the urban and TV are improved from 15 to 25%, and overall accuracy(OA) is reached from 72.5% to 79.3%. Results oabtained with the CNN model is also compared with the traditional machine learning models such as support vector machine(SVM) and k-nearest neighbors(KNN). CNN over performes with 79.3% OA, followed by KNN with 66% OA while the performance of SVM is the worst among them all with 60% OA.
更多查看译文
关键词
Classification,CNN,Interferometric coherence,Synthetic Aperture Radar (SAR),Sentinel-1
AI 理解论文
溯源树
样例
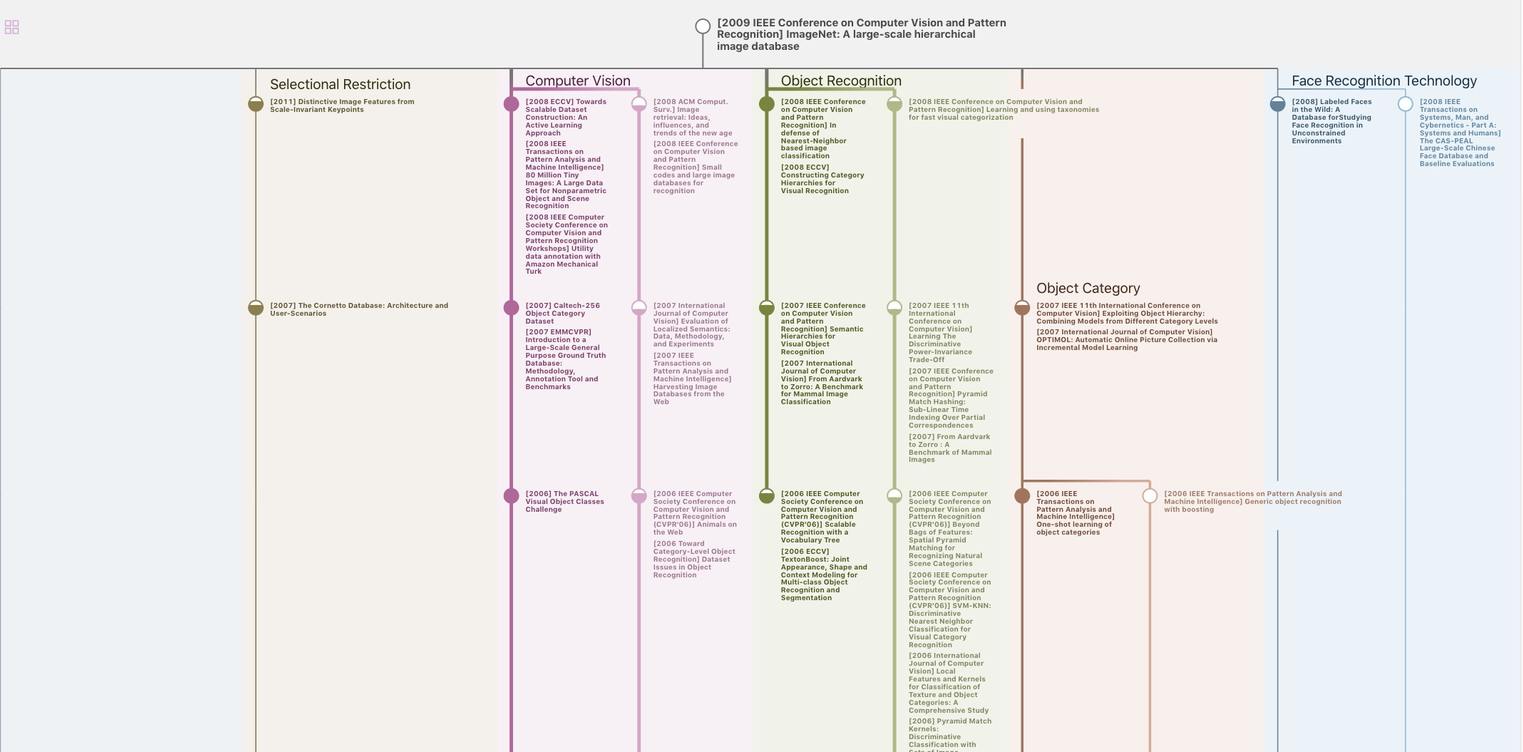
生成溯源树,研究论文发展脉络
Chat Paper
正在生成论文摘要