Fundamental Limits of Personalized Federated Linear Regression with Data Heterogeneity.
International Symposium on Information Theory (ISIT)(2022)
摘要
Federated learning is a nascent framework for collaborative machine learning over networks of devices with local data and local model updates. Data heterogeneity across the devices is one of the challenges confronting this emerging field. Personalization is a natural approach to simultaneously utilize information from the other users’ data and take data heterogeneity into account. In this work, we study the linear regression problem where the data across users are generated from different regression vectors. We present an information-theoretic lower bound of the minimax expected excess risk of personalized linear models. We show an upper bound that matches the lower bound within constant factors. The results characterize the effect of data heterogeneity on learning performance and the trade-off between sample size, problem difficulty, and distribution discrepancy, suggesting that the discrepancy-to-difficulty ratio is the key factor governing the effectiveness of heterogeneous data.
更多查看译文
关键词
collaborative machine learning,information theory,federated learning,personalized federated linear regression,personalized linear models,regression vectors,linear regression problem,data heterogeneity
AI 理解论文
溯源树
样例
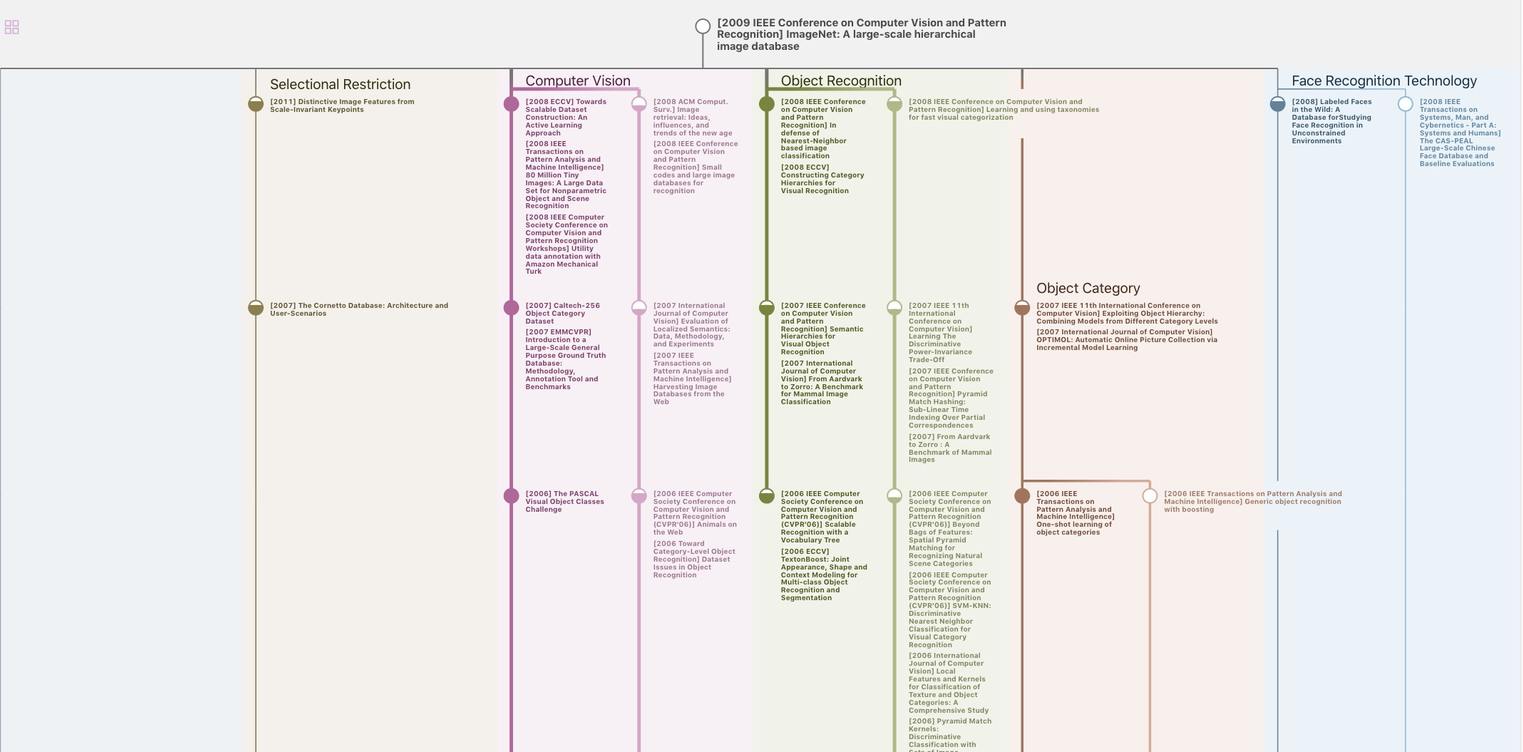
生成溯源树,研究论文发展脉络
Chat Paper
正在生成论文摘要