Unsupervised Adaptation of DNN for Brain-Computer Interface Spellers.
Signal Processing and Communications Applications Conference (SIU)(2022)
摘要
Brain-computer interface (BCI) spellers, based on the steady-state evoked potentials (SSVEP), significantly contribute to the communication of individuals with neuromuscular disorders. These systems aim to predict a target character that a user is intended to spell as fast as possible while maintaining high accuracy. Accordingly, target character identification methods aim to reach the high information transfer rate (ITR). Methods reaching high ITR values in the literature use participants’ labeled data for user calibration, which requires long and exhausting experiments for every individual that will use the speller. In this study, we developed a method that does not require labeled data from the new users; as the system is used it utilizes the accumulated unlabeled data effectively. Our method transfers the information obtained from previous users to the new user by training a deep neural network (DNN). Afterward, it uses accumulated unlabeled data of the new user to adapt the transferred DNN to that user. Adaptation is performed by assuming the DNN model’s predicted target labels on the data as correct. And the model is updated in every iteration by utilizing dropout layers. Our method is compared with online template transfer canonical correlation analysis (OTT-CCA) and adaptive combined transfer canonical correlation analysis (Adaptive-C3A) methods. The comparison is performed on two large publicly available datasets (benchmark and BETA) for signal lengths between 0.2 − 1.0 seconds (s). The results have shown that our method reached approximately 5% higher maximum ITR.
更多查看译文
关键词
Brain-Computer Interface,BCI,Steady State Evoked Potentials,SSVEP,Unsupervised Learning,Deep Neural Net-work,Dropout Layer,Transfer Learning
AI 理解论文
溯源树
样例
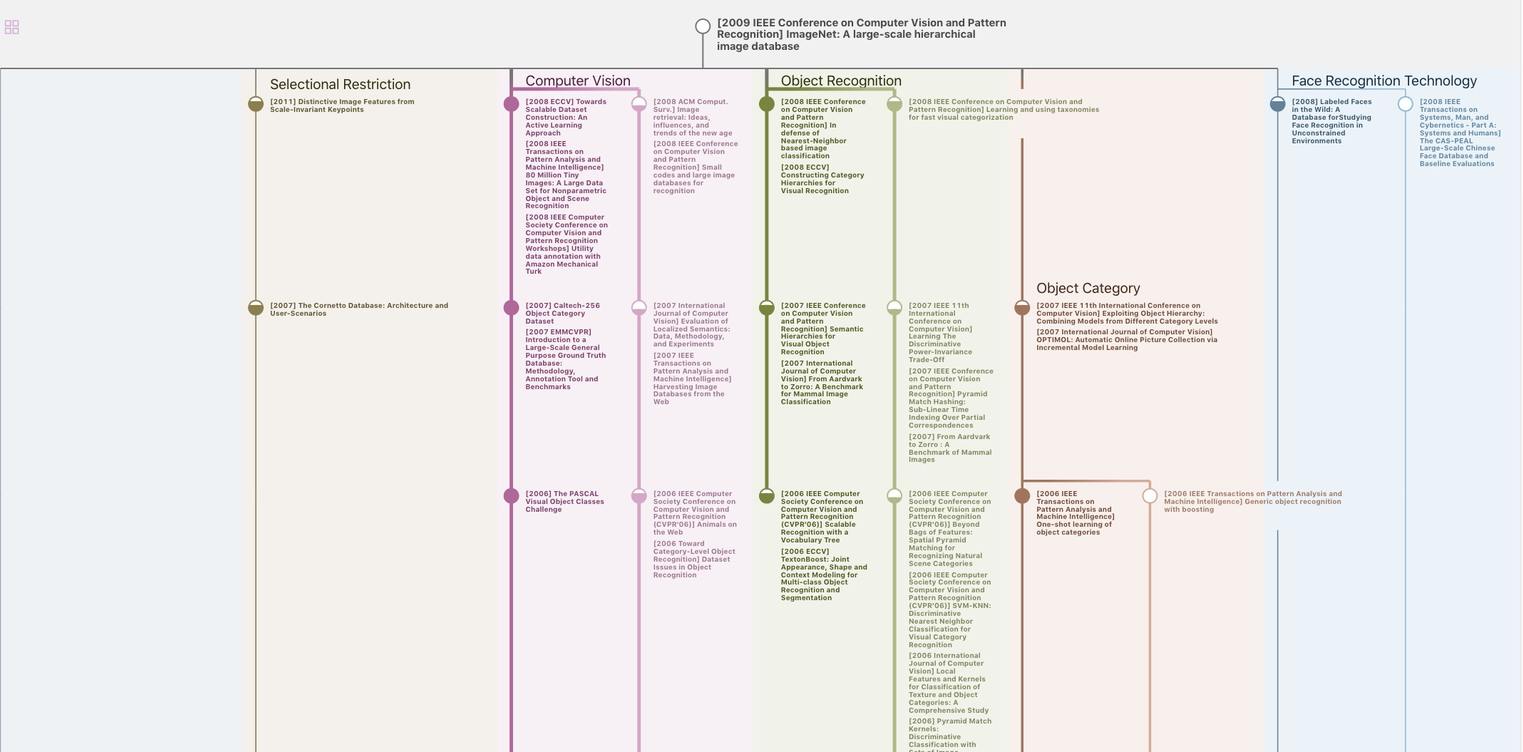
生成溯源树,研究论文发展脉络
Chat Paper
正在生成论文摘要