Detecting Adversarial Samples in Neural Network with Statistical Metrics: A Practical Approach.
International Conference on Data Science in Cyberspace (DSC)(2021)
摘要
The inherent vulnerability of deep learning systems, which is subject to adversarial attacks, limits its application in the safety-critical domain such as automatic driving, military application and so on. More seriously, the implementations of adversarial perturbation in the physical world are proved feasible by a considerable body of works. Hence, it is crucial to develop the capability of defense that matches adversarial attacks. In this paper, we firstly investigate various defensive methodologies and find that the detection-only approach shows its superiority in terms of robustness and efficiency when facing strong attack strategies. Next, detection approaches with different statistical metrics are presented, such as Kernel Density Estimation(KDE), Local Intrinsic Dimensionality (LID) and Neural Network Invariant Checking (NIC), to prove the hypothesis that adversarial examples can be totally detected if choose the reasonable statistical metrics. Hence, we believe that the research on finding more proper metrics is a promising and practical direction. Moreover, given the absence of a systematic evaluation framework, we proposed a threat model and evaluation criteria as the first step for evaluating various defense measures. Finally, it also remains a huge challenge and needs further researches to accomplish a trade-off between security and efficiency in this area.
更多查看译文
关键词
Adversarial attack,complete defense,detection,statistical metric
AI 理解论文
溯源树
样例
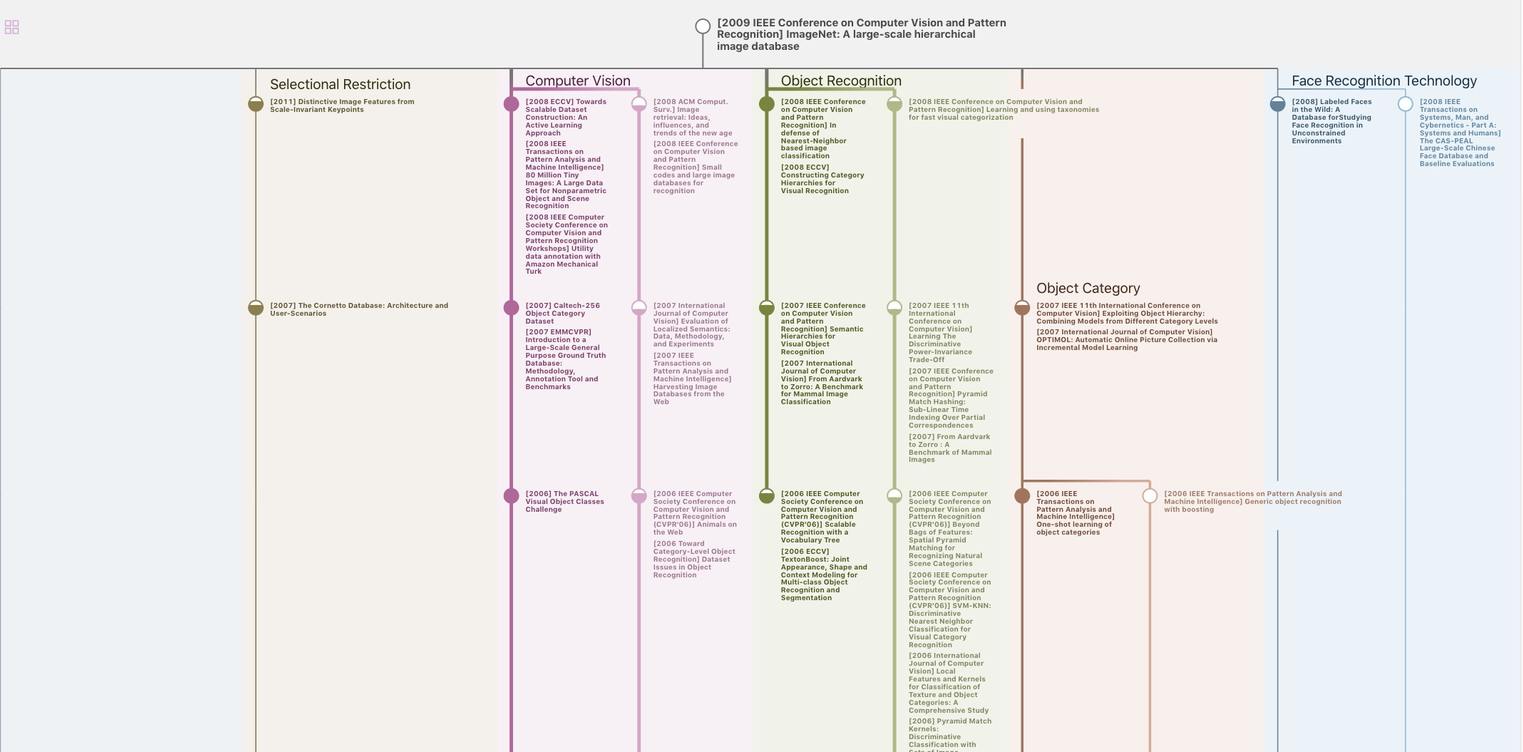
生成溯源树,研究论文发展脉络
Chat Paper
正在生成论文摘要