Clustering-based Curriculum Construction for Sample-Balanced Federated Learning
Artificial Intelligence(2022)
摘要
Federated learning is a distributed machine learning scheme that provides data privacy-preserving solution. A key challenge is data distribution heterogeneity of on different parties in federated learning. Existing methods only focus on the training rule of local model rather than data itself. In this paper, we reveal an fact that improving the performance of the local model can bring performance gain to the global model. Motivated by this finding, this paper proposes a Clustering-based curriculum construction method to rank the complexity of instances, and develops a Federation curriculum learning algorithm (FedAC). Specifically, FedAC assigns different weights to training samples of different complexity, which is able to take full advantage of the valuable learning knowledge from a noisy and uneven-quality data. Experiments were conducted on two datasets in terms of performance comparison, ablation studies, and case studies, and the results verified that FedAC can improve the performance of the state-of-the-art Federated learning methods.
更多查看译文
关键词
Curriculum learning, Federated learning, Neural networks
AI 理解论文
溯源树
样例
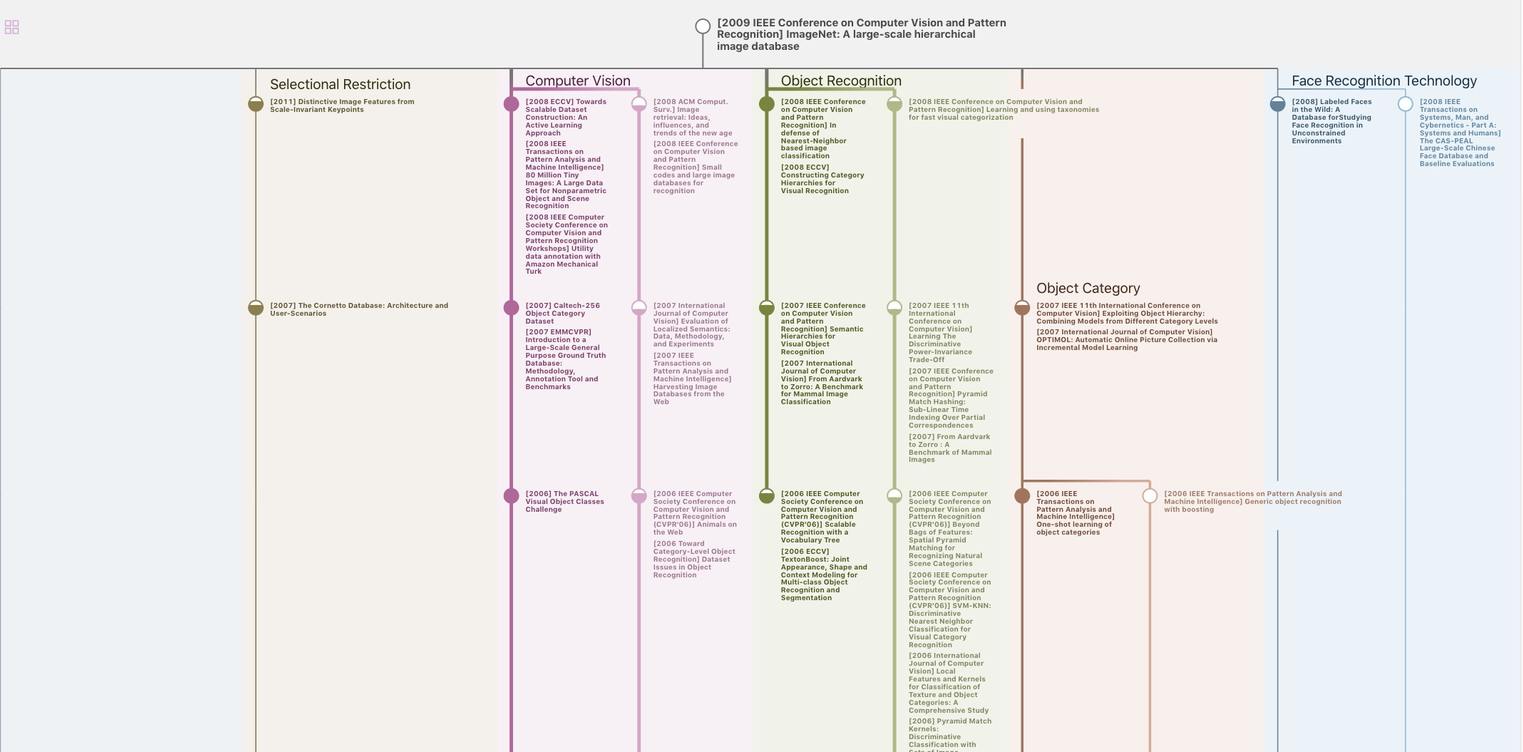
生成溯源树,研究论文发展脉络
Chat Paper
正在生成论文摘要