Data-Age Analysis for Multi-Rate Task Chains under Timing Uncertainty.
International Conference on Real-Time and Network Systems (RTNS)(2022)
摘要
Safety and control functions of modern automotive systems are implemented as chains of periodic data producer/consumer tasks run at different rates. To simplify the development of such systems, automotive standards relax synchronization requirements between tasks, allowing a task to run even if its dependent tasks are inactive. This gives birth to more complex timing constraints such as data age, which specifies the maximum duration of time that input data of a task chain is still allowed to affect the output of the chain. We develop a technique to compute lower and upper bounds on the data age of multi-rate task chains that execute upon a heterogeneous computing platform using a job-level fixed-priority scheduling policy. To the best of our knowledge, we are the first to consider uncertainties in the timing parameters (namely, both the release jitter and execution-time variation) of the tasks. Such an assumption makes the problem more challenging as it increases the number of possible schedules that the system may encounter during its lifespan. We represent these uncertainties by timing intervals and devise an analysis that uses those intervals to explore possible dependencies between jobs. We incorporated various pruning rules to make the analysis much faster and far less pessimistic than the state of the art. Our evaluations on an industrial case study as well as synthetic task sets show that our analysis reduces the overestimation of the data age by 36% on average (and up to 42%) in comparison to the state of the art when the number of tasks varies from 10 to 50.
更多查看译文
AI 理解论文
溯源树
样例
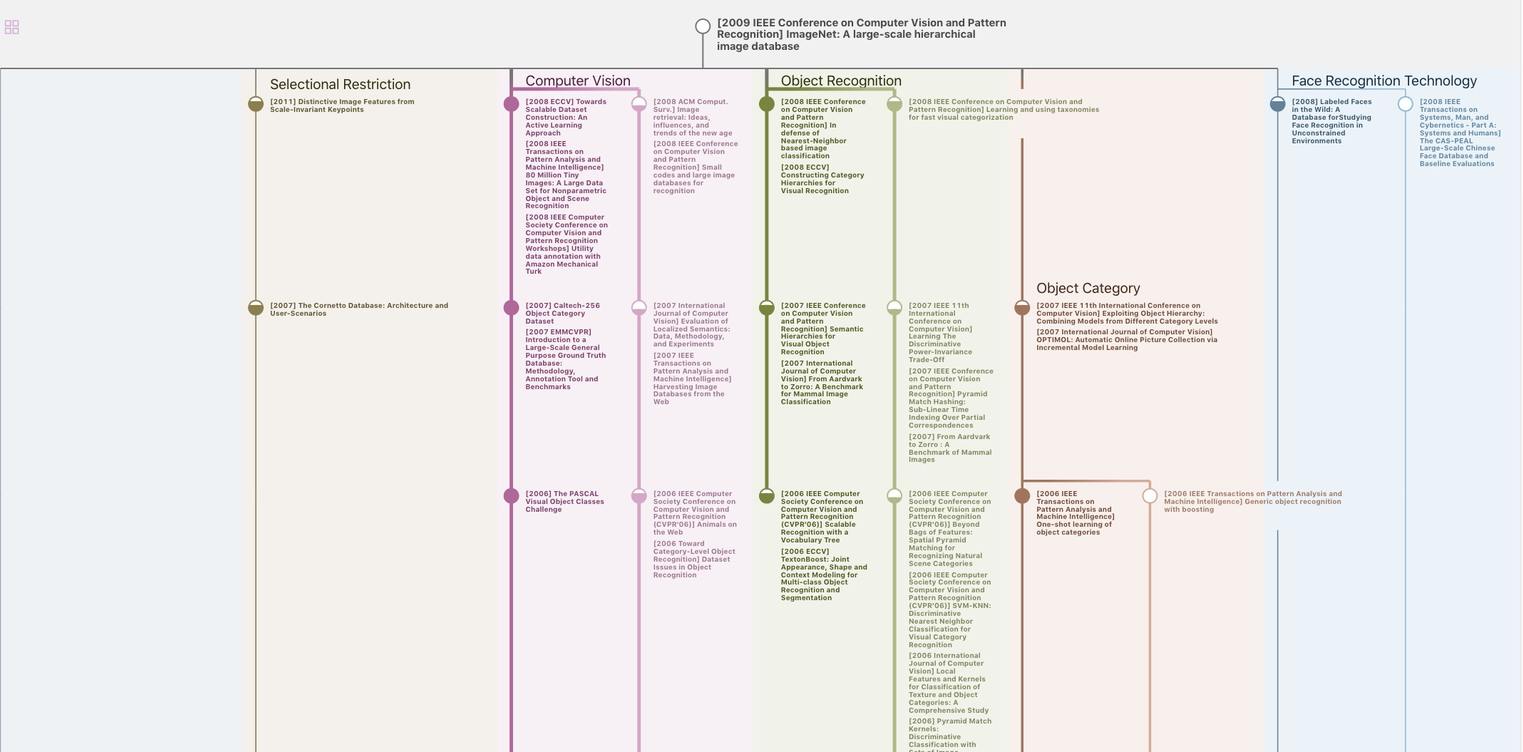
生成溯源树,研究论文发展脉络
Chat Paper
正在生成论文摘要