A Deep Learning Weather Model for Precipitation Nowcasting over China.
IEEE International Geoscience and Remote Sensing Symposium (IGARSS)(2022)
摘要
Precipitation is the critical components in the hydrological cycle over the Earth. Accurate and reliable high-resolution precipitation nowcasting with up to two hours ahead is a strong demand for many sectors that make decisions based on weather forecast. Due to the complexity of atmosphere, precipitation prediction is a long standing scientific challenge with direct impact on social and economic departments throughout the world. Numerical Weather Prediction(NWP) is the most common approach to provide people with weather information in near future based on the simulation of atmospheric dynamics based on physical laws, which usually comes across the “spin-up” problem and is hard to make full use of tremendous amount of Earth observation data that increase quickly as more and more observation instruments are being deployed around the world. The machine learning (ML) networks (DNN) witnessed remarkable progress in recent years due to increased amounts of available data, better model architectures and ease of implementation on powerful specialized hardware such as GPU and TPUs (Sφnderby 2020; Jouppi et al., 2017). Recently the DNN is now able to effectively process large time series spatial and temporal input data. Up on these developments of ML and hardware, we introduce China Artificial Intelligence Nowcasting system(CAINS) based on a deep learning model for precipitation nowcasting over China. As shown in Figure 1, at least 8 temporally continuous radar observed images are needed to drive CAINS to produce 60 (six-hour) precipitation images. Figure 2 shows the 6-hour precipitation nowcasting results with initial time at 20210513(Beijing time, BJT) 08:00. Figure 3 show the performance of nowcasting results by CAINS and Optical Flow(OF) algorithm in terms of Probability Of Detection (POD), Critical Success Index (CSI), and Equitable Threat Score(ETS). It is noted that CAINS outperform OF with pronouncedly better skills.
更多查看译文
关键词
deep learning weather model,critical components,hydrological cycle,reliable high-resolution precipitation,weather forecast,precipitation prediction,long standing scientific challenge,social departments,economic departments,weather information,atmospheric dynamics,physical laws,spin-up problem,Earth observation data,observation instruments,machine learning networks,DNN,increased amounts,model architectures,powerful specialized hardware,time series,temporal input data,deep learning model,8 temporally continuous radar,precipitation images,6-hour precipitation nowcasting results,Critical Success Index
AI 理解论文
溯源树
样例
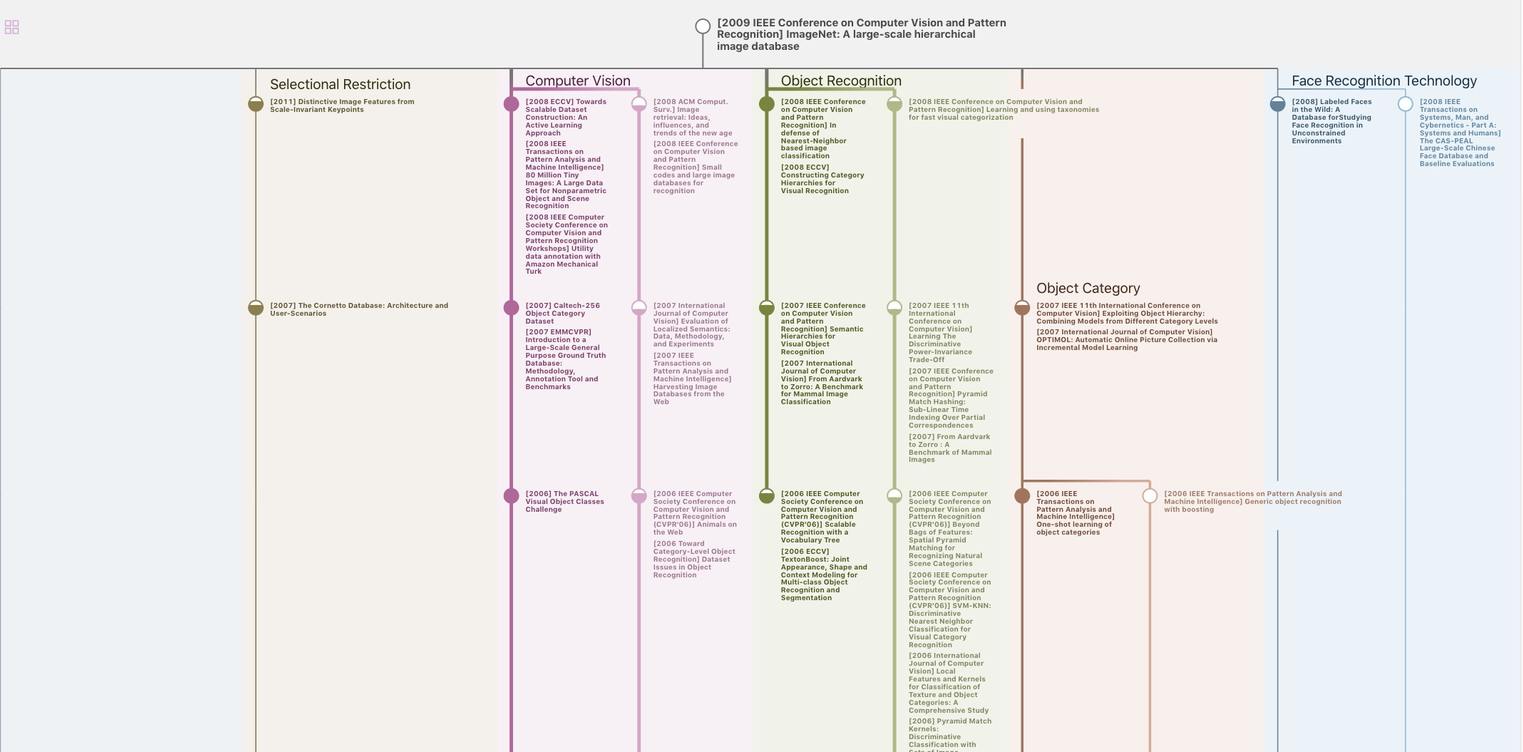
生成溯源树,研究论文发展脉络
Chat Paper
正在生成论文摘要