Real-Time Human Falling Recognition via Spatial and Temporal Self-Attention Augmented Graph Convolutional Network.
International Conference on Real-time Computing and Robotics (RCAR)(2022)
摘要
Currently, the skeleton-based human action recognition (e.g. walking, sitting and falling down) has achieved great interest, because the skeleton graph is robust to complex background and illumination changes compared to images. In this paper, a complete solution to real-time falling recognition task for intelligent monitoring has been provided. First, a manually annotated skeleton dataset for falling down action recognition is published. Then, a real-time self-attention augmented graph convolutional network (ST-SAGCN) is proposed. The network contains two novel architectures: a spatial self-attention module and a temporal self-attention module, which can effectively learn intra-frame correlations between different body parts, and inter-frame correlations between different frames for each joint. Finally, extensive comparative experiments on the dataset have proven that the proposed model can achieve remarkable improvement on falling recognition task. When the model is deployed in intelligent monitoring system, it achieves an inference speed over 40 fps and meets the demand of practical applications.
更多查看译文
关键词
manually annotated skeleton dataset,real-time self-attention augmented graph convolutional network,temporal self-attention module,intra-frame correlations,inter-frame correlations,intelligent monitoring system,skeleton-based human action recognition,skeleton graph,real-time human falling recognition task,ST-SAGCN,spatial self-attention module
AI 理解论文
溯源树
样例
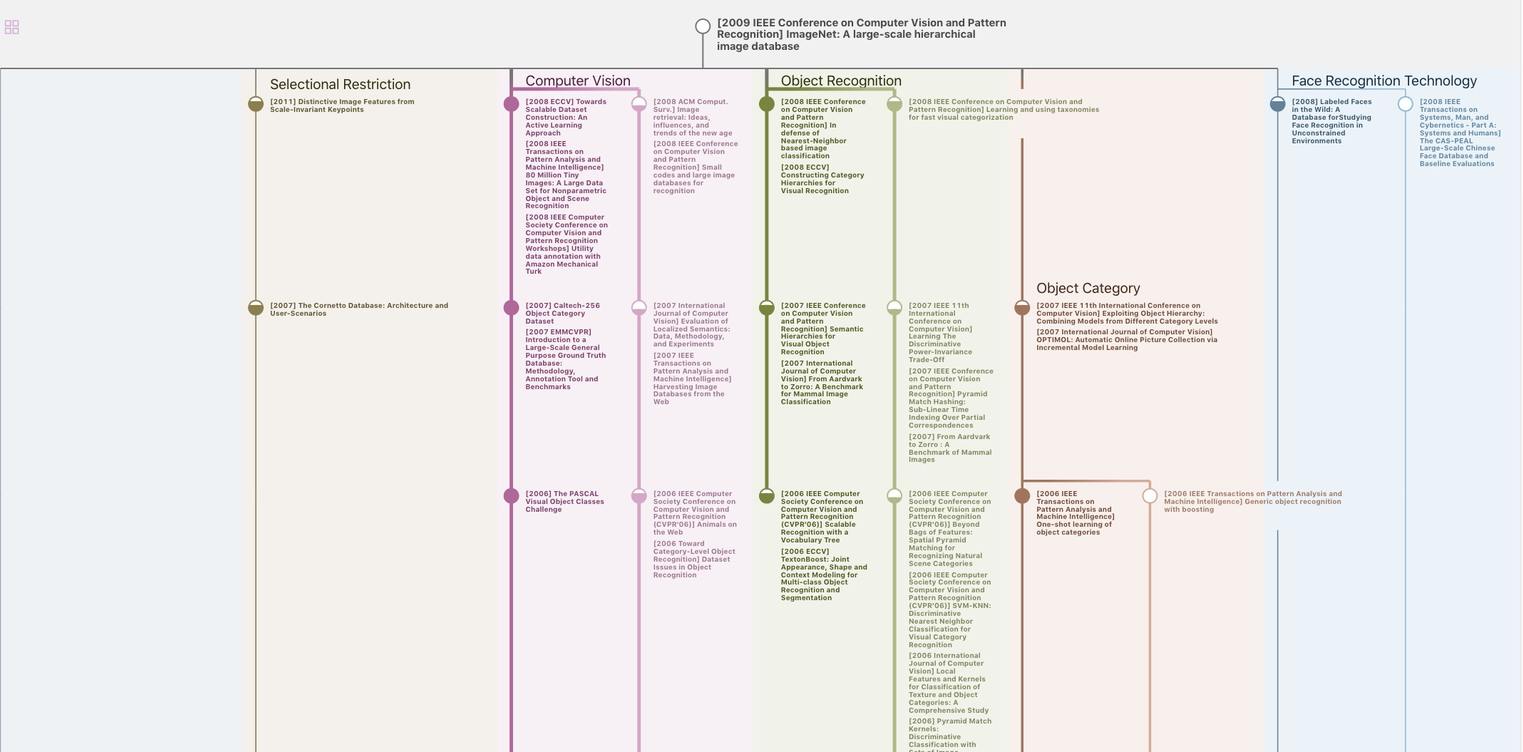
生成溯源树,研究论文发展脉络
Chat Paper
正在生成论文摘要