A Fuzzy-based Convolutional LSTM Network Approach for Citywide Traffic Flow Prediction
2022 IEEE 25th International Conference on Intelligent Transportation Systems (ITSC)(2022)
摘要
Citywide traffic flow prediction is of great importance to intelligent transportation systems and smart cities. Although many deep learning methods have been applied for citywide traffic flow prediction, deep learning is a deterministic representation and sheds little light on data uncertainty. In this paper, a fuzzy-based convolutional LSTM neural network (FConvLSTM) method is proposed to improve the accuracy of citywide traffic flow prediction by taking data uncertainty into consideration. FConvLSTM is a hybrid model which combines fuzzy learning with a convolutional LSTM neural network (ConvLSTM). The impact of data uncertainty is lessened with the help of fuzzy neural networks and ConvLSTM is adopted to explore the spatio-temporal characteristics of traffic data, which can learn spatial dependencies and temporal dependencies jointly. Experimental results on a real dataset verify the outperformance of the FConvLSTM method.
更多查看译文
关键词
citywide traffic flow prediction,data uncertainty,fuzzy-based convolutional LSTM neural network method,fuzzy learning,FConvLSTM,ConvLSTM,spatio-temporal traffic data characteristics,spatial dependency learning,temporal dependency learning,deep learning
AI 理解论文
溯源树
样例
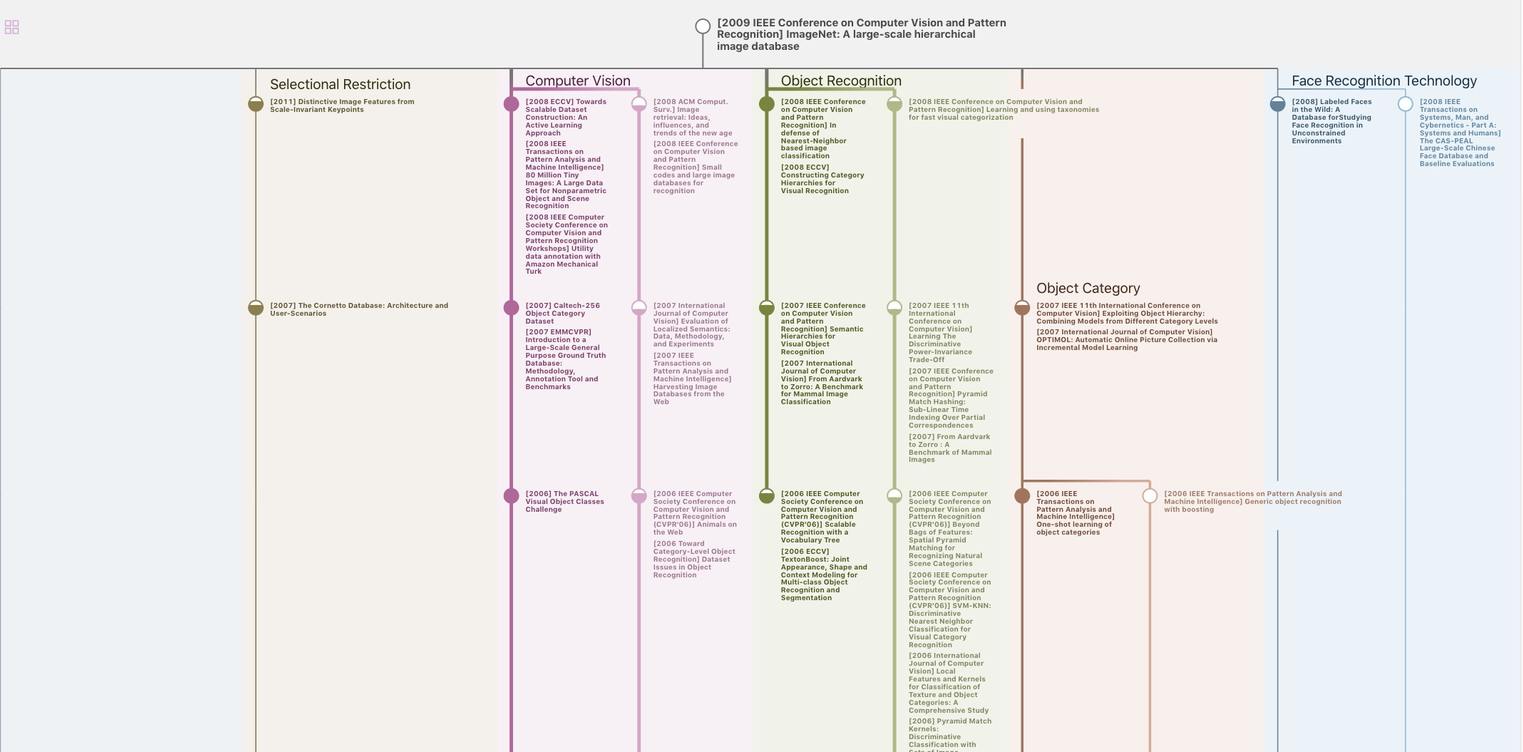
生成溯源树,研究论文发展脉络
Chat Paper
正在生成论文摘要