Quantitative Characterization of Ductility for Fractographic Analysis
Progress in Industrial Mathematics at ECMI 2021(2022)
摘要
We develop a machine-learning image segmentation pipeline that detects ductile (as opposed to brittle) fracture in fractography images. To demonstrate the validity of our approach, use is made of a set of fractography images representing fracture surfaces from cold-spray deposits. The coatings have been subjected to varying heat treatments in an effort to improve their mechanical properties. These treatments yield markedly different microstructures and result in a wide range of mechanical properties that combine brittle and ductile fracture once the materials undergo rupture. To detect regions of ductile fracture, we propose a simple machine learning network based on a 32-layers U-Net framework and trained on a set of small image patches. These regions most often contain small dimples and differ by the surface roughness. Overall, the machine-learning method shows good predictive capabilities when compared to segmentation by a human expert. Finally, we highlight other possible applications and improvements of the proposed method.
更多查看译文
关键词
fractographic analysis,ductility,quantitative characterization
AI 理解论文
溯源树
样例
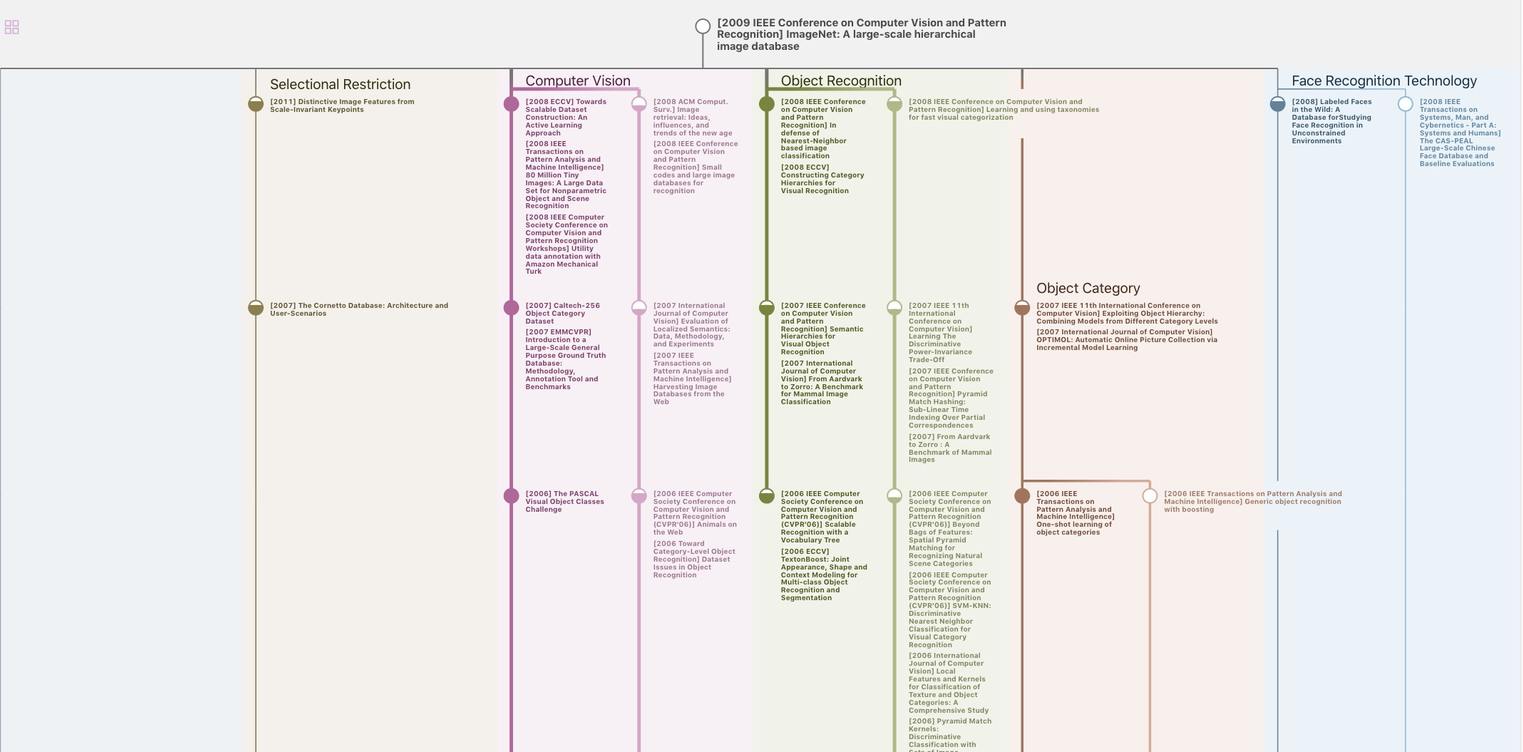
生成溯源树,研究论文发展脉络
Chat Paper
正在生成论文摘要