Coalitional Fairness of Autonomous Vehicles at a T-Intersection.
International Conference on Intelligent Transportation Systems (ITSC)(2022)
摘要
Currently, autonomous vehicles (AVs) generally make decisions in their self-interest instead of the social good. Although humans already commit such actions, a decision made by an AV is directly connected to a company allowing potential unfair actions to occur on a large scale with far worse consequences. To explore fairness, this paper analyzes the efficiency and group fairness of AVs' interactions at a simplified garage Tintersection. To portray the current and future presence of AVs, mixed autonomy and fully autonomous traffic are simulated by varying the percentages of AVs that belong to an AV company. This complex multi-agent planning problem is solved by utilizing SARSA, a model-free reinforcement learning (RL) algorithm, to learn the decision-making algorithm of an AV company. Rather than abide by society's traffic rules, the AVs followed a "selfish" policy p where they may block vehicles to benefit their coalition. The metrics used to evaluate the fairness of these actions and their effects on efficiency are the Difference in Group Fairness (DGF) and Change in Efficiency (CIE). With out a fairness regulation, following policy p may unintentionally be detrimental to efficiency and cause group fairness issues. Moreover, a policy learned from an RL algorithm is a black box, thus, AV makers utilizing such methods cannot simply inject a rule but need to consider implementing a regulation into their decision-making algorithm. Through reward shaping the unfairness of actions is restricted, resulting in improvements of mean CIE of up to 3.14% and 1.63% for the mixed and fully autonomous case meanwhile the mean DGF decreases by as much as -2.99% and -0.53%.
更多查看译文
关键词
group fairness,efficiency,autonomous vehicles
AI 理解论文
溯源树
样例
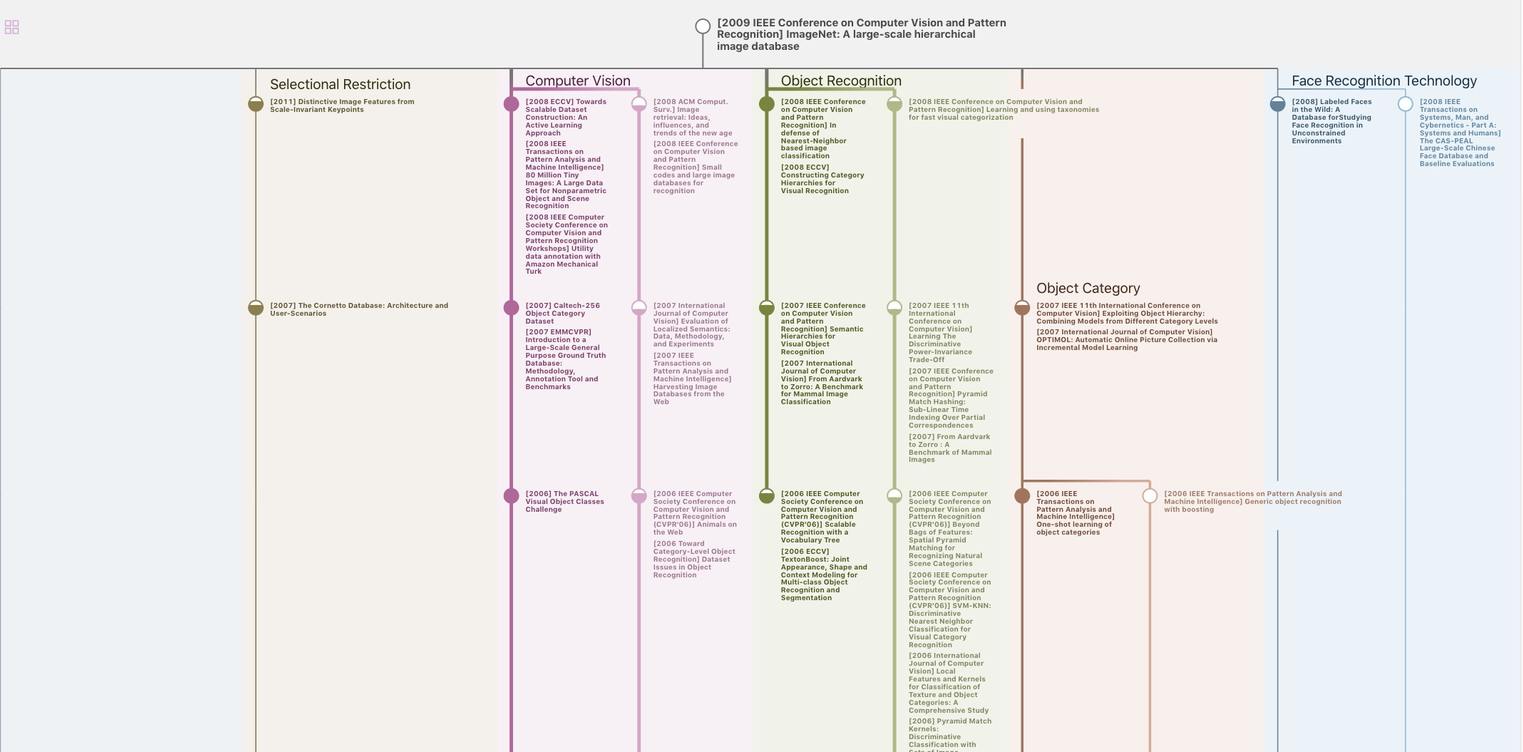
生成溯源树,研究论文发展脉络
Chat Paper
正在生成论文摘要