A Combination of Radiative Transfer Model Simulation and Neural Network Modeling for the Retrieval of Volcanic Ash Parameters by Means of Copernicus Sentinel-3/SLSTR Data.
IGARSS 2022 - 2022 IEEE International Geoscience and Remote Sensing Symposium(2022)
摘要
In this study we present a novel approach dedicated to the retrieval of volcanic ash parameters by means of data acquired from the Sea and Land Surface Temperature Radiometer on board of the Copernicus Sentinel-3. In this framework, we developed a procedure combining Radiative Transfer Model simulations and Neural Network for estimating three volcanic ash parameters such as aerosol optical depth, effective radius and ash mass. The Radiative Transfer Model simulations have been considered for producing synthetic training sets, which have been used in the training phase of the Neural Networks development. In particular, nine latitude's belts have been identified for training several Neural Networks ensuring the global coverage of the method. The approach has been tested by comparing the results of the trained NN with the ones obtained by applied the state-of-art Look Up Table and the Volcanic Plume Retrieval procedures. The results of the methodologies applied on Raikoke, 2019 eruption demonstrated the feasibility of the proposed approach by registering values of the correlation coefficient between all the three methods ranging between the 65% and the 94%.
更多查看译文
关键词
Radiative Transfer Model,Volcanic Ash Retrievals,SLSTR,Neural Networks,LUT,VPR
AI 理解论文
溯源树
样例
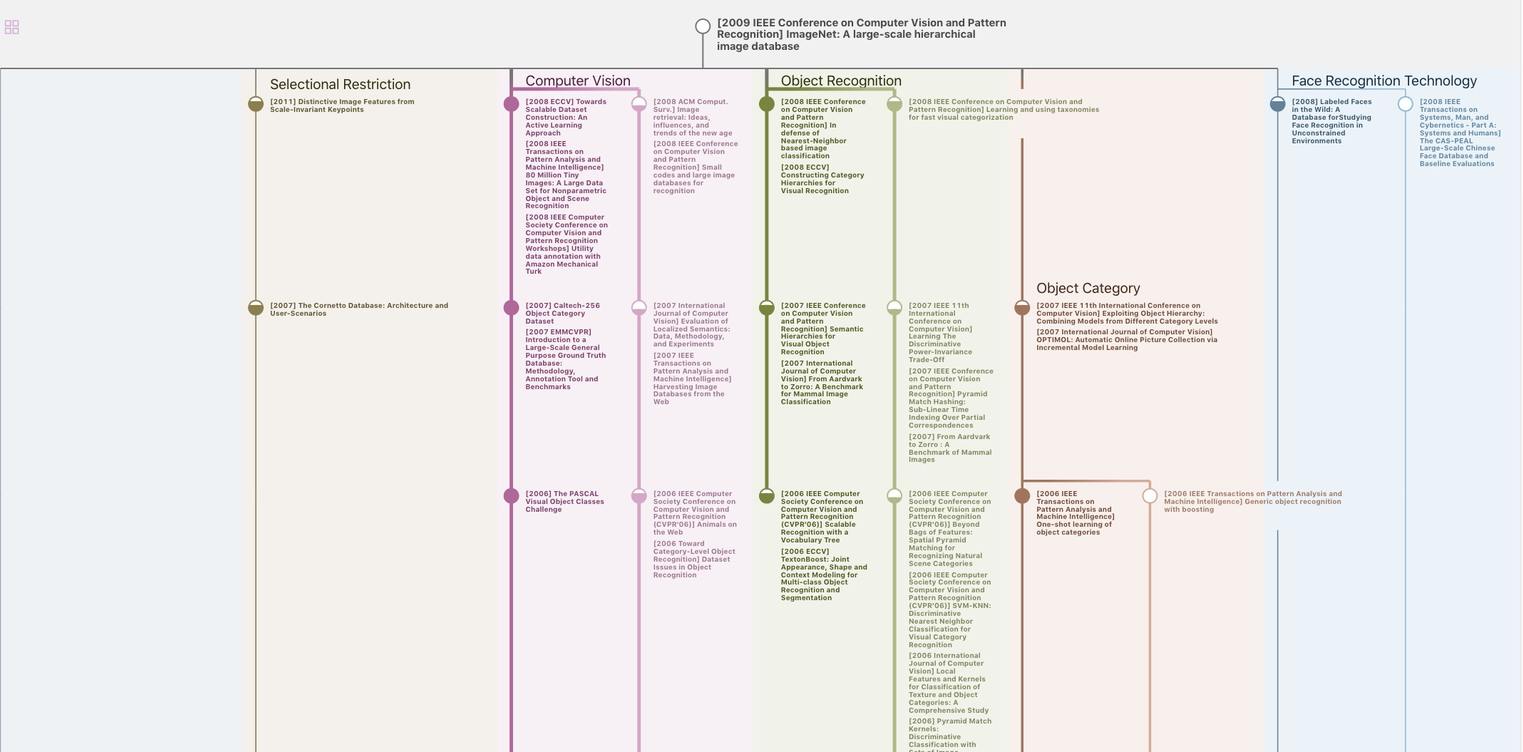
生成溯源树,研究论文发展脉络
Chat Paper
正在生成论文摘要