ConveXplainer for Graph Neural Networks
Intelligent Systems(2022)
摘要
Graph neural networks (GNNs) have become the most prominent framework for representation learning on graph-structured data. Nonetheless, cue to its black-box nature, they often suffer from the same plague that afflicts many deep learning systems: lack of interpretability. To mitigate this issue, many recent approaches have been proposed to explain GNN predictions. In this paper, we propose a simple explanation method for graph neural networks. Drawing inspiration from recent works showing that GNNs can often be simplified without any impact on performance, we propose distilling GNNs into simpler (linear) models and explaining the latter instead. After distillation, we extract explanations by solving a convex optimization problem which identifies the most relevant nodes for a given node-level prediction. Experiments on synthetic and real-world benchmarks show that our method is competitive with, if not outperforms, state-of-the-art explainers for GNNs.
更多查看译文
关键词
Graph neural networks, Explainability, Model distillation
AI 理解论文
溯源树
样例
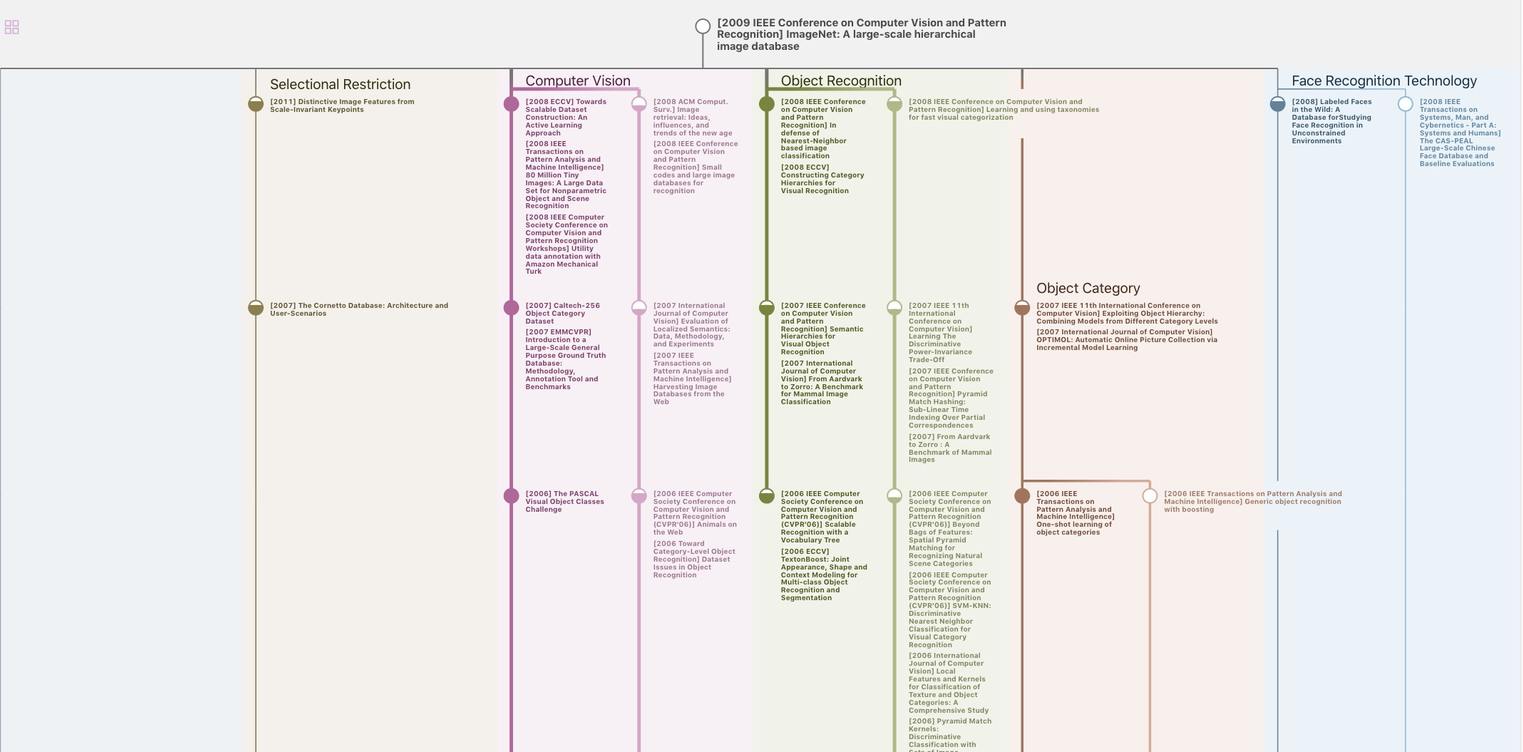
生成溯源树,研究论文发展脉络
Chat Paper
正在生成论文摘要