Reinforcement Learning Approach for Multi-period Inventory with Stochastic Demand.
Professional Practice in Artificial Intelligence (PPAI)(2022)
摘要
Finding an optimal solution to multi-period inventory ordering decision problems with uncertain demand is important for any manufacturing organization. Moreover, these problems are NP-hard as there are many factors to consider including customer demand and lead time which are stochastic in nature. This paper describes a reinforcement learning (RL) approach, Q-learning in particular, to decide on ordering policies. We formulated the finite horizon single-product multi-period problem into a reinforcement learning model in the form of Markov decision processes (MDP) and solve it to obtain the near-optimal solutions. Mixed integer linear programming (MILP) technique is still common in solving these problems; but they usually lack simplicity and may not optimized near to optimal. We formulated the same problem using the mixed integer linear programming model as the baseline algorithm so that we can compare it with RL approach. In comparison to MILP, the reinforcement learning agent performed better in making ordering decisions over the finite horizon. Obtaining better performance in multi-decisions and reduce the total inventory costs.
更多查看译文
关键词
Reinforcement learning, Multi-period inventory management, Q-learning
AI 理解论文
溯源树
样例
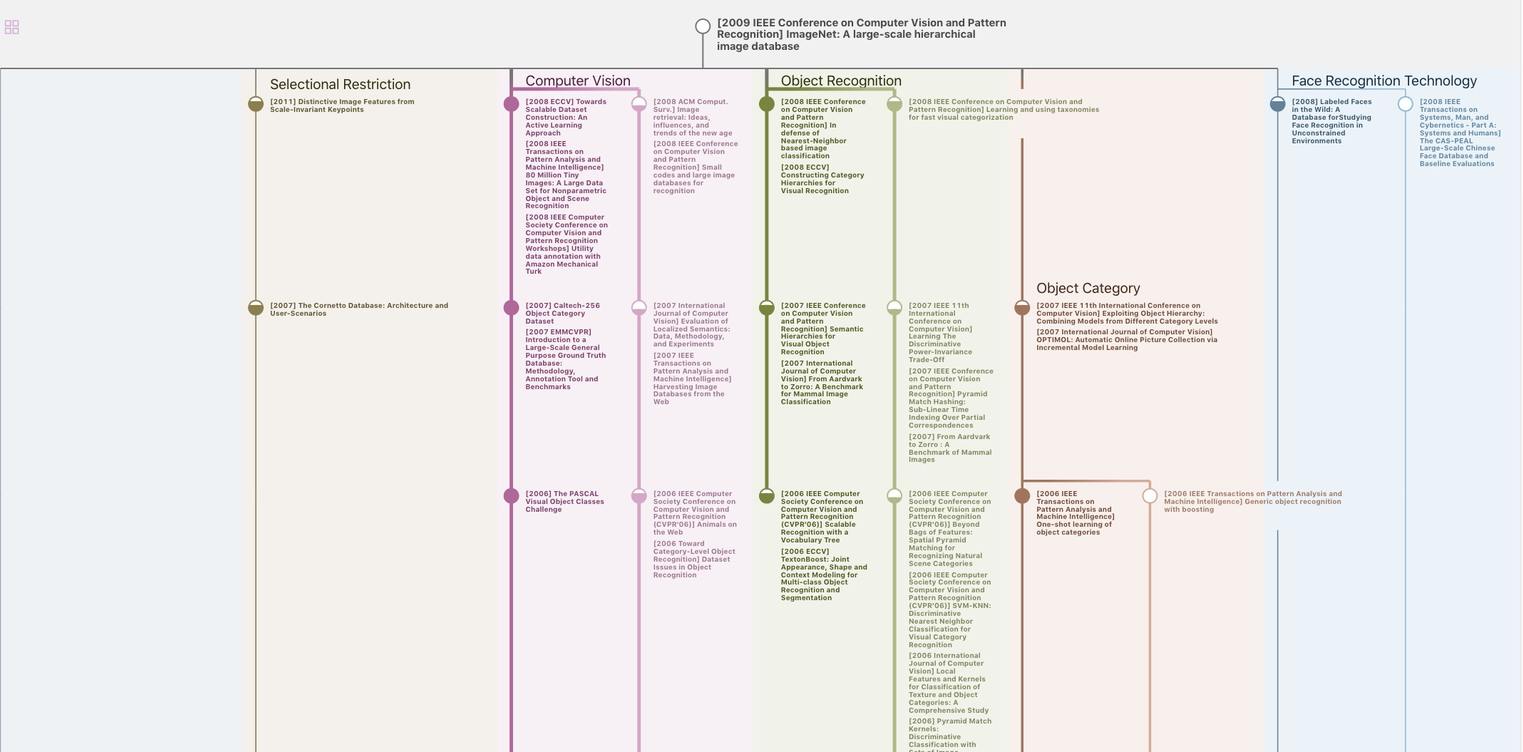
生成溯源树,研究论文发展脉络
Chat Paper
正在生成论文摘要