Rough Sets for Intelligence on Embedded Systems
International Syposium on Methodologies for Intelligent Systems (ISMIS)(2022)
摘要
In the continuing effort to run artificial intelligence (AI) on resource-constrained embedded devices, we present experiments that test the viability of enabling an embedded system to run Rough Set Theory. We first show that the Fuzzy-Rough Nearest Neighbor (FRNN) algorithm, a classic Rough Sets methodology, can indeed be effectively run on an embedded device. Next we compare 10 iterations of four algorithms in terms of power consumption, speed, accuracy, and model size. Specifically, we analyze on an embedded system, KNN, Fuzzy-Rough Nearest Neighbour (FRNN) without weights, FRNN with weights and Fuzzy Rough One-Versus-One (FROVOCO). Herein, we present a step towards the goal of designing an AI microprocessor, without software, based entirely on Rough Sets.
更多查看译文
关键词
Embedded systems,Rough Set Theory
AI 理解论文
溯源树
样例
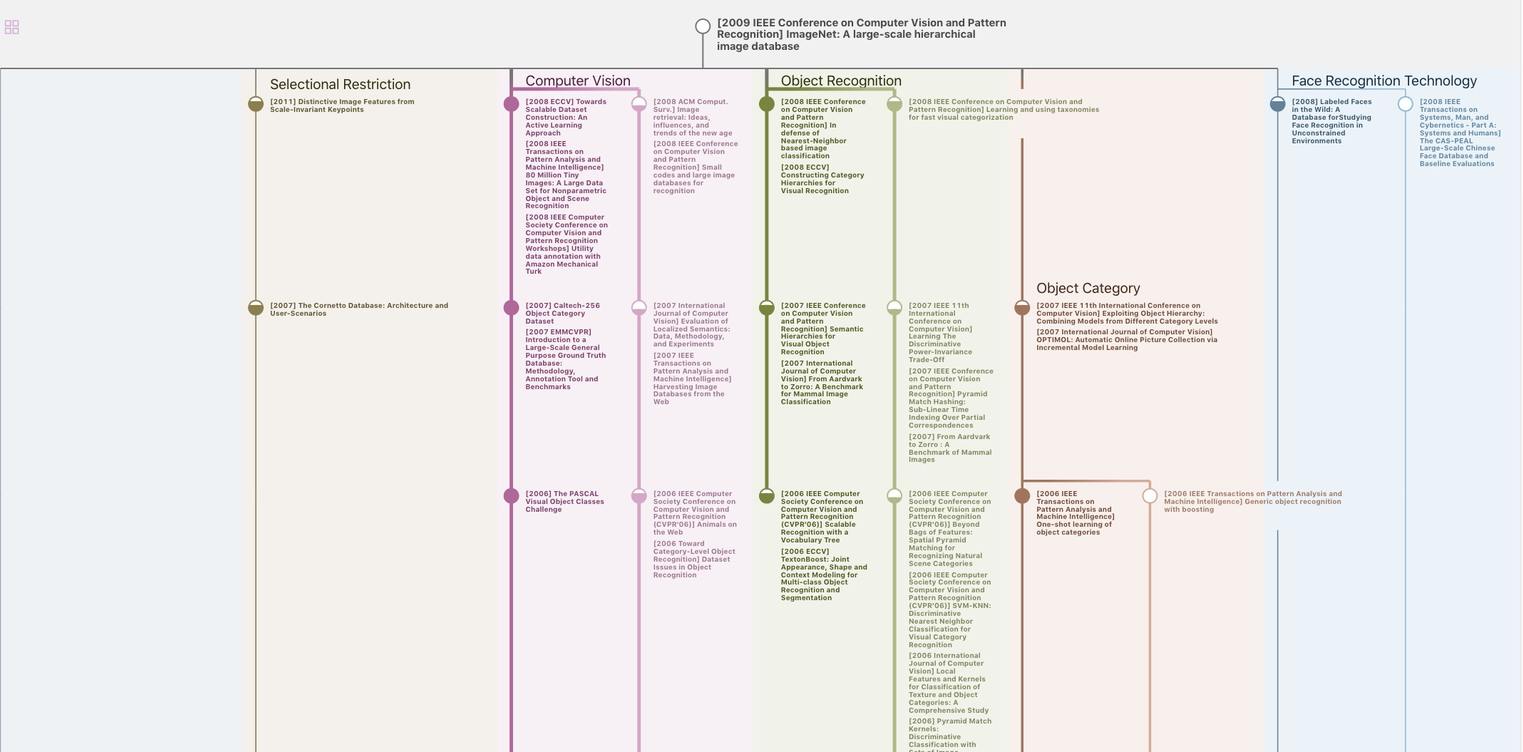
生成溯源树,研究论文发展脉络
Chat Paper
正在生成论文摘要