Real-time Attention-Augmented Spatio-Temporal Networks for Video-based Driver Activity Recognition.
International Conference on Intelligent Transportation Systems (ITSC)(2022)
摘要
Identifying driver behaviour and activities from in-cabin video cameras (especially the distracting non-driving activities), has been recently shown to be effective in enhancing the safety and the driving experience in smart and partiallyautomated vehicles. In the literature, the problem of videobased driver activity recognition is often tackled by using traditional deep learning-based human-action recognition systems. Despite their powerful capabilities, they seem not well-suited for video-based driver activity recognition, due to their complex and inefficient architecture that requires a huge amount of computational resources. Additionally, given the similarities of different non-driving activities that share the same pattern of upper body movements (e.g. drinking versus eating), it makes it harder for traditional human-action recognition systems to pick up or differentiate between these subtle changes. Thus, in this work we are proposing a novel framework based on an efficient spatio-temporal neural network architecture augmented with an attention mechanism that can differentiate between the subtle differences of similar non-driving activities. Our framework has been evaluated on one of the largest benchmark datasets for fine-grained recognition of driver activities and it has outperformed the state-of-art approach by more than 4% in the top-1 accuracy score with a boosting of 13x the run-time speedup during the inference.
更多查看译文
关键词
driver,real-time real-time,attention-augmented,spatio-temporal,video-based
AI 理解论文
溯源树
样例
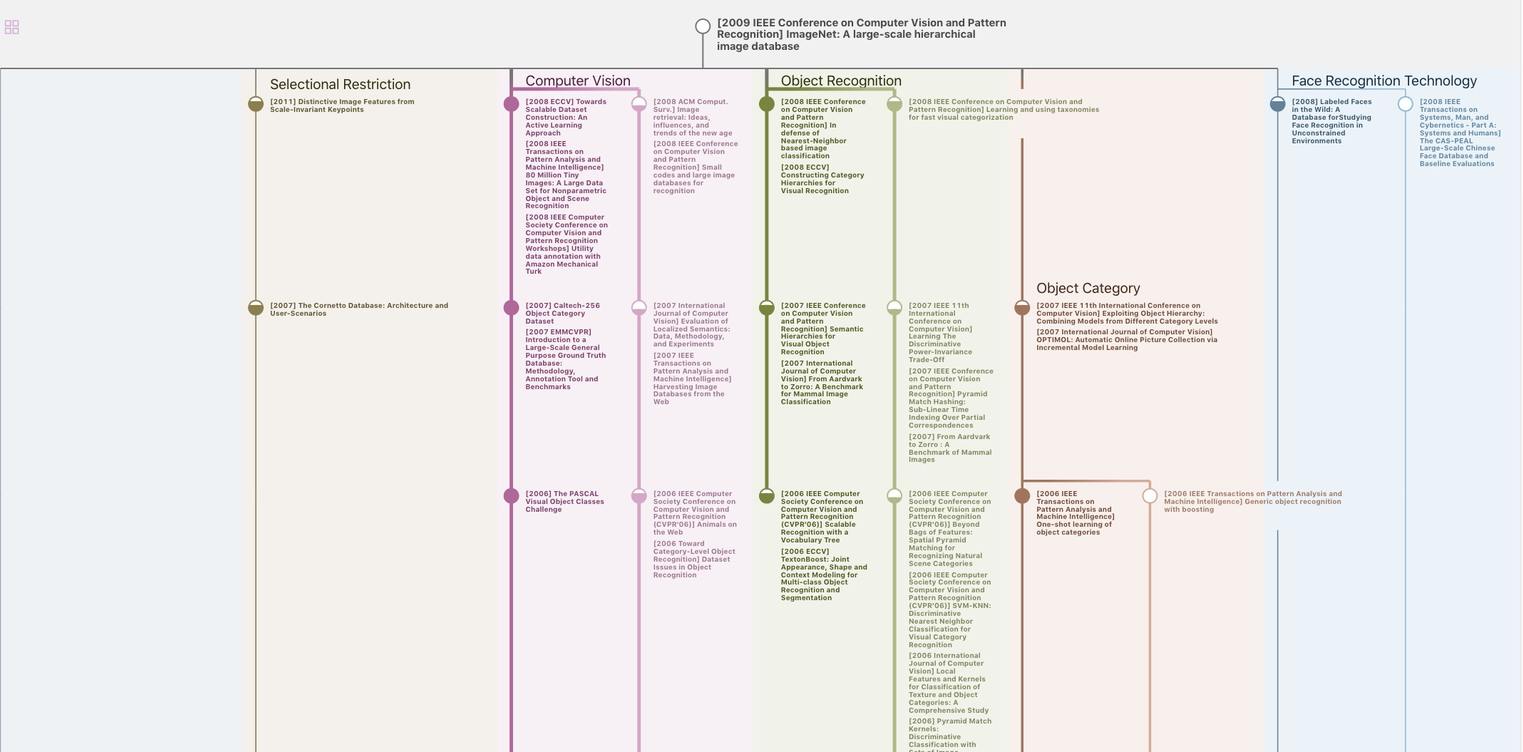
生成溯源树,研究论文发展脉络
Chat Paper
正在生成论文摘要