Automated Multi-Modal Transformer Network (amtnet) for 3D Medical Images Segmentation.
PHYSICS IN MEDICINE AND BIOLOGY(2023)
摘要
Objective.Over the past years, convolutional neural networks based methods have dominated the field of medical image segmentation. But the main drawback of these methods is that they have difficulty representing long-range dependencies. Recently, the Transformer has demonstrated super performance in computer vision and has also been successfully applied to medical image segmentation because of the self-attention mechanism and long-range dependencies encoding on images. To the best of our knowledge, only a few works focus on cross-modalities of image segmentation using the Transformer. Hence, the main objective of this study was to design, propose and validate a deep learning method to extend the application of Transformer to multi-modality medical image segmentation.Approach.This paper proposes a novel automated multi-modal Transformer network termed AMTNet for 3D medical image segmentation. Especially, the network is a well-modeled U-shaped network architecture where many effective and significant changes have been made in the feature encoding, fusion, and decoding parts. The encoding part comprises 3D embedding, 3D multi-modal Transformer, and 3D Co-learn down-sampling blocks. Symmetrically, the 3D Transformer block, upsampling block, and 3D-expanding blocks are included in the decoding part. In addition, a Transformer-based adaptive channel interleaved Transformer feature fusion module is designed to fully fuse features of different modalities.Main results.We provide a comprehensive experimental analysis of the Prostate and BraTS2021 datasets. The results show that our method achieves an average DSC of 0.907 and 0.851 (0.734 for ET, 0.895 for TC, and 0.924 for WT) on these two datasets, respectively. These values show that AMTNet yielded significant improvements over the state-of-the-art segmentation networks.Significance.The proposed 3D segmentation network exploits complementary features of different modalities during the feature extraction process at multiple scales to increase the 3D feature representations and improve the segmentation efficiency. This powerful network enriches the research of the Transformer to multi-modal medical image segmentation.
更多查看译文
关键词
Image Segmentation,Medical Image Analysis,MRI Segmentation,Deformable Image Registration,Image Recognition
AI 理解论文
溯源树
样例
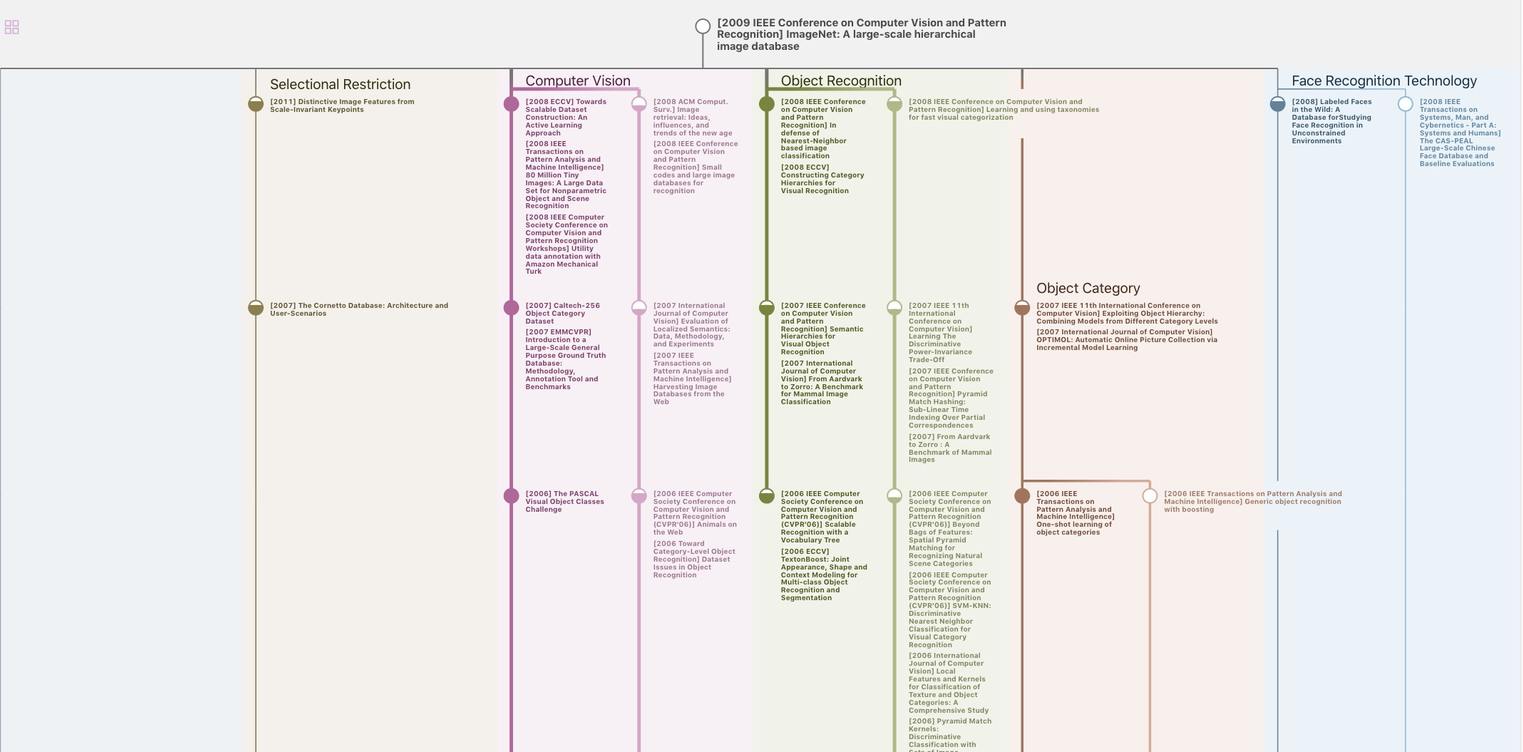
生成溯源树,研究论文发展脉络
Chat Paper
正在生成论文摘要