A Deep Learning Framework for the Prediction of Conversion to Alzheimer Disease
Bioinformatics and Biomedical Engineering(2022)
摘要
Alzheimer disease (AD) is the most common form of senile brain disorder. AD is not reversible, but its neuropathology can be detected several years before severe clinical manifestations. AD diagnosis is carried out relying on several clinical data, such as MRI structural and functional data, PET and DTI imaging, neuropsychological tests’ scores, genetic data, and others. Approaches that use complementary information and heterogeneous sources of data might have a decisive impact on the ability to early identify and consequently treat those subjects with a higher probability of conversion. We propose an on-going work on a Deep Learning framework that integrates different sources of data such as imaging data, clinical data, neuropsychological tests’ scores, and the temporal information related to the last medical evaluation of the subject, with the aim of estimating the probability of conversion from mild-cognitive-impairment (MCI) to AD or from a stable clinical profile to MCI in a period of time that varies from 6 months to 18 months. The possibility of predicting disease conversion is an open problem in this field, and wants to answer to a specific need of clinicians. The ADNI public data-set represents the reference data-set: an extensive and detailed analysis of ADNI has been performed to assess the sample size available for the training and testing of the network, that is now under construction, and the first results will be soon available. The network will also be tested with clinical data of the Fondazione Santa Lucia, Rome (Italy), and results will be discussed with the neurologists, neuropsychologists, and physics that are actively working with us.
更多查看译文
关键词
Alzheimer disease, Deep Learning, Prediction, Biomarkers identification
AI 理解论文
溯源树
样例
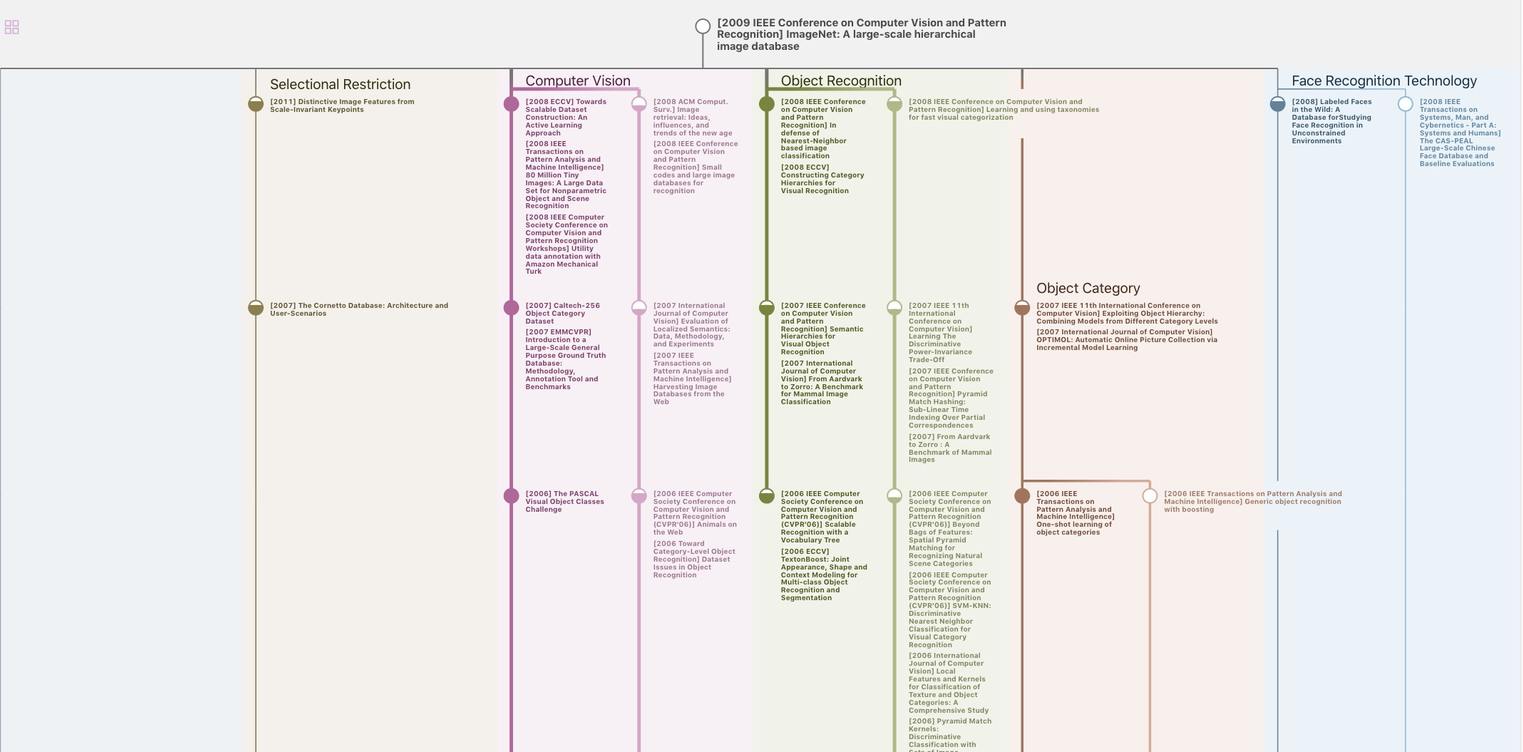
生成溯源树,研究论文发展脉络
Chat Paper
正在生成论文摘要