A Comparative Study: Classification Vs. Matrix Factorization for Therapeutics Recommendation
International Syposium on Methodologies for Intelligent Systems (ISMIS)(2022)
摘要
Hospital information systems (HIS) hold various healthcare information of patients. Most of them are held as structural data by a database table. This information include history of diagnoses, medications, applied procedures and laboratory results of patients which can be used by machine learning methods to predict some useful information about patients. These predictions can be the progress of a disease, which is called prognosis, or it can also be therapeutics which includes medications and procedures. In this paper, we explain how to recommend therapeutics using various machine learning approaches, especially by comparing classification methods with matrix factorization (a recommender systems approach). In order to evaluate the performance of compared methods, we applied experiments on real patients' electronic health records (EHR). We observed that matrix factorization outperforms the compared classification approaches in terms of accuracy. Therefore, it is feasible to employ matrix factorization in clinical decision support systems to provide therapeutics recommendations which improves the daily performance of physicians, so the life quality of the patients.
更多查看译文
关键词
Recommender systems,Matrix factorization,Multi-label classification,Healthcare
AI 理解论文
溯源树
样例
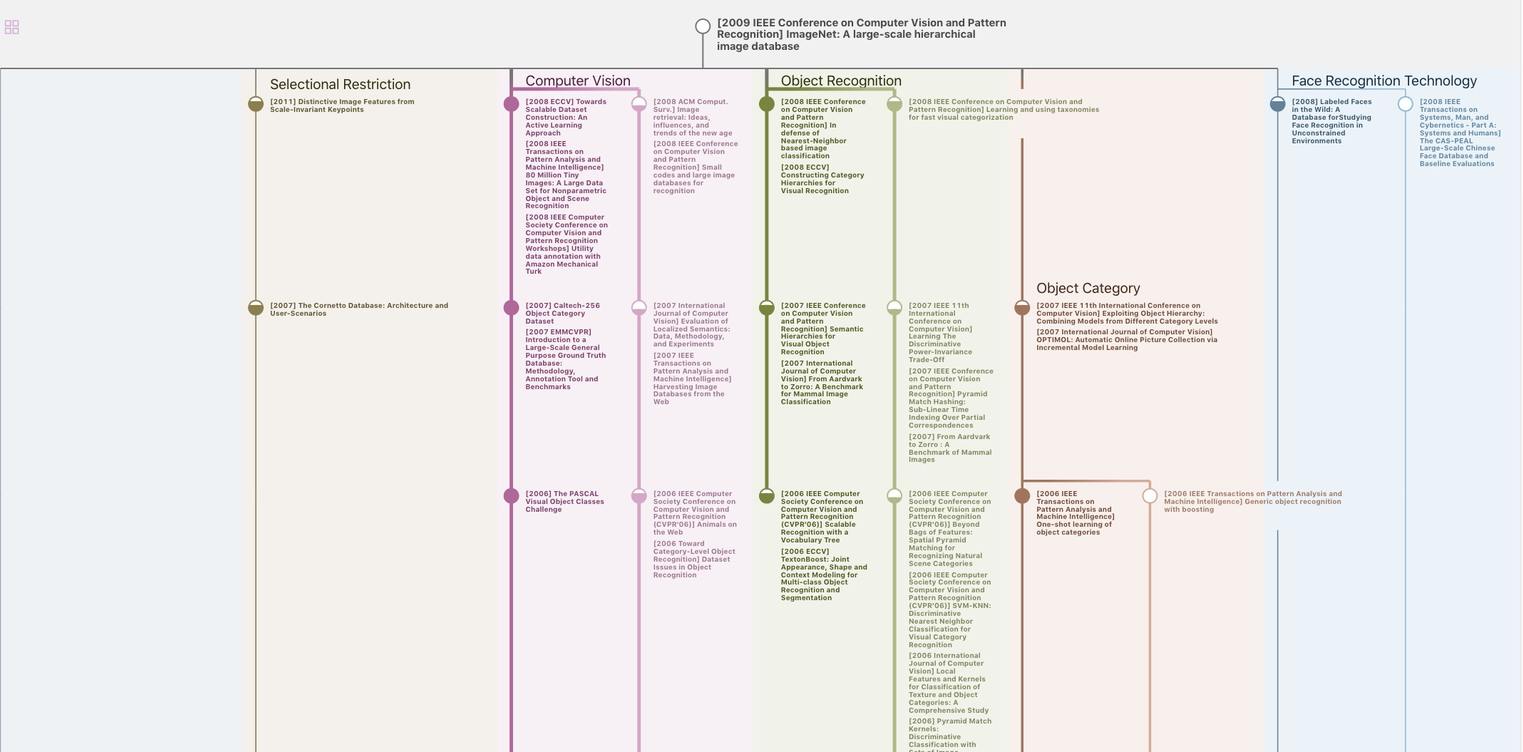
生成溯源树,研究论文发展脉络
Chat Paper
正在生成论文摘要