Risk-Averse MDPs under Reward Ambiguity
arxiv(2023)
摘要
We propose a distributionally robust return-risk model for Markov decision processes (MDPs) under risk and reward ambiguity. The proposed model optimizes the weighted average of mean and percentile performances, and it covers the distributionally robust MDPs and the distributionally robust chance-constrained MDPs (both under reward ambiguity) as special cases. By considering that the unknown reward distribution lies in a Wasserstein ambiguity set, we derive the tractable reformulation for our model. In particular, we show that that the return-risk model can also account for risk from uncertain transition kernel when one only seeks deterministic policies, and that a distributionally robust MDP under the percentile criterion can be reformulated as its nominal counterpart at an adjusted risk level. A scalable first-order algorithm is designed to solve large-scale problems, and we demonstrate the advantages of our proposed model and algorithm through numerical experiments.
更多查看译文
关键词
risk-averse
AI 理解论文
溯源树
样例
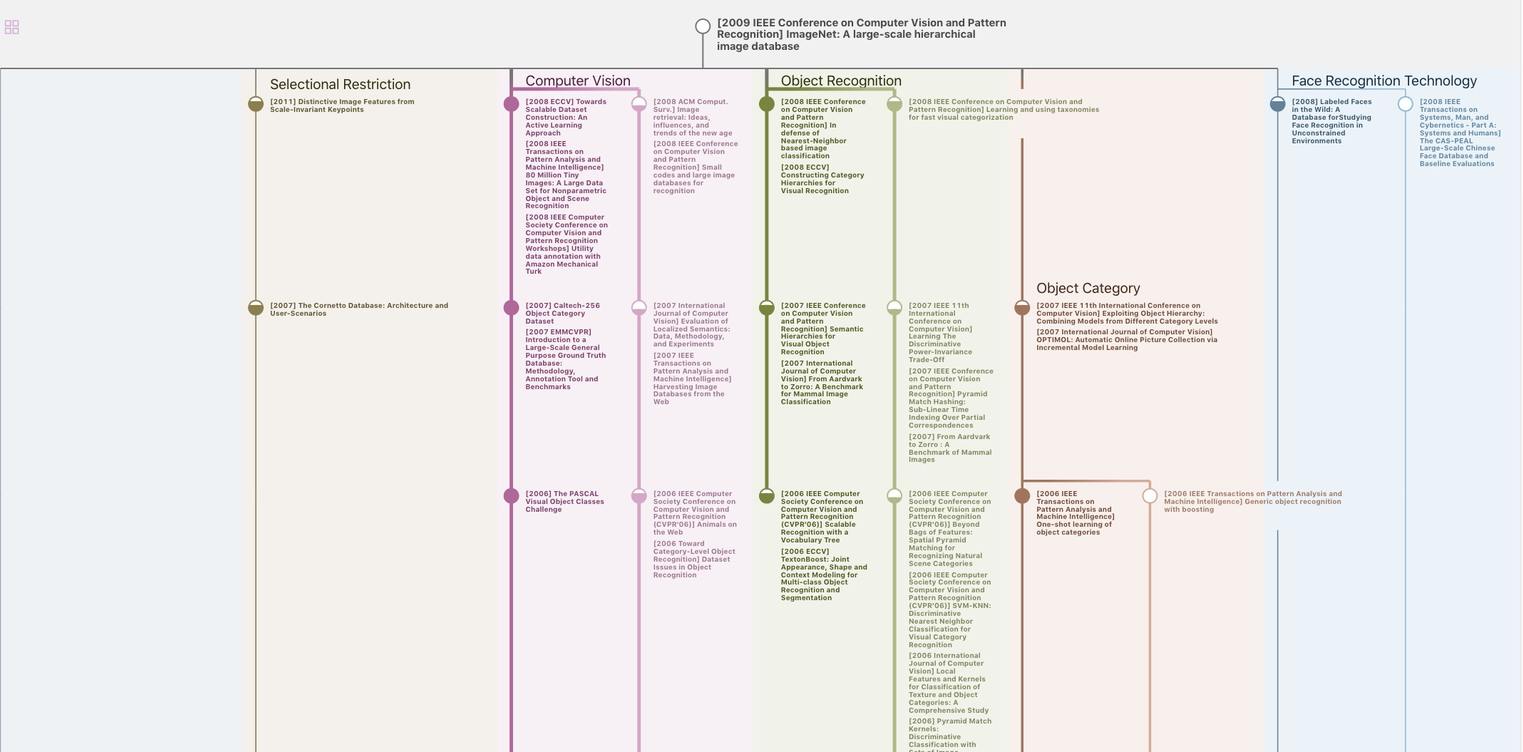
生成溯源树,研究论文发展脉络
Chat Paper
正在生成论文摘要