Deep Learning Models in Forest Mapping Using Multitemporal SAR and Optical Satellite Data.
IEEE International Geoscience and Remote Sensing Symposium (IGARSS)(2022)
摘要
In this study, we evaluate the potential of deep learning models in predicting forest tree height in boreal forest zone using ESA Sentinel-1 and Sentinel-2 images. The performance of studied deep learning models is compared to several popular conventional machine learning approaches. The study area is located near Hyytiala forestry station in Finland, and represents a conifer-dominated mixed boreal forestland. Improved predictions were obtained when using combined optical and SAR data for all studied models. Our results indicate that UNet based models can achieve better accuracy in predicting forest tree heights (RMSE of 1.90m, R-2 of 0.69), compared to traditional parametric and machine learning models with RMSE range of 2.27-2.41m and R-2 range of 0.50-0.56 when satellite optical and radar data are combined.
更多查看译文
关键词
forest mapping,multitemporal sar,deep learning
AI 理解论文
溯源树
样例
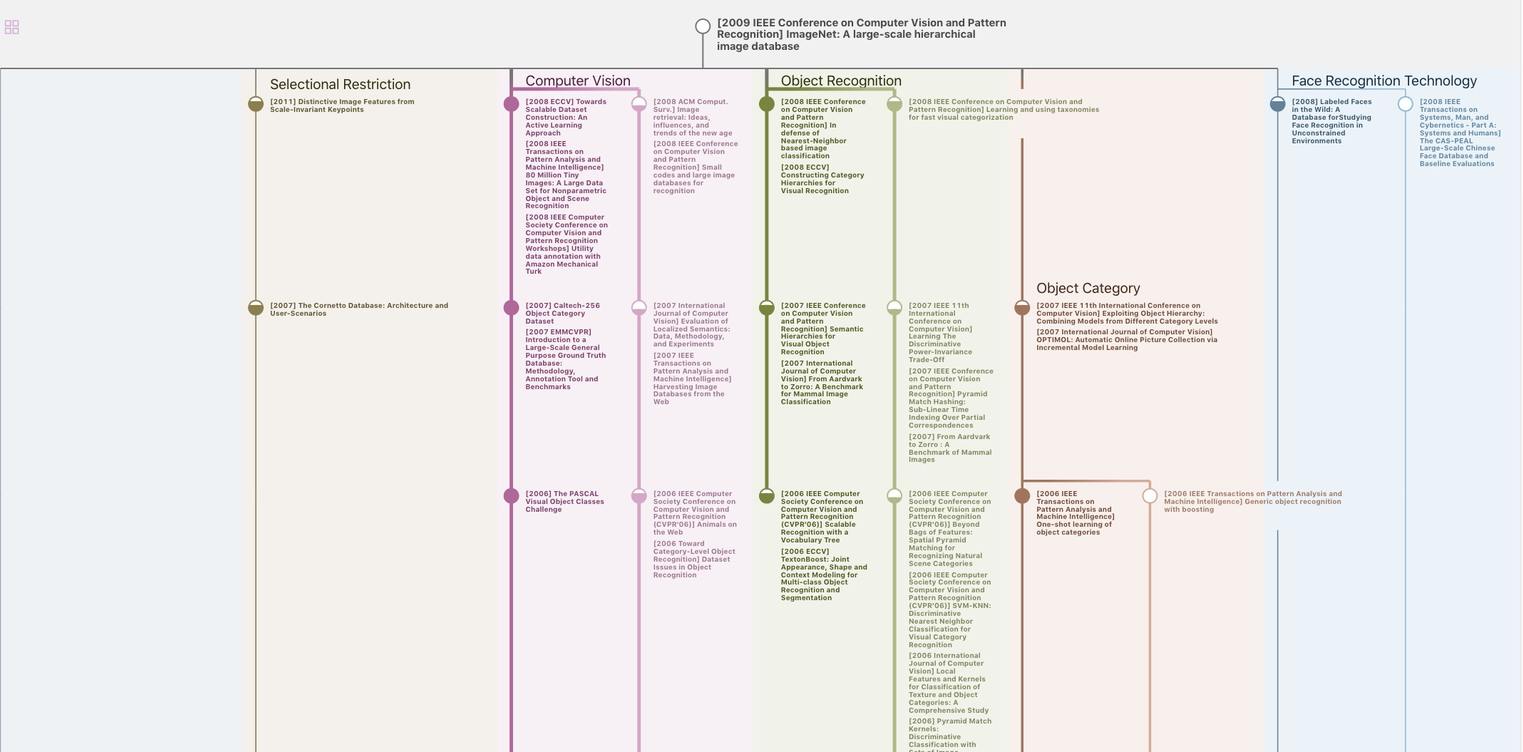
生成溯源树,研究论文发展脉络
Chat Paper
正在生成论文摘要