Quantum Convolutional Circuits for Earth Observation Image Classification.
IEEE International Geoscience and Remote Sensing Symposium (IGARSS)(2022)
摘要
The amount of study on Quantum Machine Learning (QML) is increasing extensively due to its potential advantages in terms of representational power and computational resources. These advances suggest a possibility to extend its usage into the context of Earth Observations, where Machine Learning (ML) plays an important role due to its extensive amount of data to be manipulated. This paper presents our preliminary results of binary quantum classifiers, which consist of Quantum Convolutional Neural Networks (QCNNs), applied on Earth Observation datasets, EuroSAT and SAT4, with classically-reduced features. Especially, we compare the performance of different data embedding techniques and quantum circuits for binary classification tasks.
更多查看译文
关键词
Quantum Convolutional circuits,Earth Observation image classification,Quantum Machine Learning,QML,representational power,computational resources,Earth Observations,binary quantum classifiers,Quantum Convolutional Neural Networks,Earth Observation datasets,classically-reduced features,quantum circuits,binary classification tasks
AI 理解论文
溯源树
样例
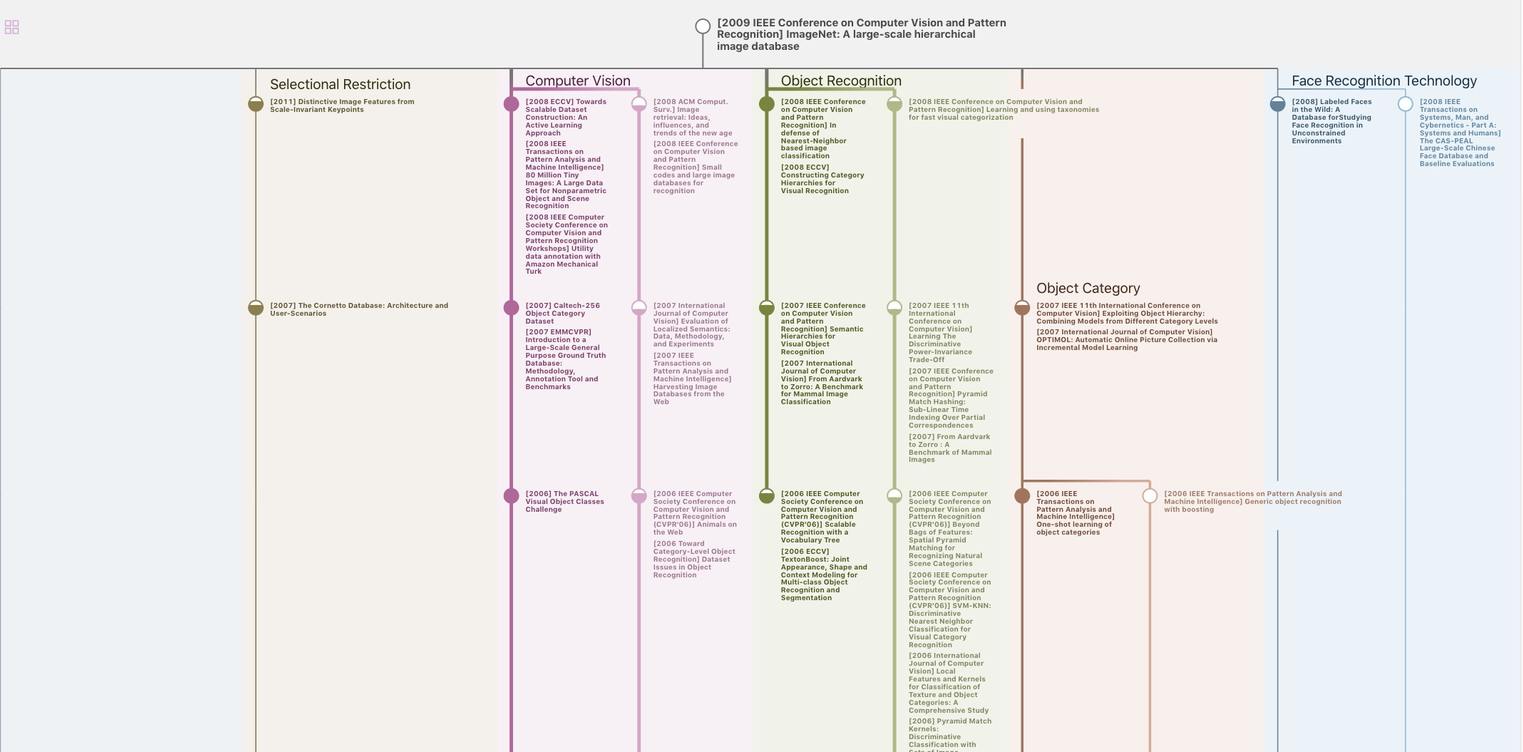
生成溯源树,研究论文发展脉络
Chat Paper
正在生成论文摘要