Learning-Based Model Predictive Control for the Energy Management of Hybrid Electric Vehicles Including Driving Mode Decisions
IEEE Transactions on Vehicular Technology(2023)
摘要
This paper presents an online-capable controller for the energy management system of a parallel hybrid electric vehicle based on model predictive control. Its task is to minimize the vehicle's fuel consumption along a predicted driving mission by calculating the distribution of the driver's power request between the electrical and the combustive part of the powertrain, and by choosing the driving mode, which depends on the vehicle's clutch state. The inclusion of the clutch state in a model predictive control structure is not trivial because the underlying optimization problem becomes a mixed-integer program as a consequence. Using Pontryagin's Minimum Principle and a simplified vehicle model, it is possible to prove that a drive cycle-dependent critical power request Pcrit exists, which uniquely separates the optimal driving mode. Based on this result, a learning algorithm is proposed to determine Pcrit during the operation of the vehicle. The learning algorithm is incorporated into a multi-level controller structure and the working principle of the resulting multi-level learning-based model predictive controller is analyzed in detail using two realistic driving missions. A comparison to the solution obtained by Dynamic Programming reveals that the proposed controller achieves close-to-optimal performance.
更多查看译文
AI 理解论文
溯源树
样例
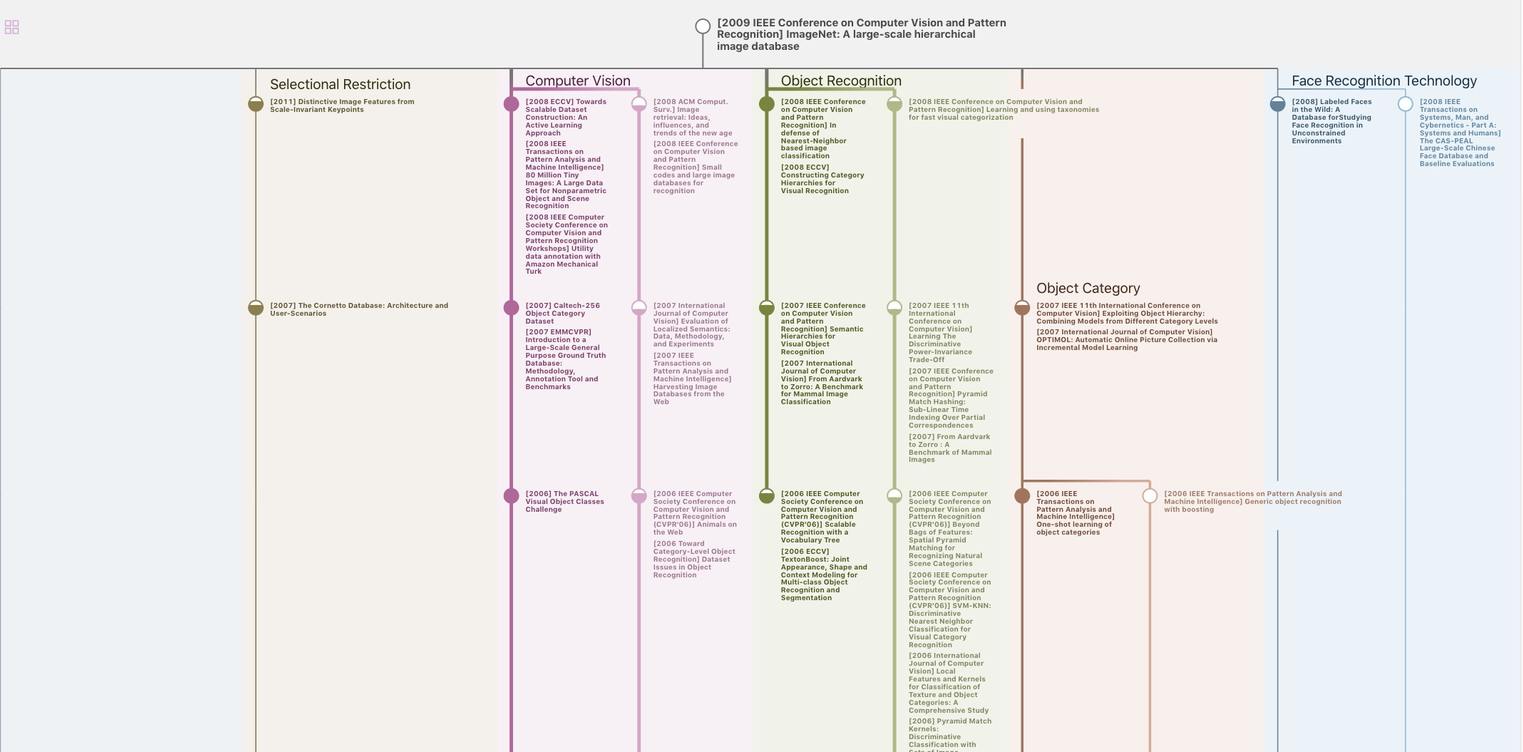
生成溯源树,研究论文发展脉络
Chat Paper
正在生成论文摘要