A Hybrid Deep Learning Approach for Advanced Persistent Threat Attack Detection.
International Conference on Future Networks and Distributed Systems (ICFNDS)(2021)
摘要
Advanced Persistent Threat (APT) attack is one of the most common and costly destructive attacks on the target system. This attack has become a challenge for companies, governments, and organizations’ information security systems. In recent years, methods for detecting and preventing APT attacks that use machine learning or deep learning algorithms to analyze indications and anomalous behaviors in network traffic have become popular. However, due to a lack of typical data from attack campaigns, the APT attack detection approach that uses behavior analysis and evaluation approaches encounter many issues. Network traffic analysis to detect a common APT attack is one of the solutions for dealing with this situation. This paper develops efficient and flexible deep learning models. To analyze huge network traffic, a hybrid deep learning approach that builds two models is used: Stacked Autoencoder with Long Short-Term Memory (SAE-LSTM) and Convolutional Neural Networks with Long Short-Term Memory Network (CNN-LSTM) to detect indications of APT attacks. A reliable dataset ’DAPT2020’ that covers all APT stages is used to evaluate the proposed approach. The experimental results demonstrate that the hybrid deep learning approach proved to give higher performance than the individual deep learning model in detecting malicious behavior in each APT stage.
更多查看译文
AI 理解论文
溯源树
样例
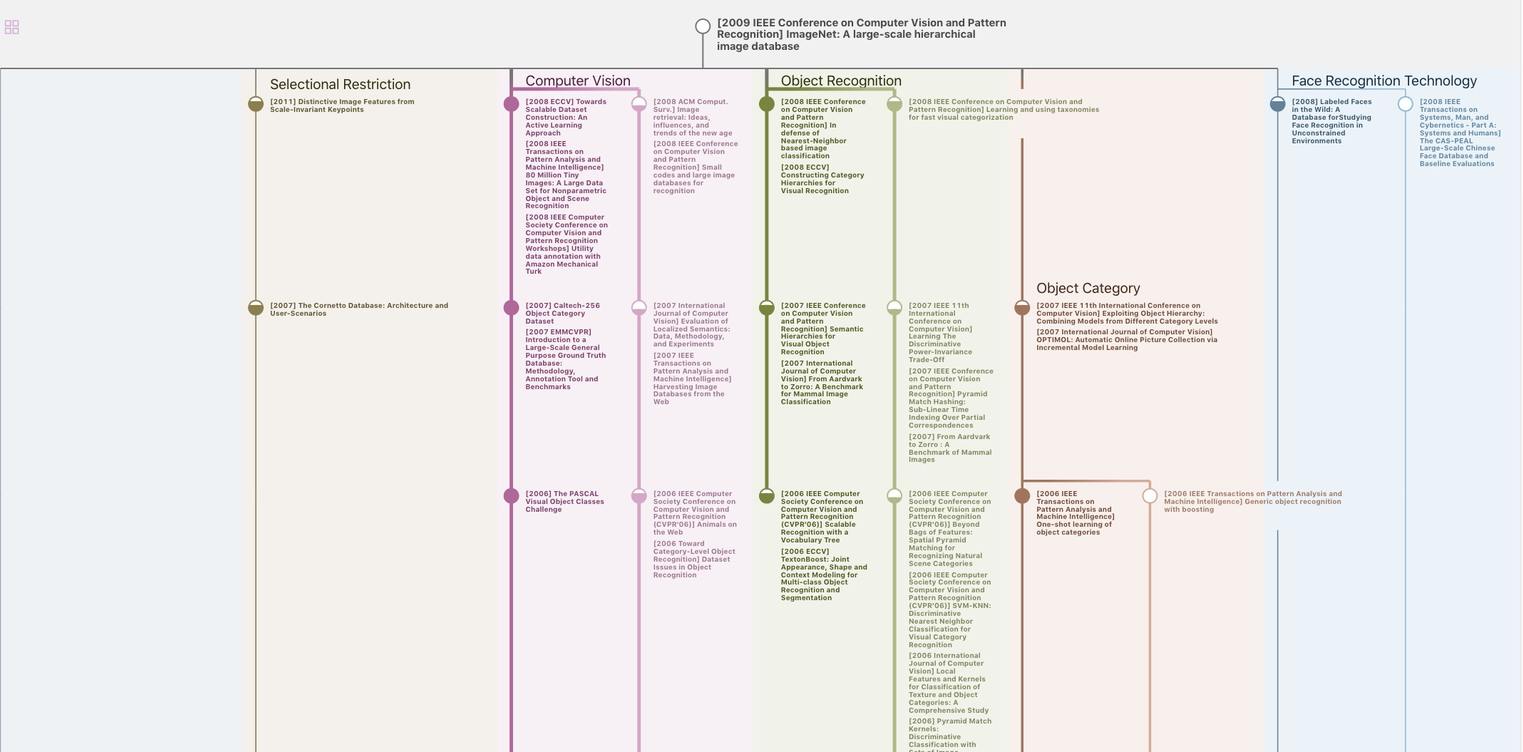
生成溯源树,研究论文发展脉络
Chat Paper
正在生成论文摘要