Measuring Convergence Inertia: Online Learning in Self-adaptive Systems with Context Shifts.
Leveraging Applications of Formal Methods (ISoLA)(2022)
摘要
To deal with situations not specifically designed for (unknown-unknowns), self-adaptive systems need to learn the best – or at least good enough – action to perform in each context faced during operation. An established solution for doing so is through the use of online learning. The complexity of online learning however increases in the presence of context shifts – which are typical in self-adaptive systems. In this paper, we (i) propose a new metric, convergence inertia , to assess the robustness of reinforcement learning policies against context shifts, and (ii) use it to assess the robustness of different policies within the family of multi-armed bandits (MAB) to context shifts. Through an experiment with a self-adaptation exemplar of a web server, we demonstrate that inertia and the accompanying interpretation of the unknown-unknowns problem is a viable way to inform the selection of online learning policies for self-adaptive systems, since it brings the influence of context shifts to the forefront. In our experiment, we found that non-stationary MAB policies are better suited to handling context shifts in terms of inertia, although stationary policies tend to perform well in terms of overall convergence.
更多查看译文
关键词
online learning,convergence inertia,self-adaptive
AI 理解论文
溯源树
样例
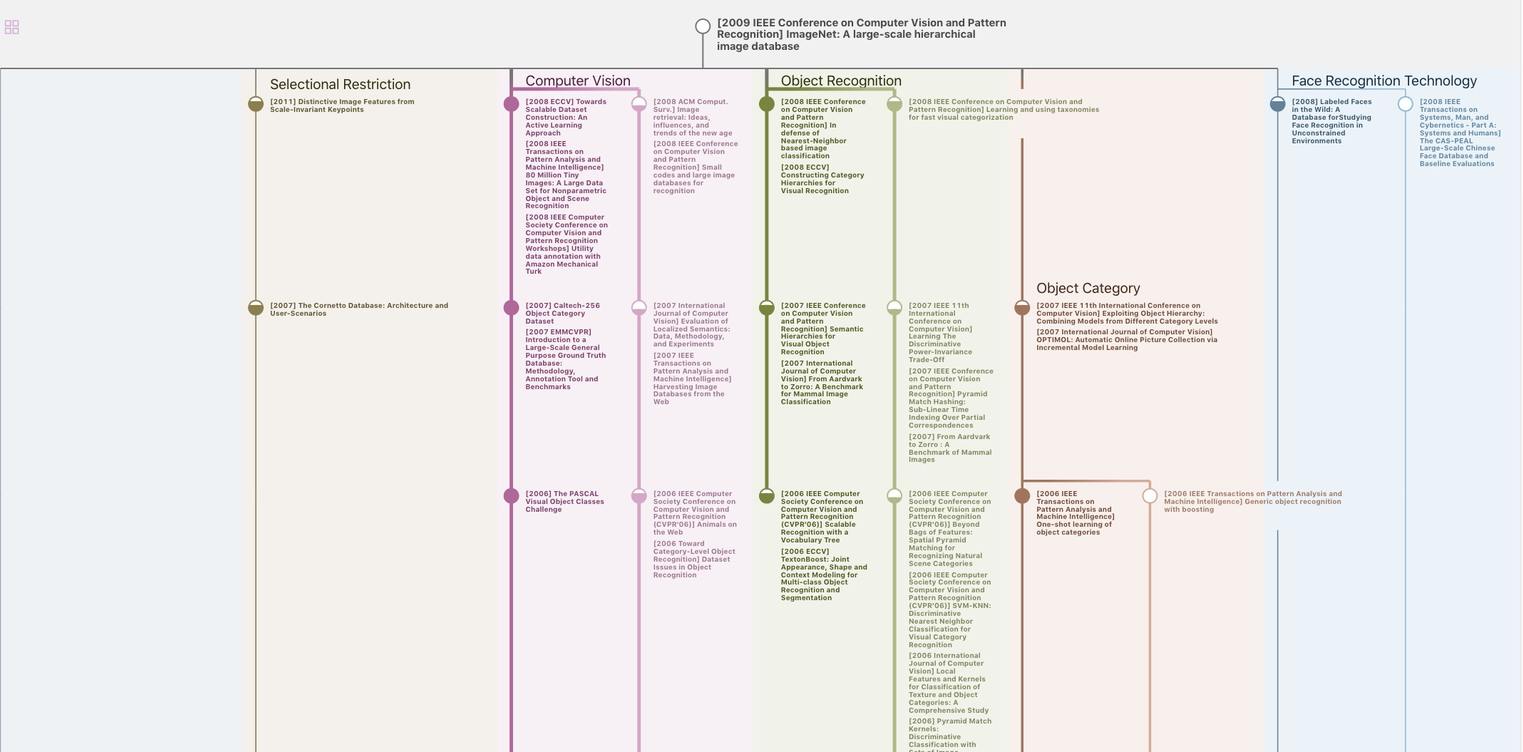
生成溯源树,研究论文发展脉络
Chat Paper
正在生成论文摘要