Research on Lower limb Movement Pattern Recognition Method Based on ReliefF-KPCA-SVM.
IEEE International Conference on Robotics and Biomimetics (ROBIO)(2021)
摘要
Faced with the complexity of traditional multi-sensor information fusion and the low recognition rate of lower limb motion pattern, a lower limb motion pattern recognition method based on ReliefF(feature weighting algorithms) and KPCA(kernel principle component analysis) combined with SVM(support vector machine) is proposed. Firstly, IMU and plantar pressure were selected as the information sources for gait pattern recognition, and the time domain and energy features (mean value, mean square error, extreme value, correlation coefficient and wavelet entropy) of the collected lower limb motion information were selected for ReliefF feature selection. Secondly, KPCA feature dimensionality reduction is performed on the selected features to remove the redundant information, and the eigenvalues can reflect the characteristics of motion mode are obtained. Finally, SVM was used to classify the feature vectors of lower limb motion patterns, and 10 movement patterns were identified, such as walking, upstairs, downstairs, uphill, downhill, jogging, cycling, squatting, sitting and standing. The recognition results of this paper are compared with BP neural network and PNN probabilistic neural network, which verifies the feasibility and practicability of this method.
更多查看译文
关键词
correlation coefficient,energy features,extreme value,feature vectors,gait pattern recognition,information sources,kernel principle component analysis,KPCA feature dimensionality reduction,lower limb motion information,lower limb motion pattern recognition method,lower limb movement pattern recognition method,mean square error,mean value,motion mode,movement patterns,redundant information,ReliefF feature selection,ReliefF-KPCA-SVM,time domain,wavelet entropy
AI 理解论文
溯源树
样例
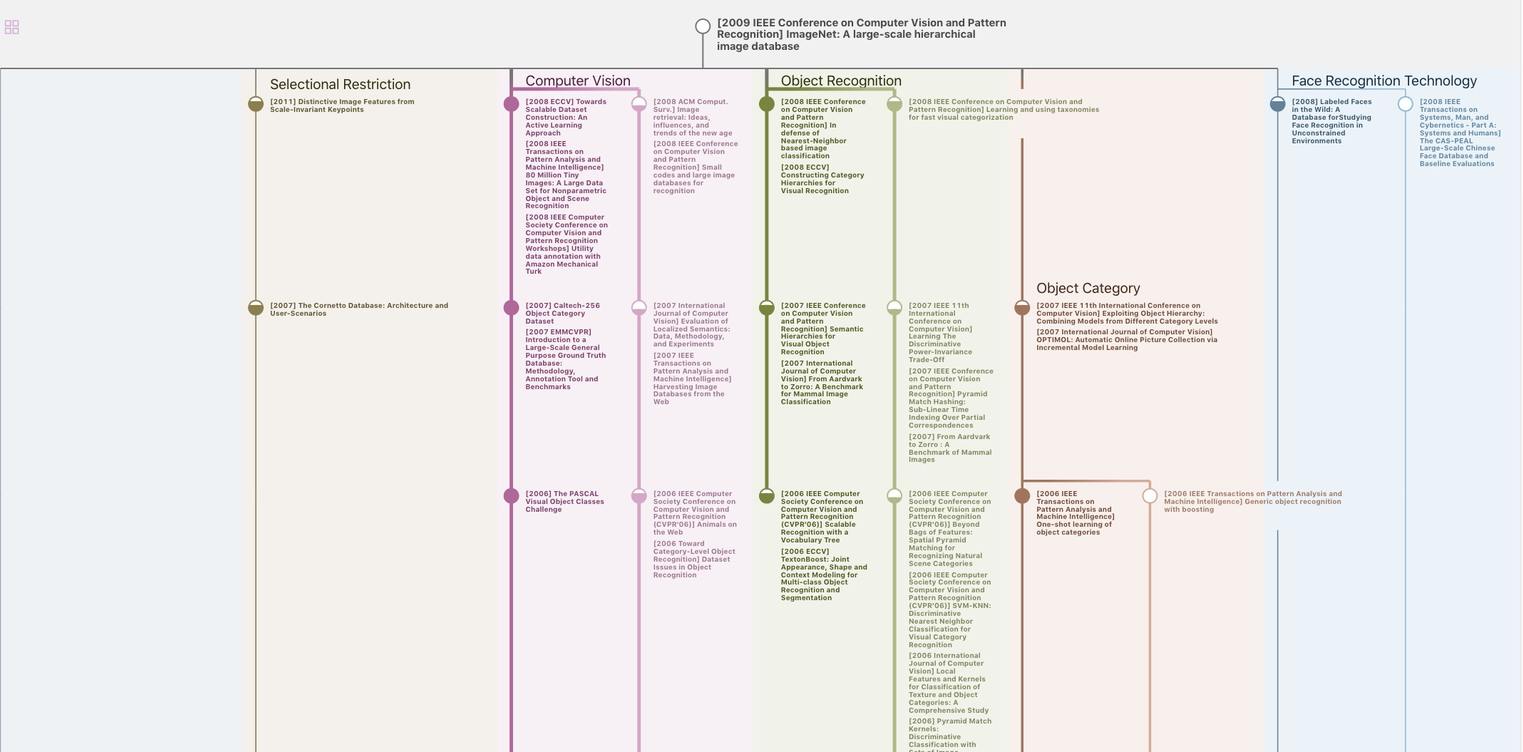
生成溯源树,研究论文发展脉络
Chat Paper
正在生成论文摘要