Safe Reinforcement Learning for Urban Driving using Invariably Safe Braking Sets.
International Conference on Intelligent Transportation Systems (ITSC)(2022)
摘要
Deep reinforcement learning (RL) has been widely applied to motion planning problems of autonomous vehicles in urban traffic. However, traditional deep RL algorithms cannot ensure safe trajectories throughout training and deployment. We propose a provably safe RL algorithm for urban autonomous driving to address this. We add a novel safety layer to the RL process to verify the safety of high-level actions before they are performed. Our safety layer is based on invariably safe braking sets to constrain actions for safe lane changing and safe intersection crossing. We introduce a generalized discrete high-level action space, which can represent all high-level intersection driving maneuvers and various desired accelerations. Finally, we conducted extensive experiments on the inD dataset containing urban driving scenarios. Our analysis demonstrates that the safe agent never causes a collision and that the safety layer's lane changing verification can even improve the goalreaching performance compared to the unsafe baseline agent.
更多查看译文
关键词
urban autonomous driving,safety layer,RL process,high-level actions,invariably safe braking sets,safe lane,safe intersection crossing,generalized discrete high-level action space,high-level intersection,urban driving scenarios,safe agent,safe reinforcement learning,deep reinforcement learning,motion planning problems,autonomous vehicles,urban traffic,traditional deep RL algorithms,safe trajectories,provably safe RL algorithm
AI 理解论文
溯源树
样例
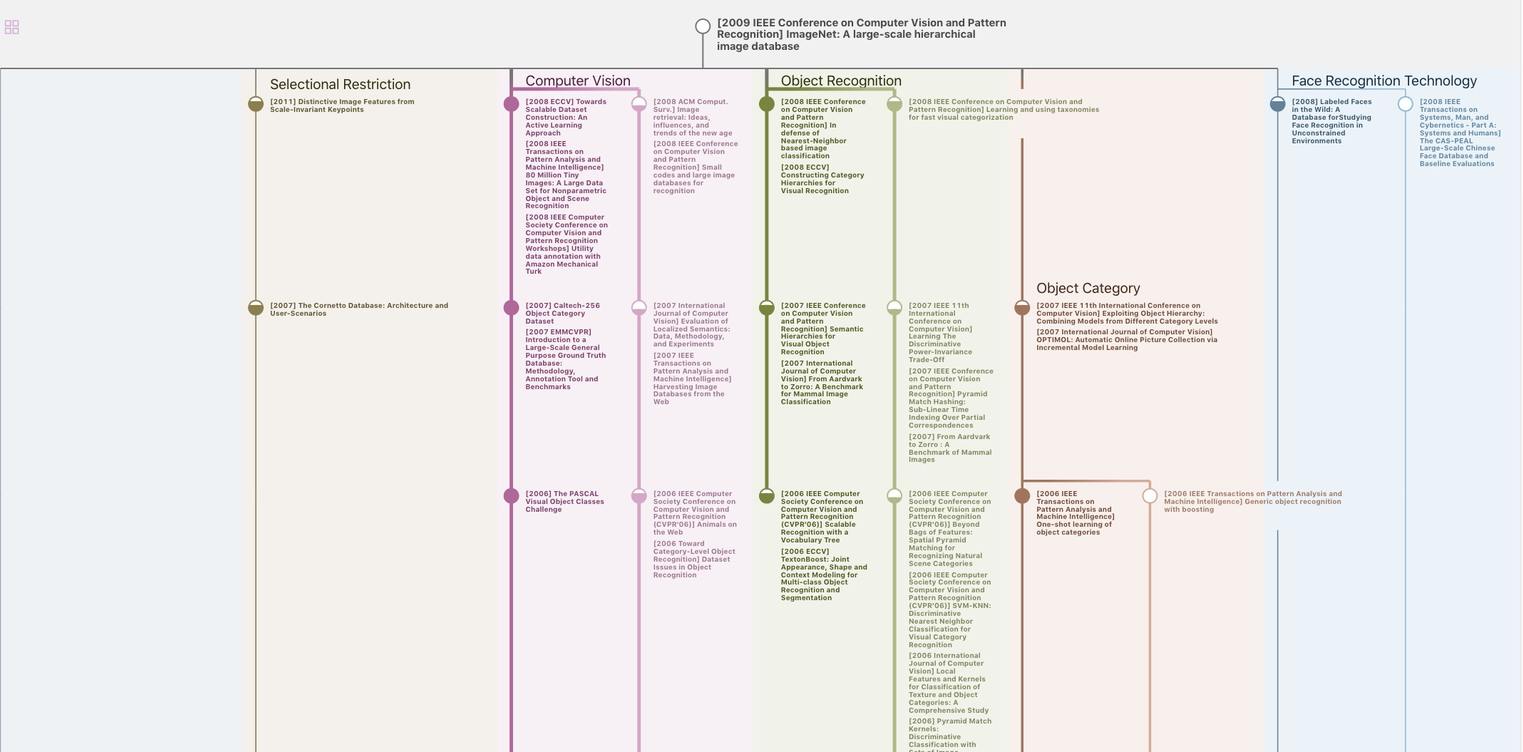
生成溯源树,研究论文发展脉络
Chat Paper
正在生成论文摘要