Online Weakly DR-Submodular Optimization with Stochastic Long-Term Constraints
Theory and Applications of Models of Computation(2023)
摘要
The online optimization has been extensively studied under a variety of different settings. In this paper, we consider the online maximization problems with stochastic linear cumulative constraints, where the objective functions are the sum of
$$\rho $$
-weakly DR-submodular functions and concave functions. Inspired by the penalty function strategy, we propose an algorithm of primal-dual type to solve this class of problems. Under mild conditions, we show that the algorithm achieves sublinear regret bounds and cumulative budget violation bounds with high probability.
更多查看译文
关键词
Online maximization, Weakly DR-submodular, Concave, Sublinear
AI 理解论文
溯源树
样例
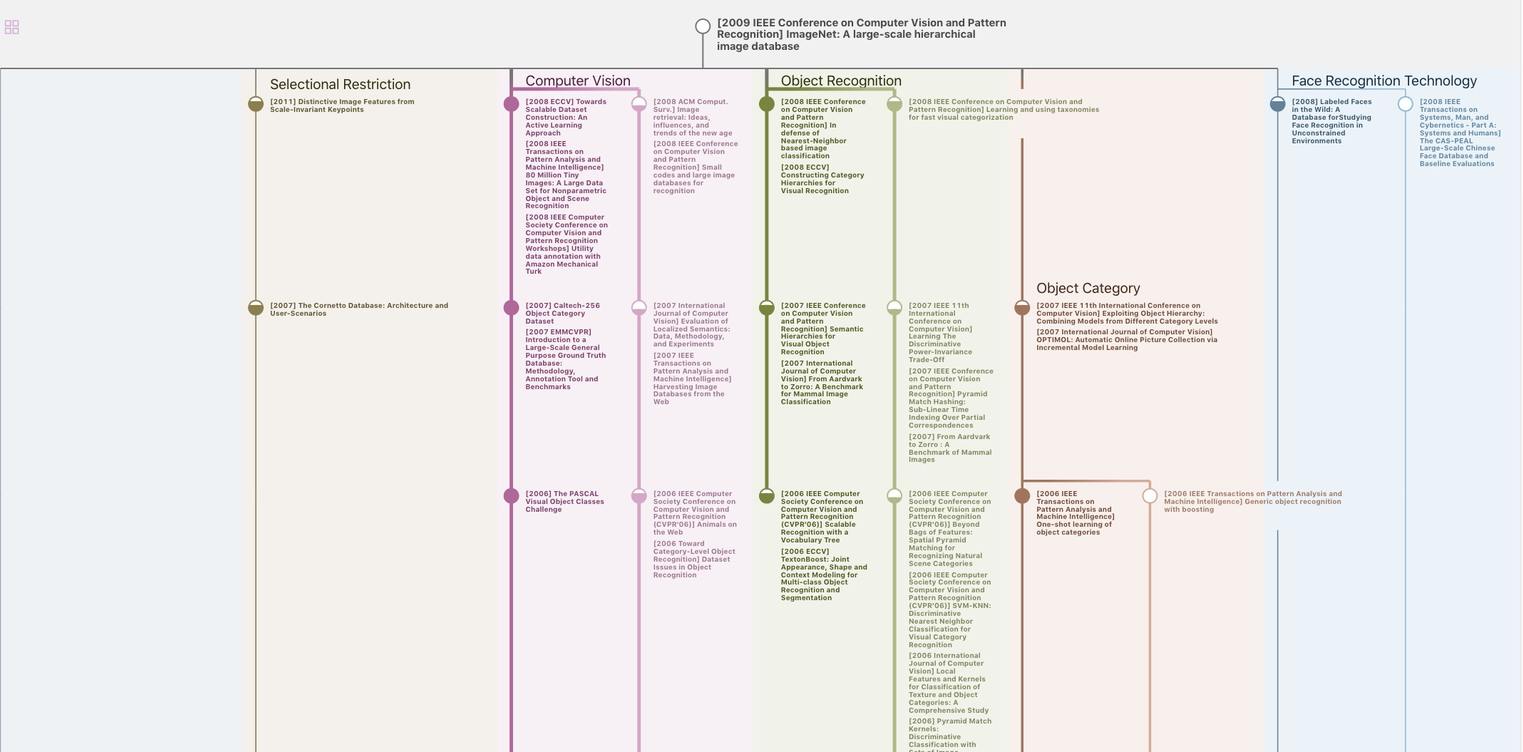
生成溯源树,研究论文发展脉络
Chat Paper
正在生成论文摘要