Predicting Headline Effectiveness in Online News Media using Transfer Learning with BERT.
International Conference on Deep Learning Theory and Applications (DeLTA)(2021)
摘要
The decision to read an article in online news media or social networks is often based on the headline, and thus writing effective headlines is an important but difficult task for the journalists and content creators. Even defining an effective headline is a challenge, since the objective is to avoid click-bait headlines and be sure that the article contents fulfill the expectations set by the headline. Once defined and measured, headline effectiveness can be used for content filtering or recommending articles with effective headlines. In this paper, a metric based on received clicks and reading time is proposed to classify news media content into four classes describing headline effectiveness. A deep neural network model using the Bidirectional Encoder Representations from Transformers (BERT) is employed to classify the headlines into the four classes, and its performance is compared to that of journalists. The proposed model achieves an accuracy of 59% on the four-class classification, and 72-78% on corresponding binary classification tasks. The model outperforms the journalists being almost twice as accurate on a random sample of headlines.
更多查看译文
关键词
BERT,Headline Effectiveness,Journalism,Machine Learning,Natural Language Processing
AI 理解论文
溯源树
样例
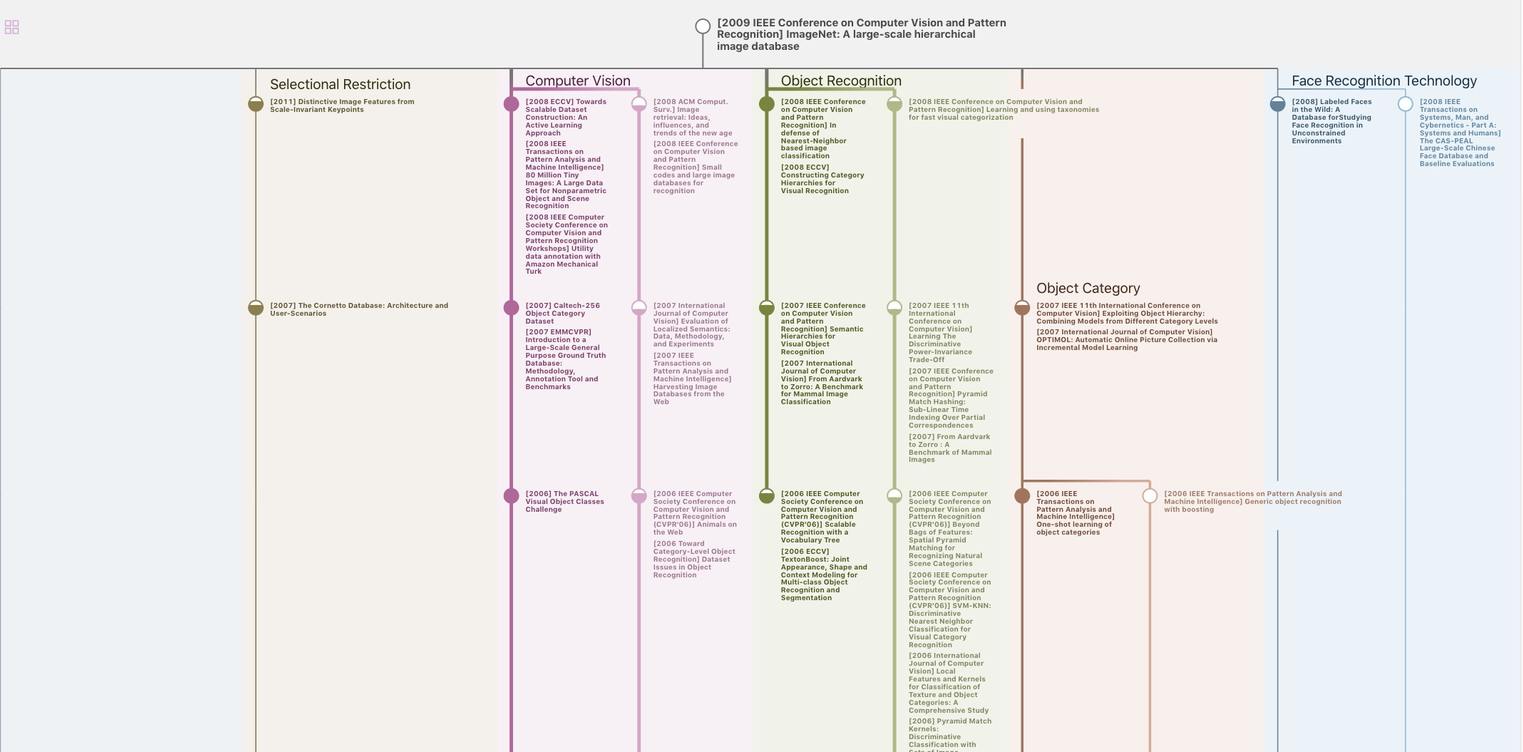
生成溯源树,研究论文发展脉络
Chat Paper
正在生成论文摘要