Evaluating Pre-training Objectives for Low-Resource Translation into Morphologically Rich Languages.
International Conference on Language Resources and Evaluation (LREC)(2022)
摘要
The scarcity of parallel data is a major limitation for Neural Machine Translation (NMT) systems, in particular for translation into morphologically rich languages (MRLs). An important way to overcome the lack of parallel data is to leverage target monolingual data, which is typically more abundant and easier to collect. We evaluate a number of techniques to achieve this, ranging from back-translation to random token masking, on the challenging task of translating English into four typologically diverse MRLs, under low-resource settings. Additionally, we introduce Inflection Pre-Training (or PT-Inflect), a novel pre-training objective whereby the NMT system is pre-trained on the task of re-inflecting lemmatized target sentences before being trained on standard source-to-target language translation. We conduct our evaluation on four typologically diverse target MRLs, and find that PT-Inflect surpasses NMT systems trained only on parallel data. While PT-Inflect is outperformed by back-translation overall, combining the two techniques leads to gains in some of the evaluated language pairs.
更多查看译文
关键词
low resource nmt, morphology, inflection
AI 理解论文
溯源树
样例
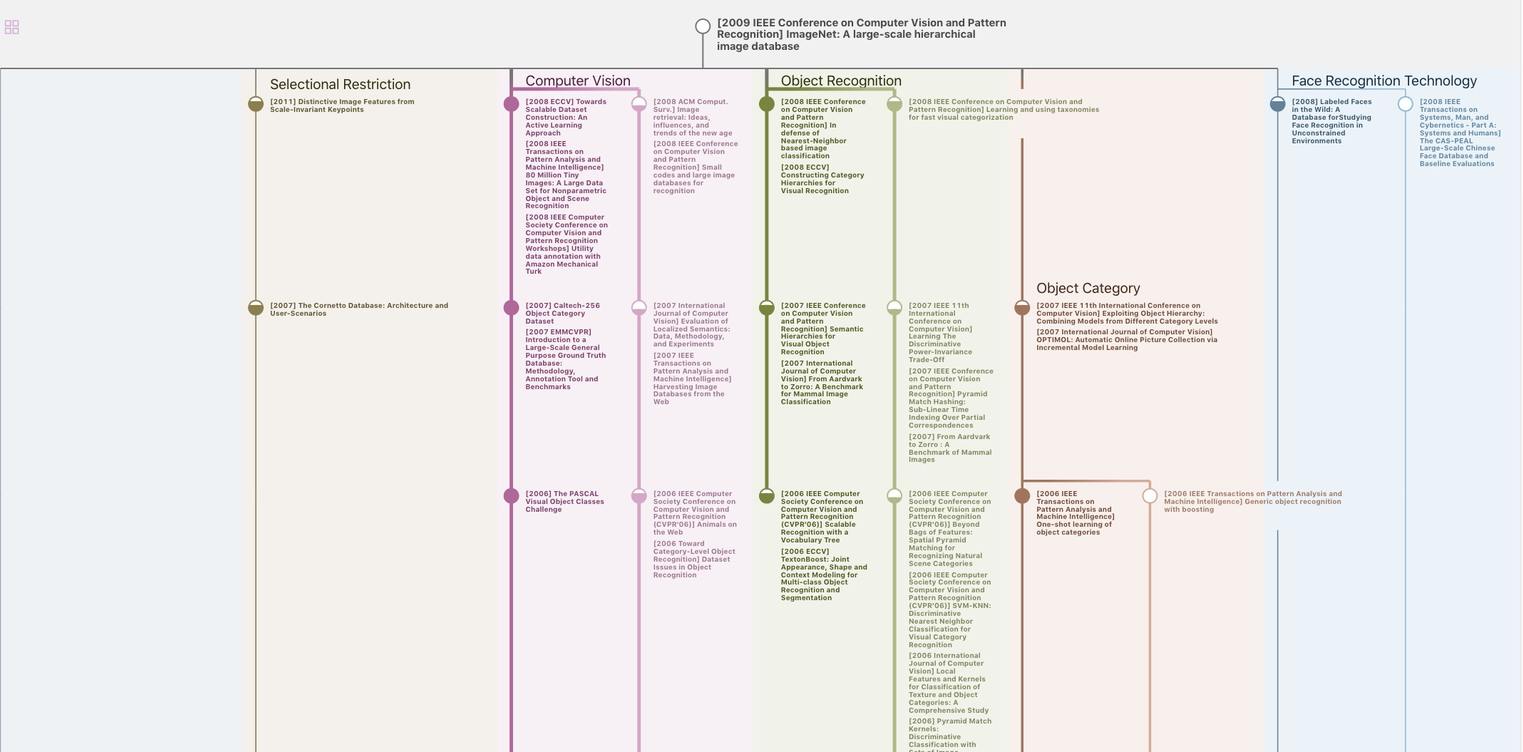
生成溯源树,研究论文发展脉络
Chat Paper
正在生成论文摘要