Using Vehicle Data to Enhance Prediction of Accident-Prone Areas
International Conference on Intelligent Transportation Systems (ITSC)(2022)
摘要
Detection of accident-prone areas in road networks is crucial to increase road safety and reduce accident risk. Traditionally, statistical methods and, more recently, neural networks have been applied to identify accident-prone road network areas and reduce crash rates. However, these approaches rely on historical accident data as input, often unavailable in urban regions. Furthermore, nearly accidents are not part of statistical accident records; however, vehicle braking and acceleration data can reveal risk areas with frequent nearly accidents. In this paper, we propose a novel risk area detection approach, which examines an entire city and detects accident prone-areas by adopting a convolutional neural network to vehicle data. This deep learning method leverages various features extracted from car fleet data, including acceleration and braking signals, together with traffic lights, road networks, and point-of-interest data. We evaluate our approach against several established machine learning algorithms, including linear regression, support vector machines, and an artificial neural network. Our experiments on real-world data demonstrate that the proposed approach outperforms the baselines in discriminating accident-prone areas from safer ones in terms of accuracy, precision, recall, and F1 score.
更多查看译文
关键词
vehicle data,prediction,areas,accident-prone
AI 理解论文
溯源树
样例
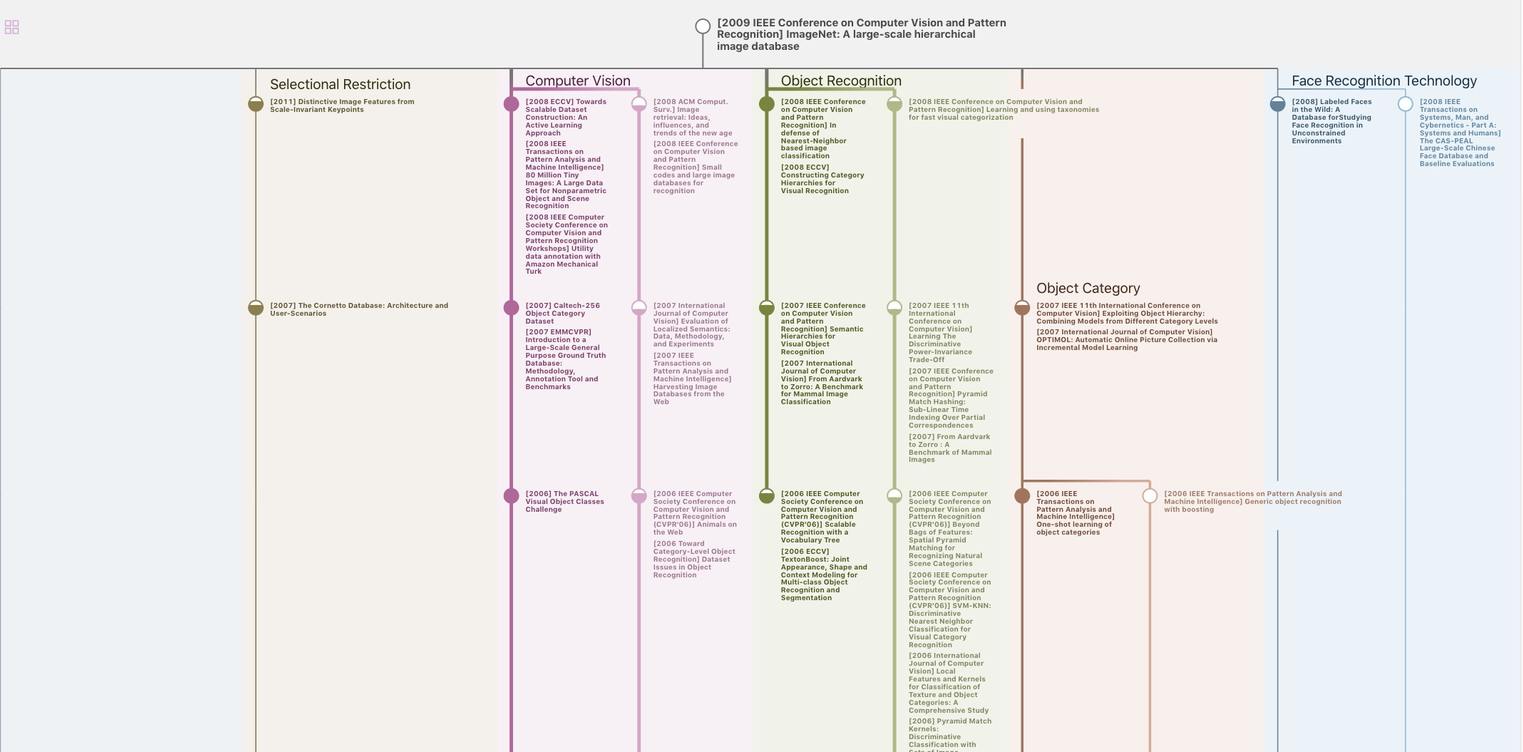
生成溯源树,研究论文发展脉络
Chat Paper
正在生成论文摘要