Accurate Cloud Detection using Feature Refining Attention Network and S-NPP Cris Fsr Data.
IEEE International Geoscience and Remote Sensing Symposium (IGARSS)(2022)
摘要
Cloud detection is an important and challenging problem in atmospheric remote sensing. This study improves the cloud detection performance of the previously proposed cross-track infrared sounder (CrIS) full spectrum resolution (FSR) cloud detection index (FCDI) by changing the channel pairing criteria and using a new feature refining attention network (FRAnet). Instead of using the standard deviation of the simulated CrIS channel's brightness temperatures (BTs) as pairing index, this study adopts the root mean square error (RMSE) of the fitting results of the candidate pairs to select channel pairs. Since the channel's BT standard deviation mainly reflects the channel's reliability while the RMSE can directly reflect the pairing and fitting quality. Different RMSE thresholds are specified to represent the physical characteristics of different band groups, i.e., the long wave to short wave (LW-SW), LW to medium wave (MW), and MW-SW. After the new channel pairs being selected, the attention mechanism based FRAnet is applied to optimal utilize the FCDIs obtained from over 200 pairs. 40-day data is used for this study, and a precise algorithm is applied to match the CrIS data to the visible infrared imaging radiometer suite (VIIRS) data. As a result, new cloud labels are used. Simulation results show a largely improved cloud detection accuracy from the previously achieved similar to 80% to similar to 87.8%.
更多查看译文
关键词
CrIS, VIIRS, cloud detection, FCDI, deep learning, attention mechanism
AI 理解论文
溯源树
样例
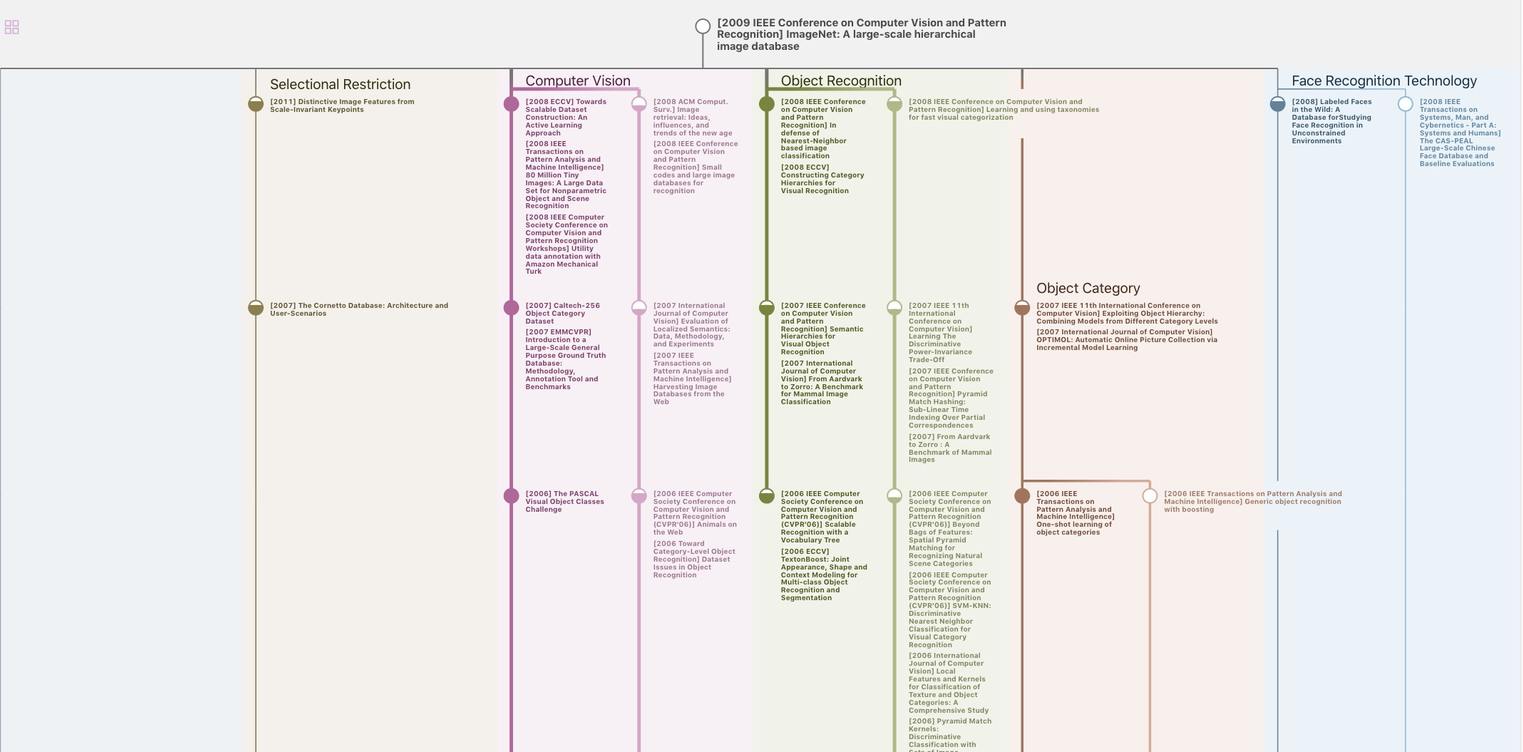
生成溯源树,研究论文发展脉络
Chat Paper
正在生成论文摘要