Label Enhancement Using Inter-example Correlation Information.
Pacific Rim International Conference on Artificial Intelligence (PRICAI)(2022)
摘要
Label distribution learning (LDL) to characterize the importance of different labels by label distribution has achieved good results in many application fields. LDL can learn more semantic information from the data than multi-label learning, however, most of the data in practical applications are single-label annotated or multi-label annotated, lacking the complete label distribution information suitable for label distribution learning. Thus, label enhancement (LE) is proposed to recover the label distributions from the logical labels. In this paper, we propose a new label enhancement method using inter-example correlation information that can automatically learn label correlations from data and jointly learn model and label correlations in a unified learning framework. Moreover, we also exploit the feature correlations constraining the model in the proposed method, which solves the problem that existing label enhancement algorithms cannot fully utilize the label information to improve the model performance. The experimental results on several real-world data sets validate the effectiveness of our method.
更多查看译文
关键词
Label enhancement,Label distribution learning,Label correlation,Feature correlation,Low rank
AI 理解论文
溯源树
样例
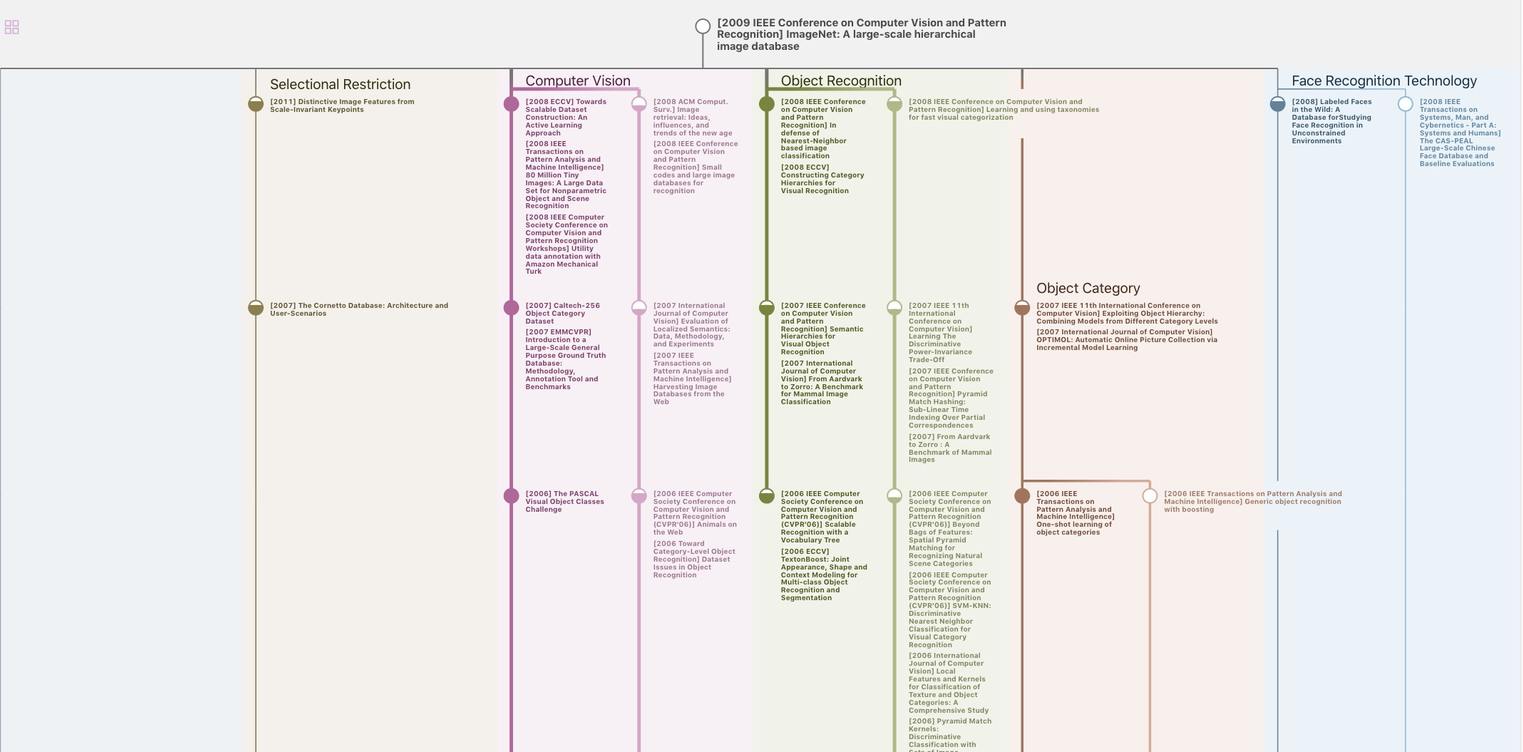
生成溯源树,研究论文发展脉络
Chat Paper
正在生成论文摘要