Evaluation of four Spatiotemporal Gap-Filling Methods in Crop Phenology Monitoring.
IEEE International Geoscience and Remote Sensing Symposium (IGARSS)(2022)
摘要
The high spatial resolution land surface phenology (LSP) monitoring is often limited by the temporal discontinuity of high spatial resolution observations. Many gap-filling methods have been proposed for LSP monitoring, however, a thorough intercomparison and evaluation is still lacking. Four widely-used methods, including the Spatial and Temporal Adaptive Reflectance Fusion Model (STARFM), the Flexible Spatiotemporal DAta Fusion (FSDAF), Multi-year based model, and Spatiotemporal Shape Matching Model (SSMM) were selected to extract green-up date (GUD) based on the Harmonized Landsat and Sentinel-2 (HLS) dataset. The results of the four methods show consistency with those of PhenoCam sites (R-2>0.64) and there is a lag phenomenon. Compared within 3 x 3 VIIRS pixel window, the difference between mean VIIRS GUDs and aggregated 30m GUDs is small and the mean absolute difference is less than 7 days. Comprehensively, SSMM has high consistency (R-2=0.75) and smaller bias (Bias=7.3days), which shows more potential in LSP monitoring.
更多查看译文
关键词
crop,gap-filling
AI 理解论文
溯源树
样例
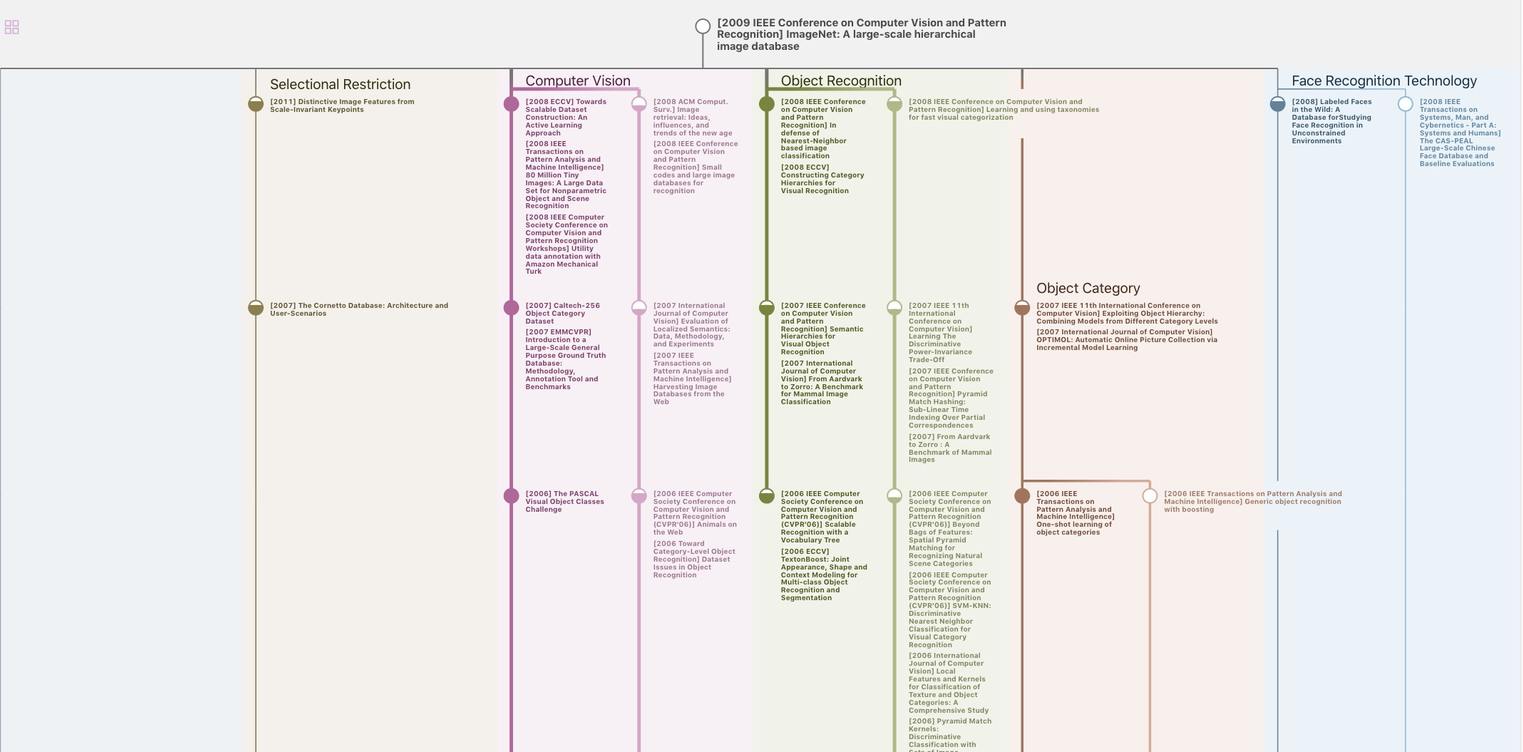
生成溯源树,研究论文发展脉络
Chat Paper
正在生成论文摘要