JointFlow: A Foot Motion Tracking Model Combining Pose Estimation Model with Optical Flow
2022 IEEE 25th International Conference on Intelligent Transportation Systems (ITSC)(2022)
摘要
Understanding driver's foot behavior helps reduce pedal-related traffic accidents. Conventional systems introduced wearable devices to track drivers' foot motion and tracking drivers' feet using non-contact sensors is still challenging. Moreover, to better understand driver's foot behavior, it is required to stably track drivers' small foot motion and at the joint level. To cope with this problem, we develop a novel foot motion tracking system, referred to as 'JointFlow.’ This system integrates a pose estimation model, i.e., OpenPose, with Optical Flow. At first, a keypoint region-based convolutional neural network (keypoint R-CNN) is trained to detect the joints of the foot. At the same time, the Lucas-Kanade algorithm of Optical Flow is used to calculate the motion of each foot joint between consecutive frames. We implemented and evaluated the system using a real-world driving dataset of 50 drivers. The evaluation result shows that JointFlow could track both small and large foot motion. By comparing with the conventional pose estimation model, we could confirm that JointFlow tracked small foot motion more stable.
更多查看译文
关键词
foot motion tracking model combining pose estimation model,Optical Flow,understanding driver,pedal-related traffic accidents,track drivers,foot motion tracking system,JointFlow,keypoint region-based convolutional neural network,foot joint,conventional pose estimation model
AI 理解论文
溯源树
样例
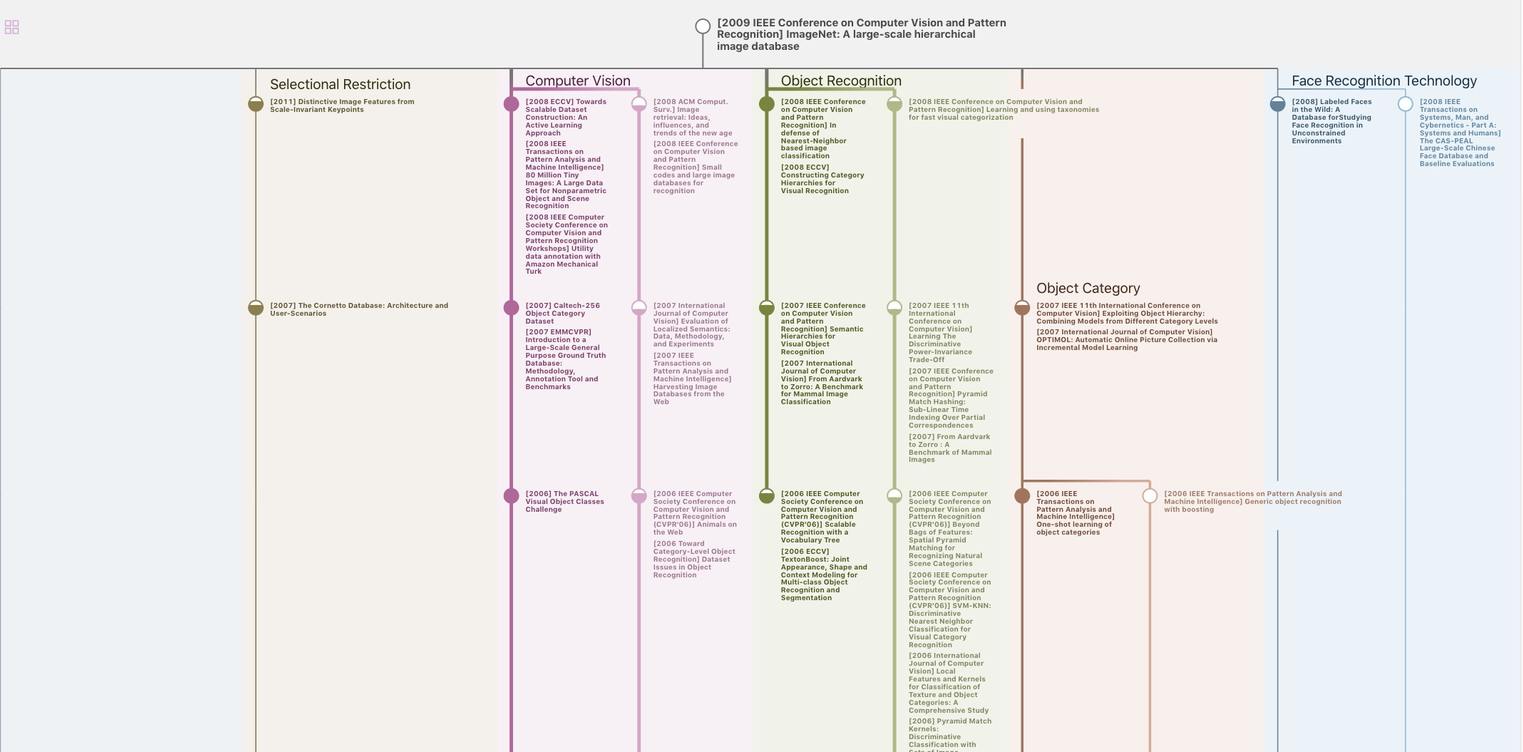
生成溯源树,研究论文发展脉络
Chat Paper
正在生成论文摘要