Phylogenetic Placement Problem: A Hyperbolic Embedding Approach
Comparative Genomics(2022)
Abstract
Phylogenetic trees define a metric space over their vertices, an observation that underlines distance-based phylogenetic inference. Several authors, including Layer and Rhodes (2017), have noted that we can embed leaves of a phylogenetic tree into high-dimensional Euclidean spaces in such a way that it minimizes the distortion of the tree distances. Jiang et al. (2021) use a deep learning approach to build a mapping from the space of sequences to the Euclidean space such that the mapped sequences accurately preserve the leaf distances on a given tree. Their tool, DEPP, uses this map to place a new query sequence onto the tree by first embedding it, an idea that was particularly promising for updating a species tree given data from a single gene despite the potential discordance of the gene tree and the species tree. In focusing on Euclidean spaces, these recent papers have ignored the strong theory that suggests hyperbolic spaces are more appropriate for embedding vertices of a tree. In this paper, we show that by moving to hyperbolic spaces and addressing challenges related to non-linearity and precision, we can reduce the distortion of distances for any given number of dimensions. The distortion of distances obtained using hyperbolic embeddings is lower than Euclidean embeddings with the same number of dimensions, both in training (backbone) and testing (query). The low-distortion distances of embeddings result in better topological accuracy in updating species trees using a single gene compared to its Euclidean counterpart. It also improves accuracy in placing queries for some datasets but not all.
MoreTranslated text
Key words
Phylogenetic placement, Deep learning, Hyperbolic spaces, Tree embedding, Distance-based phylogenetics
AI Read Science
Must-Reading Tree
Example
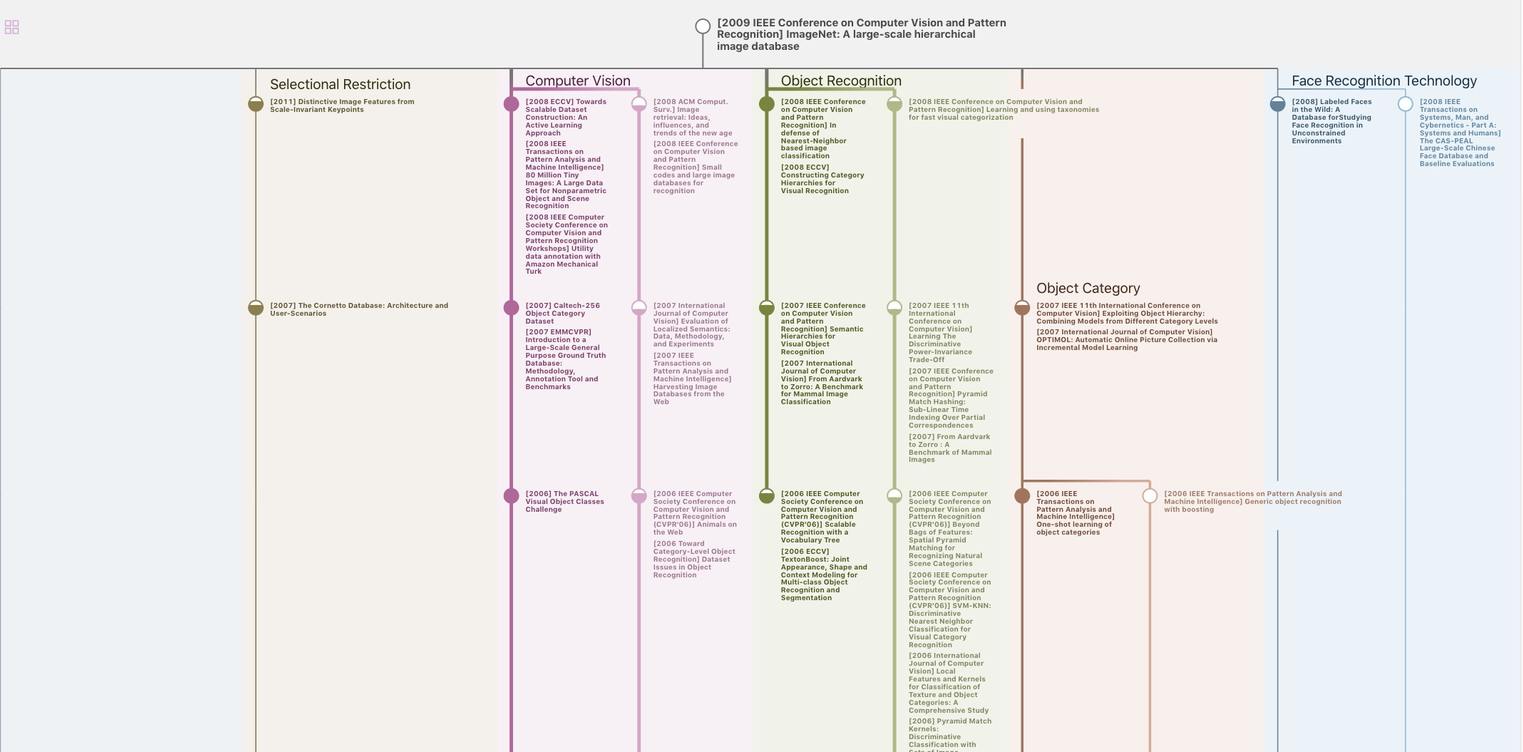
Generate MRT to find the research sequence of this paper
Chat Paper
Summary is being generated by the instructions you defined