Multi-objective Meta-return Reinforcement Learning for Sequential Recommendation
Artificial Intelligence(2023)
摘要
With the demand for information filtering among big data, reinforcement learning (RL) that considers the long-term effects of sequential interactions is attracting much attention in the sequential recommendation realm. Many RL models have shown promising results on sequential recommendation; however, these methods have two major issues. First, they always apply the conventional exponential decaying summation for return calculation in the recommendation. Second, most of them are designed to optimize a single objective on the current reward or use simple scalar addition to combine heterogeneous rewards (e.g., Click Through Rate [CTR] or Browsing Depth [BD]) in the recommendation. In real-world recommender systems, we often need to simultaneously maximize multiple objectives (e.g., both CTR and BD), for which some objectives are prone to long-term effect (i.e., BD) and others focus on current effect (i.e., CTR), leading to trade-offs during optimization. To address these challenges, we propose a Multi-Objective Meta-return Reinforcement Learning (M
$$^2$$
OR-RL) framework for sequential recommendation, which consists of a meta-return network and a multi-objective gating network. Specifically, the meta-return network is designed to adaptively capture the return of each action in an objective, while the multi-objective gating network coordinates trade-offs among multiple objectives. Extensive experiments are conducted on an online e-commence recommendation dataset and two benchmark datasets and have shown the superior performance of our approach.
更多查看译文
关键词
sequential recommendation,reinforcement learning,multi-objective,meta-return
AI 理解论文
溯源树
样例
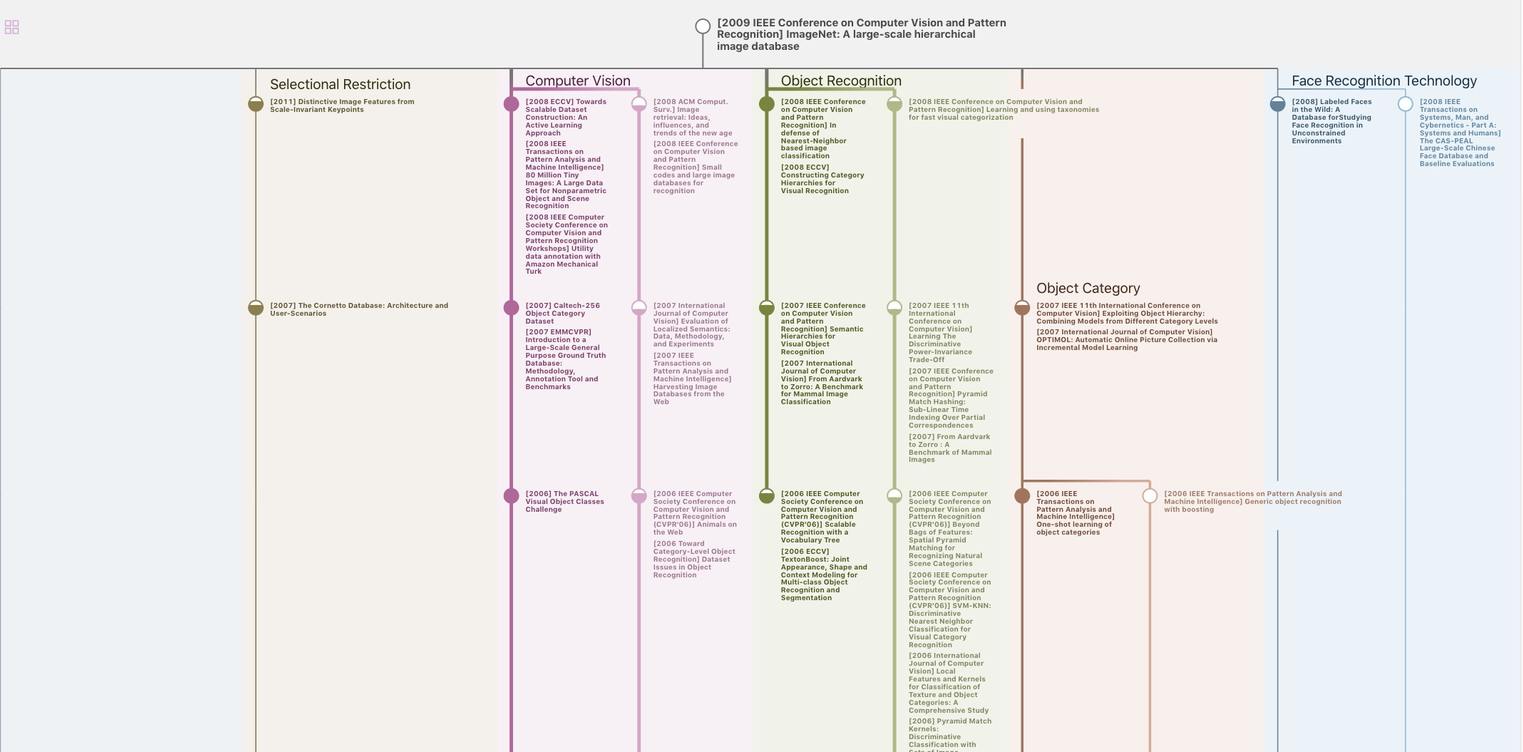
生成溯源树,研究论文发展脉络
Chat Paper
正在生成论文摘要