Emergent collective intelligence from massive-agent cooperation and competition
CoRR(2023)
Abstract
Inspired by organisms evolving through cooperation and competition between different populations on Earth, we study the emergence of artificial collective intelligence through massive-agent reinforcement learning. To this end, We propose a new massive-agent reinforcement learning environment, Lux, where dynamic and massive agents in two teams scramble for limited resources and fight off the darkness. In Lux, we build our agents through the standard reinforcement learning algorithm in curriculum learning phases and leverage centralized control via a pixel-to-pixel policy network. As agents co-evolve through self-play, we observe several stages of intelligence, from the acquisition of atomic skills to the development of group strategies. Since these learned group strategies arise from individual decisions without an explicit coordination mechanism, we claim that artificial collective intelligence emerges from massive-agent cooperation and competition. We further analyze the emergence of various learned strategies through metrics and ablation studies, aiming to provide insights for reinforcement learning implementations in massive-agent environments.
MoreTranslated text
Key words
Reinforcement Learning,Multi-agent System,Emergent Behavior
AI Read Science
Must-Reading Tree
Example
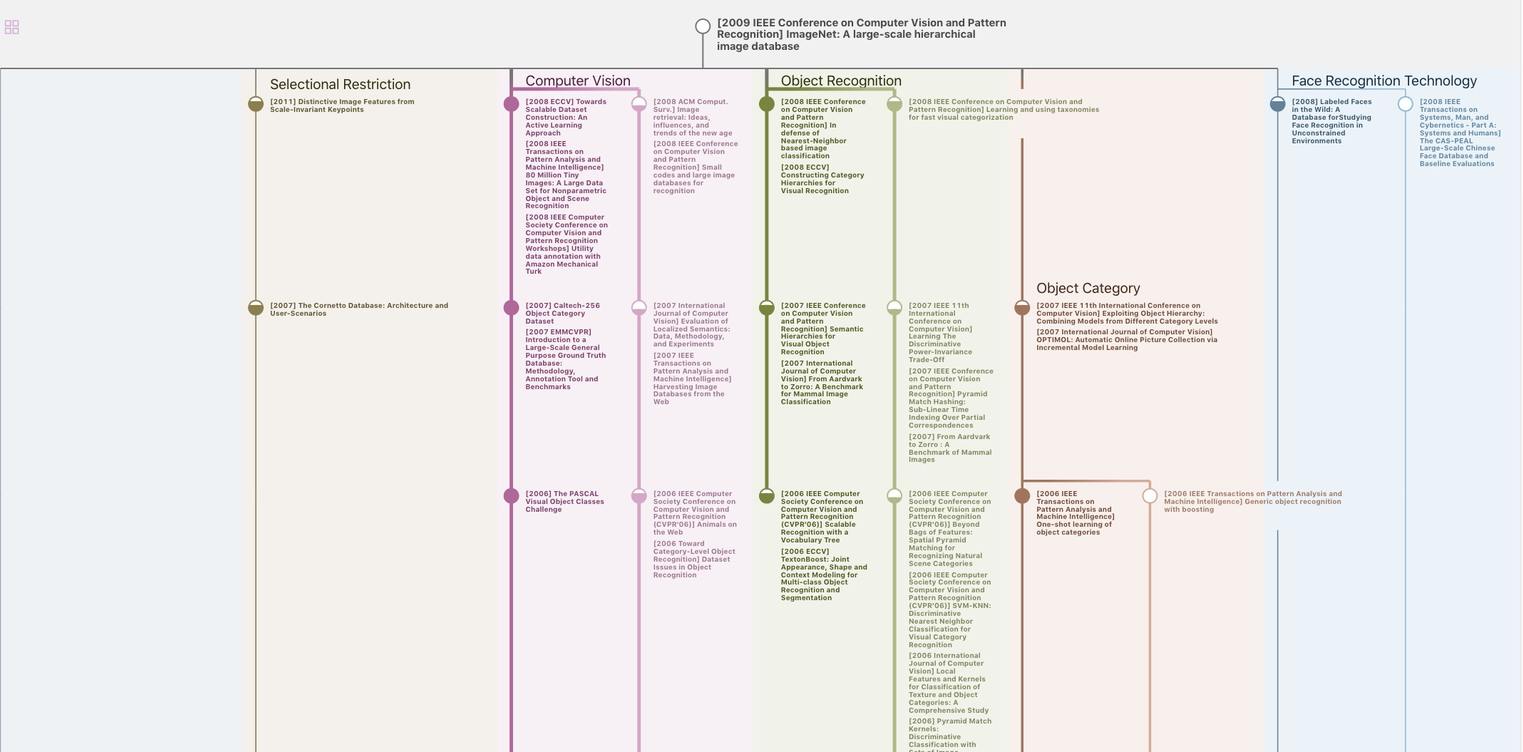
Generate MRT to find the research sequence of this paper
Chat Paper
Summary is being generated by the instructions you defined