Differentially Private Distributed Matrix Multiplication: Fundamental Accuracy-Privacy Trade-Off Limits.
International Symposium on Information Theory (ISIT)(2022)
摘要
The classic BGW algorithm of Ben Or, Goldwasser and Wigderson for secure multiparty computing demonstrates that secure distributed matrix multiplication over finite fields is possible over 2t+1 computation nodes, while keeping the input matrices private from every t colluding computation nodes. In this paper, we develop and study a novel coding formulation to explore the trade-offs between computation accuracy and privacy in secure multiparty computing for real-valued data, even with fewer than 2t+1 nodes, through a differential privacy perspective. For the case of t = 1, we develop achievable schemes and converse arguments that bound ϵ — the differential privacy parameter that measures the privacy loss — for a given accuracy level. Our achievable coding schemes are specializations of Shamir secret sharing applied to real-valued data, coupled with appropriate choice of evaluation points. We develop converse arguments that apply for general additive noise based schemes.
更多查看译文
关键词
Differential privacy,privacy-utility tradeoff,mean square error,secure multiparty computation,coded computing,distributed matrix multiplication
AI 理解论文
溯源树
样例
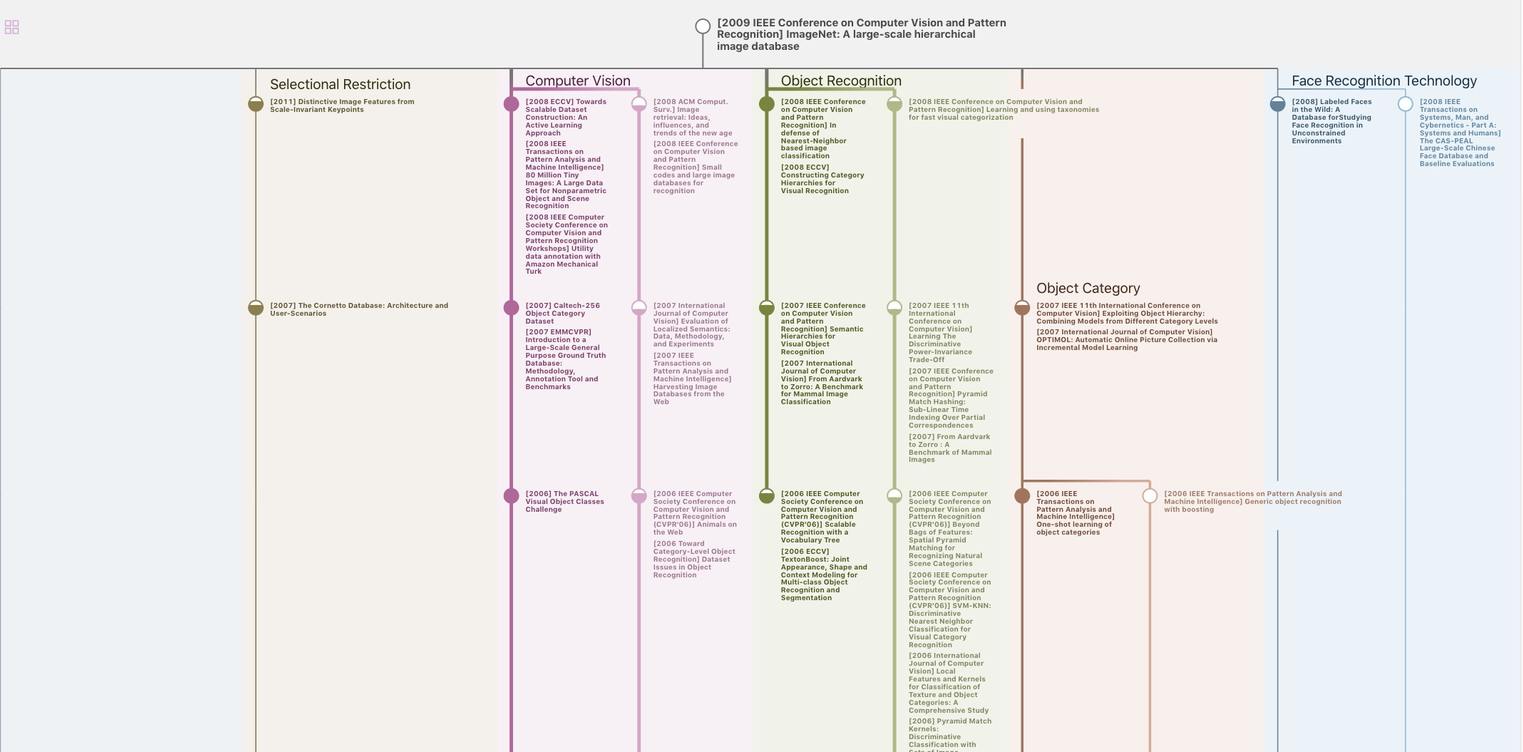
生成溯源树,研究论文发展脉络
Chat Paper
正在生成论文摘要