Learning Progression-based Automated Scoring of Visual Models.
International Conference on Pervasive Technologies Related to Assistive Environments (PETRA)(2022)
摘要
Visual models are defined as drawings created by students to illustrate their understanding of an observed scientific phenomenon. Along with the corresponding textual answers describing the phenomenon, these multimodal responses serve as rich vehicles of information for conducting assessment on students in their grasp of the underlying scientific concept. Though effective, manual scoring of these responses are both laborious and expensive. In our work, we apply a user interface tool for students to construct multimodal responses to scientific prompts, and propose an automated approach that relies on image processing to classify shapes in visual models, extract relevant features, and, ultimately, assign a learning progression score to each model. This effort is the first in a series of planned approaches targeted at utilizing multimodal evidence to evaluate, scaffold and guide students in their learning pathways in science education.
更多查看译文
AI 理解论文
溯源树
样例
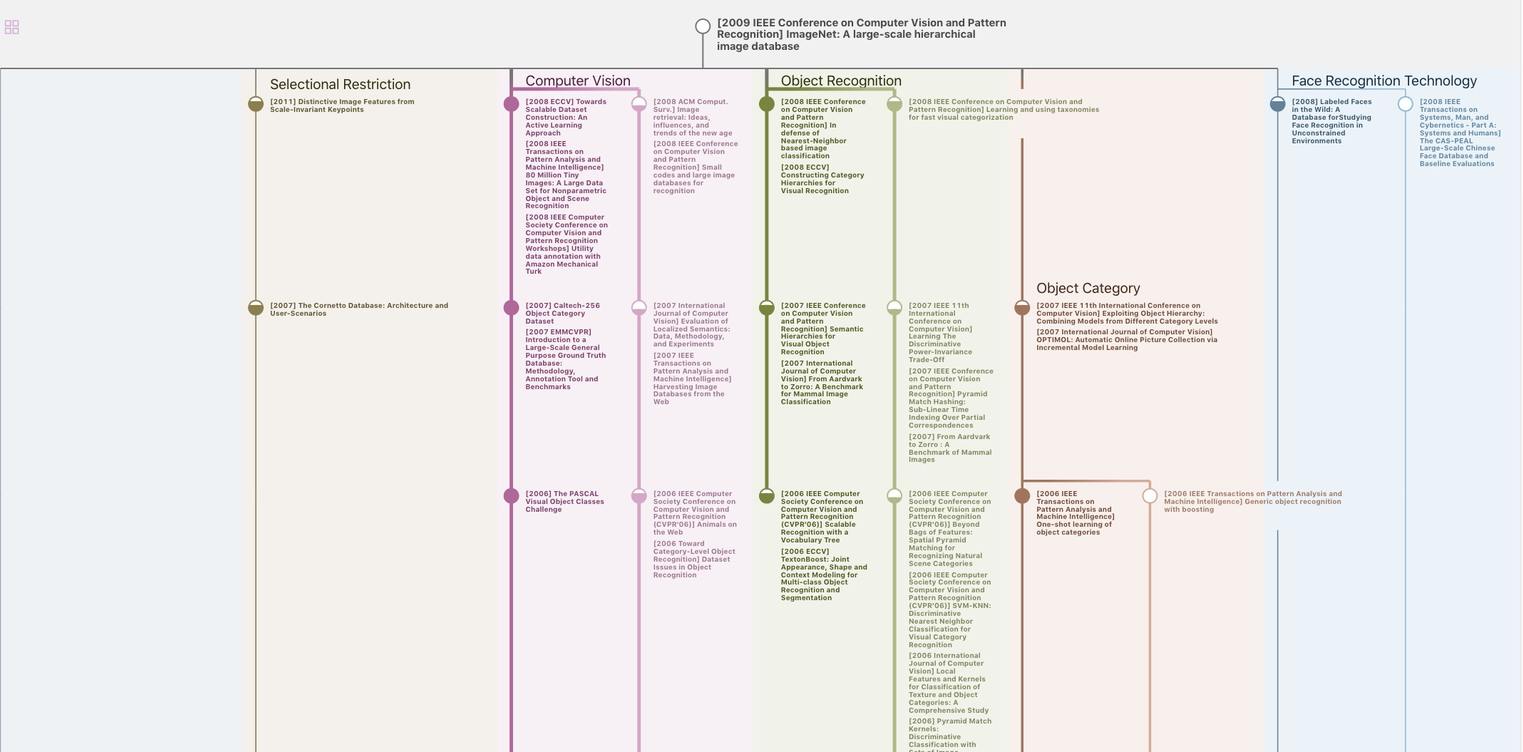
生成溯源树,研究论文发展脉络
Chat Paper
正在生成论文摘要