Forecasting of Beijing PM2.5 with a hybrid ARIMA model based on integrated AIC and improved GS fixed-order methods and seasonal decomposition
Heliyon(2022)
摘要
Accurate particulate matter 2.5 (PM) prediction plays a crucial role in the accurate management of air pollution and prevention of respiratory diseases. However, PM, as a nonlinear time series with great volatility, is difficult to achieve accurate prediction. In this paper, a hybrid autoregressive integrated moving average (ARIMA) model is proposed based on the Augmented Dickey-Fuller test (ADF root test) of annual PM data, thus demonstrating the necessity of first-order difference. The new method of using integrated akaike information criterion (AIC) and improved grid search (GS) methods is proposed to avoid the bias caused by using AIC alone to determine the order because the data are not exactly normally distributed. The comprehensive evaluation coefficient (CEC) is used to select the optimal parameter structure of the prediction model by considering multiple evaluation perspectives. The entropy value of the decomposed series is obtained by using range entropy A (RangeEn_A), and the series is reconstructed according to the entropy value, and finally the reconstructed series is predicted. We used Beijing PM data for validation and the results showed that the new hybrid ARIMA model improved values of RMSE 99.23%, MAE 99.20%, R 118.61%, TIC 99.28%, NMAE 98.71%, NMSE 99.97%, OPC 43.13%, MOPC 98.43% and CEC 99.25% compared with the traditional ARIMA model. The results show that the method does greatly improve the prediction performance and provides a convincing tool for policy formulation and governance.
更多查看译文
关键词
Autoregressive integrated moving average (ARIMA),Beijing PM2.5 prediction,Comprehensive evaluation coefficient (CEC),Integrated fixed-order,Seasonal decomposition and reconstruction
AI 理解论文
溯源树
样例
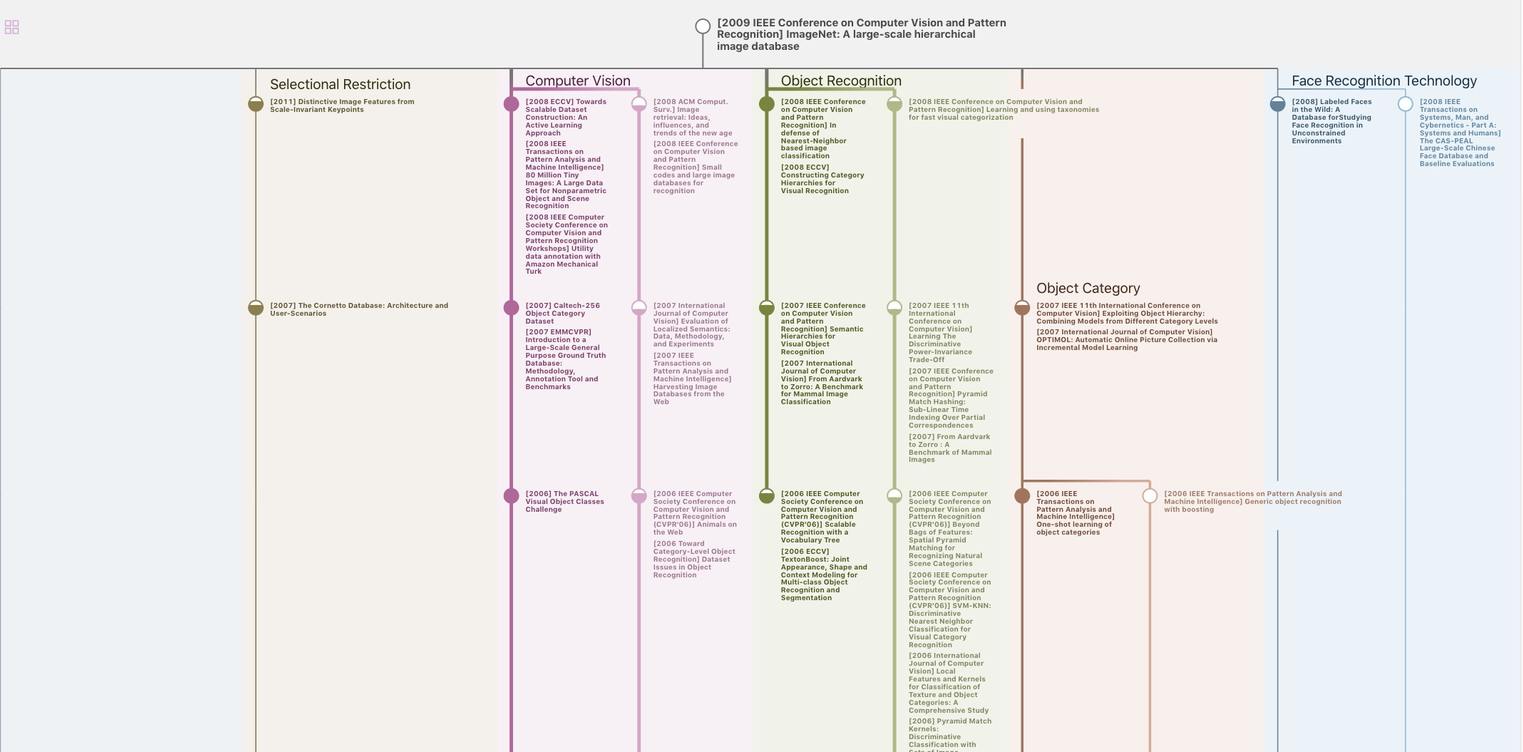
生成溯源树,研究论文发展脉络
Chat Paper
正在生成论文摘要