Fast Low Rank column-wise Compressive Sensing.
International Symposium on Information Theory (ISIT)(2022)
摘要
We study the “Low Rank column-wise Compressive Sensing (LRcCS)” problem: recover an n × q rank-r matrix, ${X^ * } = \left[ {x_1^ *,x_2^ *, \ldots x_q^ * } \right]$, with r ≪ min(n,q), from ${y_k}: = {A_k}x_k^ *,k \in [q]$, when y
k
is an m-length vector with m < n. The matrices A
k
are known and mutually independent for different k. Even though many other LR recovery problems have been extensively studied, this problem has received little attention. We introduce a novel gradient descent (GD) based solution called altGDmin, and show that, if all entries of all A
k
s are i.i.d. Gaussian, and if the right singular vectors of X
∗
satisfy the incoherence assumption, then ϵaccurate recovery of X
∗
is possible with mq > C(n+q)r
2
log(1/ϵ) total scalar samples and O(mqnrlog(1/ϵ)) time. Compared to existing work, to our best knowledge, this is the fastest solution and, for $\in < 1/\sqrt r$, it also has the best sample complexity.
更多查看译文
关键词
anm-length vector,LR recovery problems,rightsingular vectors,low rank column-wise compressivesensing,novelgradient descent based solution,altGDmin,incoherence assumption,ε-accurate recovery
AI 理解论文
溯源树
样例
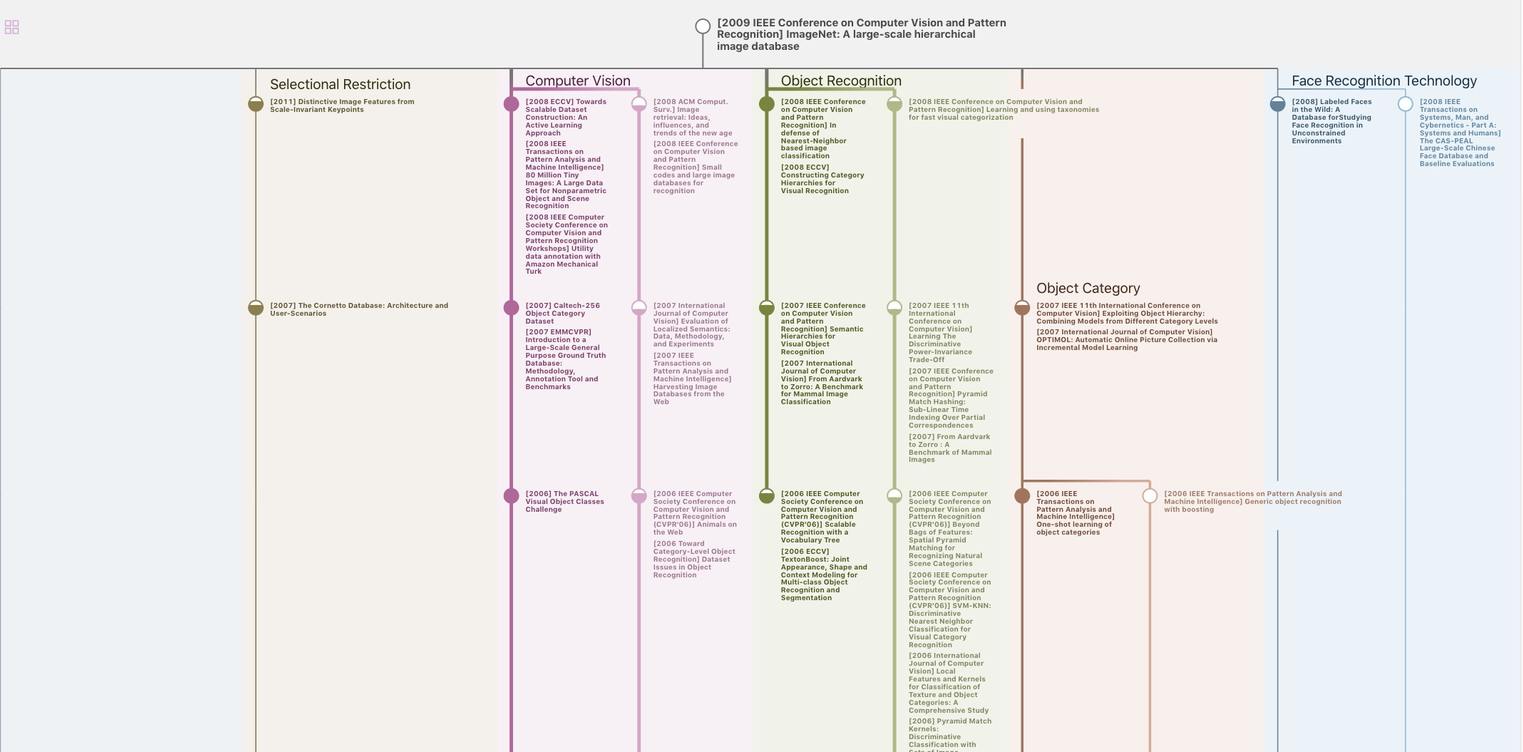
生成溯源树,研究论文发展脉络
Chat Paper
正在生成论文摘要