Distribution Guided Neural Disaggregation of PM10 and O-3 Hourly Concentrations from Daily Statistics and Low-Cost Sensors
Professional Practice in Artificial Intelligence (PPAI)(2022)
摘要
It is common for state-of-the-art research to demand higher granularity data to effectively model the atmospheric composition and personal exposure to air pollution. With the advent of Low-Cost Sensors (LCS) technology, the potential of increased spatiotemporal monitoring resolution arises, however, low cost comes with reduced measurement quality. On-site calibration via supervised machine learning (ML) is the most promising technique for the operational calibration of such devices. This study aims (a) to introduce the distribution guided neural disaggregation (DGND) method to increase the temporal resolution of air quality (AQ) low frequency data based on LCS high frequency readings and (b) simultaneously learn a calibration function with the ability to infer over the hourly resolution but with daily supervision. Towards this two-fold objective we propose an indirect training loss based on the first and second distribution moments errors to optimize a multi-layer perceptron (MLP). DGNDs generalization performance is compared against a traditionally trainedMLPwith the same architecture on a withheld test set in terms of errors and linearity. Furthermore, using the same metrics, the disaggregation results are evaluated on the original time series from which the reference moments originated. Results suggest that modeling the disaggregated (hourly) resolution of PM10 and O-3 concentrations is feasible from aggregated (daily) information indicated by modest to high linearity with coefficient of determination R-2 between 0.57-0.69 on the test set (except Sindos PM10 where R-2 < 0), and 0.49-0.83 on the original time series accompanied by moderate to low errors.
更多查看译文
关键词
distribution guided neural disaggregation,o3 hourly concentrations,sensors,pm10,low-cost
AI 理解论文
溯源树
样例
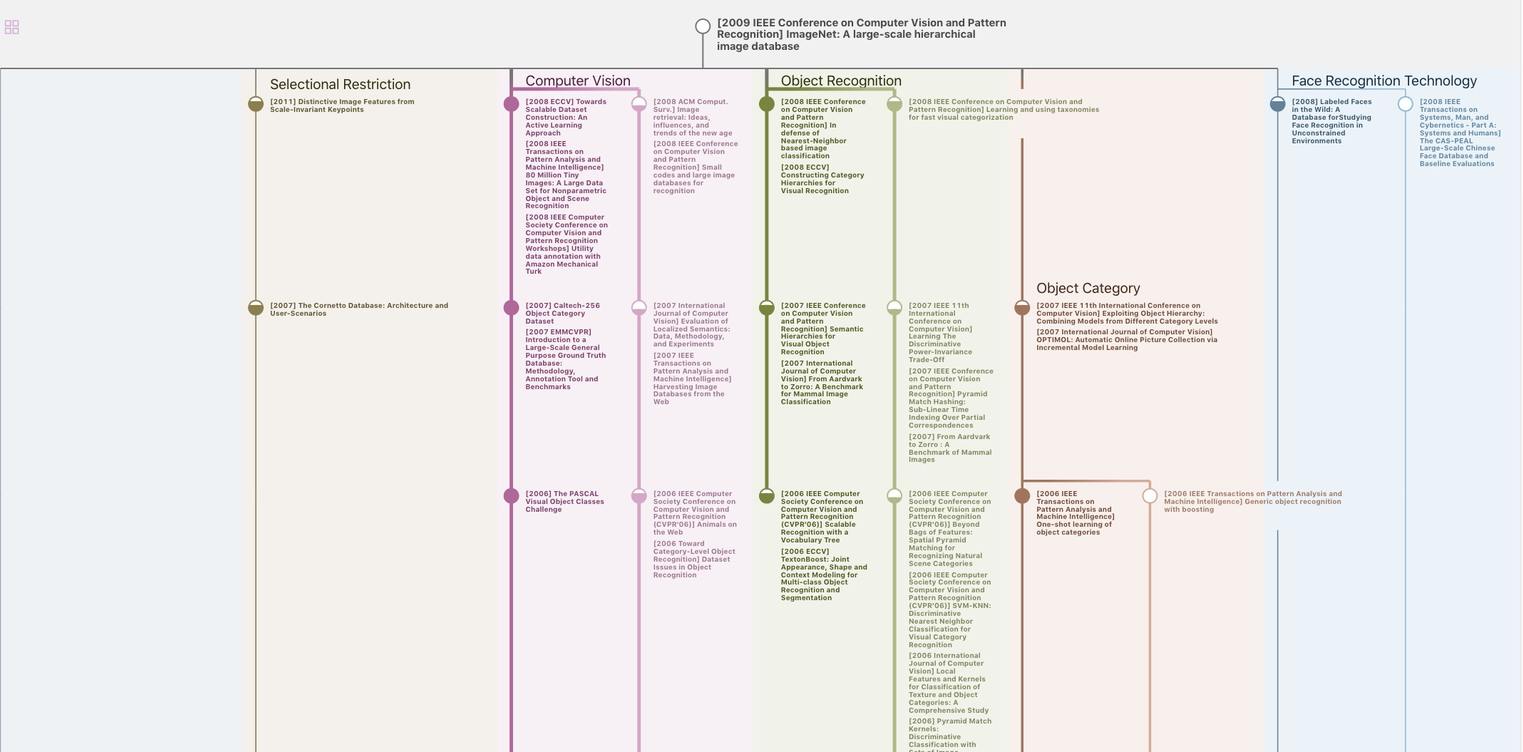
生成溯源树,研究论文发展脉络
Chat Paper
正在生成论文摘要