MultiLane: Lane Intention Prediction and Sensible Lane-Oriented Trajectory Forecasting on Centerline Graphs.
International Conference on Intelligent Transportation Systems (ITSC)(2022)
摘要
Forecasting the motion of surrounding traffic is one of the key challenges in the quest to achieve safe autonomous driving technology. Current state-of-the-art deep forecasting architectures are capable of producing impressive results. However, in many cases, they also output completely unreasonable trajectories, making them unsuitable for deployment. In this work, we present a deep forecasting architecture that leverages the map lane centerlines available in recent datasets to predict sensible trajectories; that is, trajectories that conform to the road layout, agree with the observed dynamics of the target, and react to the presence of surrounding agents. To model such sensible behavior, the proposed architecture first predicts the lane or lanes that the target agent is likely to follow. Then, a navigational goal along each candidate lane is predicted, allowing the regression of the final trajectory in a laneand goal-oriented manner. Our experiments in the Argoverse dataset show that our architecture achieves performance onpar with lane-oriented state-of-the-art forecasting approaches and not far behind goal-oriented approaches, while consistently producing sensible trajectories.
更多查看译文
关键词
lane intention prediction,sensible lane-oriented trajectory forecasting,centerline graphs,safe autonomous driving technology,current state-of-the-art deep forecasting architectures,output completely unreasonable trajectories,deep forecasting architecture,map lane centerlines,sensible trajectories,surrounding agents,sensible behavior,target agent,candidate lane,final trajectory,goal-oriented manner,lane-oriented state-of-the-art forecasting approaches,goal-oriented approaches
AI 理解论文
溯源树
样例
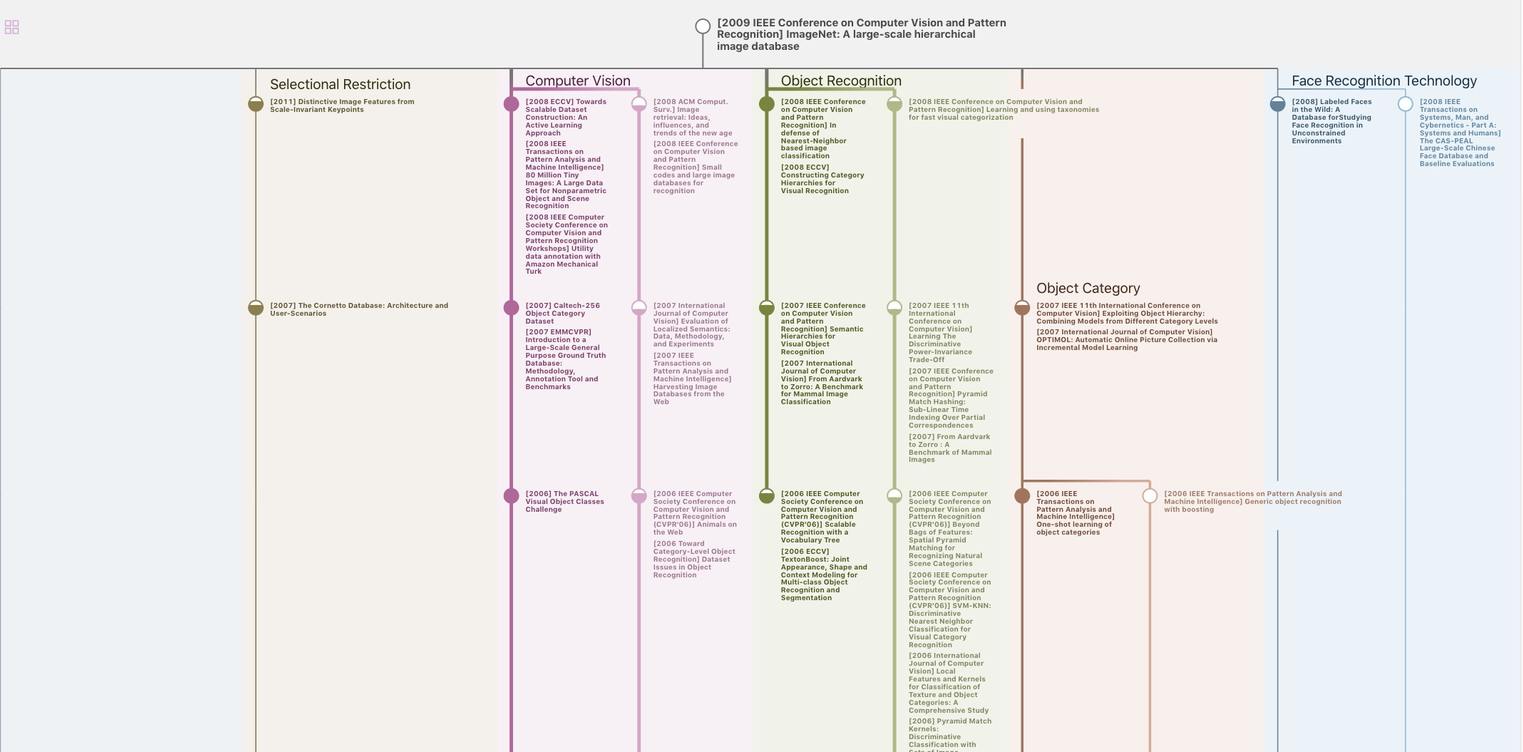
生成溯源树,研究论文发展脉络
Chat Paper
正在生成论文摘要